Hyperparameter Selection for Imitation Learning
ICML(2021)
摘要
We address the issue of tuning hyperparameters (HPs) for imitation learning algorithms in the context of continuous-control, when the underlying reward function of the demonstrating expert cannot be observed at any time. The vast literature in imitation learning mostly considers this reward function to be available for HP selection, but this is not a realistic setting. Indeed, would this reward function be available, it could then directly be used for policy training and imitation would not be necessary. To tackle this mostly ignored problem, we propose a number of possible proxies to the external reward. We evaluate them in an extensive empirical study (more than 10'000 agents across 9 environments) and make practical recommendations for selecting HPs. Our results show that while imitation learning algorithms are sensitive to HP choices, it is often possible to select good enough HPs through a proxy to the reward function.
更多查看译文
关键词
imitation,selection,learning
AI 理解论文
溯源树
样例
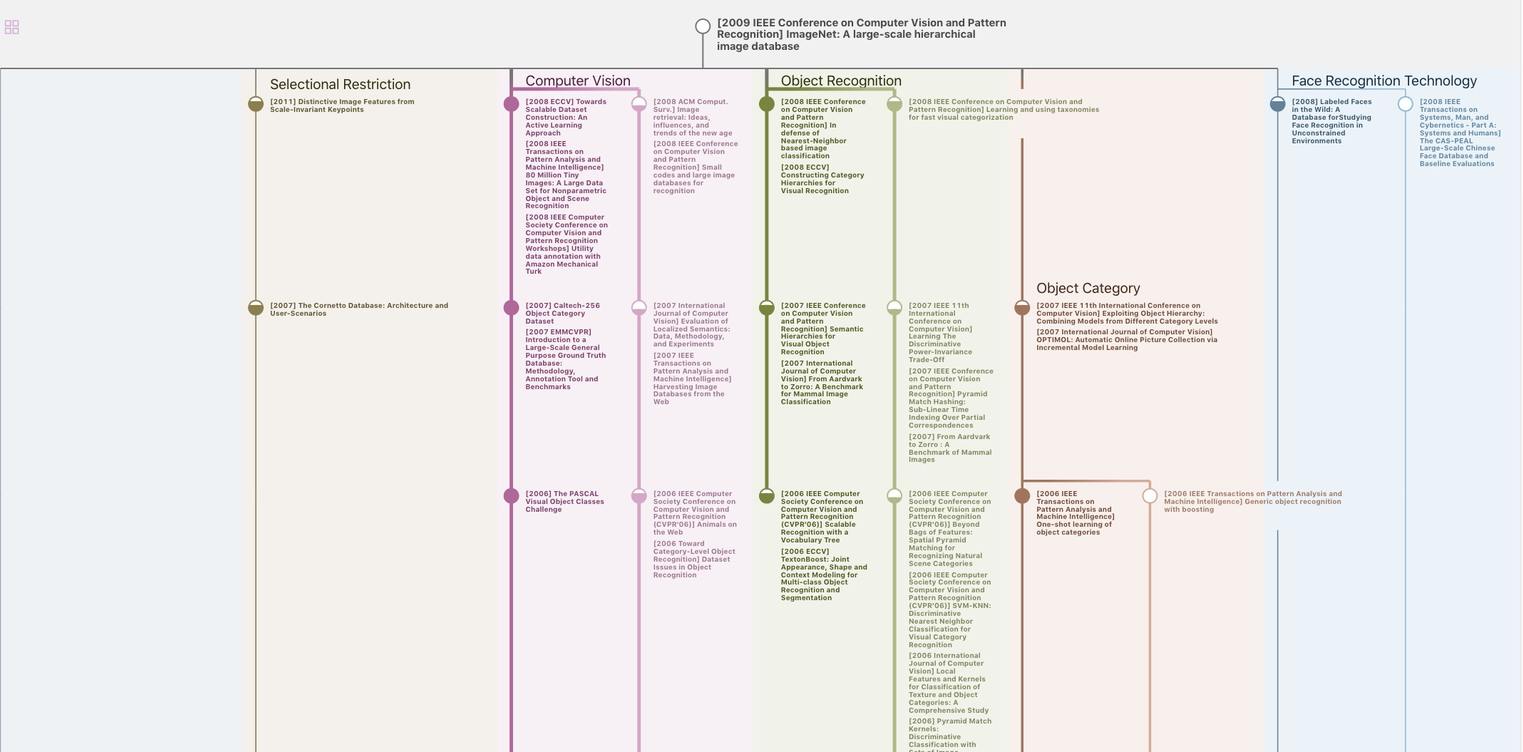
生成溯源树,研究论文发展脉络
Chat Paper
正在生成论文摘要