Criterion-based Heterogeneous Collaborative Filtering for Multi-behavior Implicit Recommendation
ACM TRANSACTIONS ON KNOWLEDGE DISCOVERY FROM DATA(2024)
摘要
Recent years have witnessed the explosive growth of interaction behaviors in multimedia information systems, where multi-behavior recommender systems have received increasing attention by leveraging data from various auxiliary behaviors such as tip and collect. Among various multi-behavior recommendation methods, non-sampling methods have shown superiority over negative sampling methods. However, two observations are usually ignored in existing state-of-the-art non-sampling methods based on binary regression: (1) users have different preference strengths for different items, so they cannot be measured simply by binary implicit data; (2) the dependency across multiple behaviors varies for different users and items. To tackle the above issue, we propose a novel non-sampling learning framework named Criterion-guided Heterogeneous Collaborative Filtering (CHCF). CHCF introduces both upper and lower thresholds to indicate selection criteria, whichwill guide user preference learning. Besides, CHCF integrates criterion learning and user preference learning into a unified framework, which can be trained jointly for the interaction prediction of the target behavior. We further theoretically demonstrate that the optimization of Collaborative Metric Learning can be approximately achieved by the CHCF learning framework in a non-sampling form effectively. Extensive experiments on three real-world datasets show the effectiveness of CHCF in heterogeneous scenarios.
更多查看译文
关键词
Collaborative filtering,neural networks,implicit feedback,multi-behavior recommendation
AI 理解论文
溯源树
样例
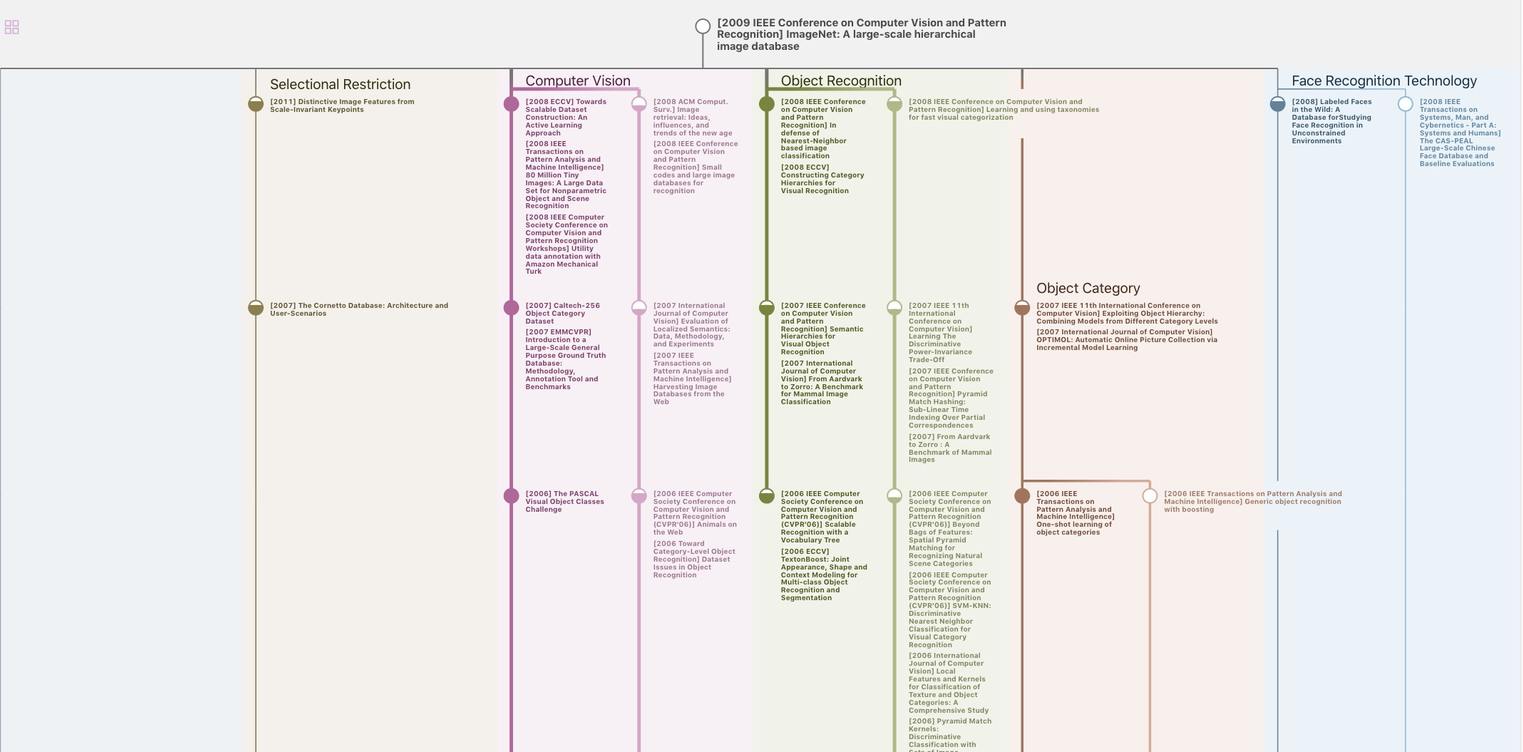
生成溯源树,研究论文发展脉络
Chat Paper
正在生成论文摘要