The Perturbed Prox-Preconditioned Spider Algorithm: Non-Asymptotic Convergence Bounds
2021 IEEE Statistical Signal Processing Workshop (SSP)(2021)
摘要
A novel algorithm named PerturbedProx-Preconditioned SPIDER (3P-SPIDER) is introduced. It is a stochastic variancereduced proximal-gradient type algorithm built on Stochastic Path Integral Differential EstimatoR (SPIDER), an algorithm known to achieve near-optimal first-order oracle inequality for nonconvex and nonsmooth optimization. Compared to the vanilla prox-SPIDER, 3P-SPIDER uses preconditioned gradient estimators. Preconditioning can either be applied "explicitly" to a gradient estimator or be introduced "implicitly" as in applications to the EM algorithm. 3P-SPIDER also assumes that the preconditioned gradients may (possibly) be not known in closed analytical form and therefore must be approximated which adds an additional degree of perturbation. Studying the convergence in expectation, we show that 3P-SPIDER achieves a near-optimal oracle inequality O(n
1/2
/ϵ) where n is the number of observations and ϵ the target precision even when the gradient is estimated by Monte Carlo methods. We illustrate the algorithm on an application to the minimization of a penalized empirical loss.
更多查看译文
关键词
Statistical Learning,Large Scale Learning,Variance reduced Stochastic gradient,Finite sum optimization,Control Variates
AI 理解论文
溯源树
样例
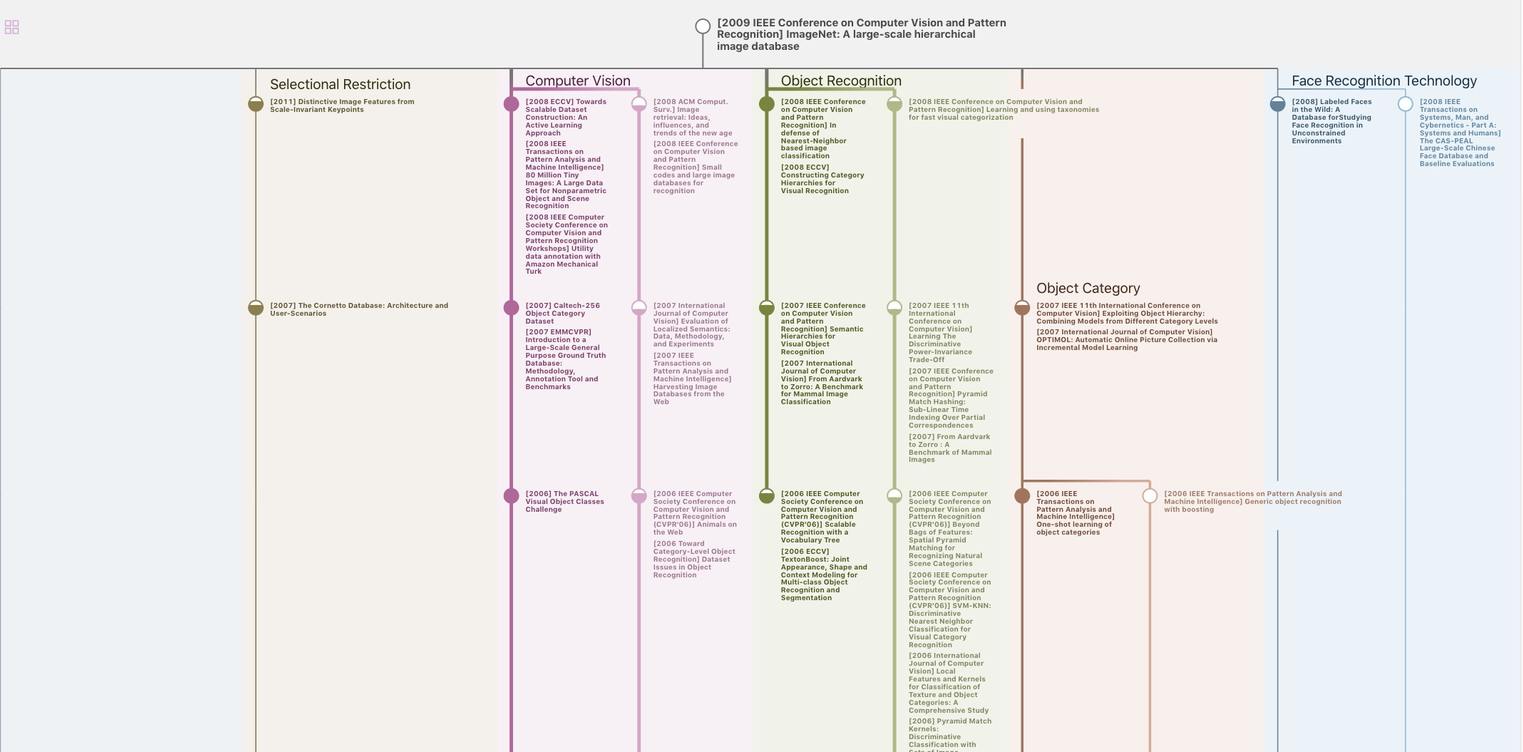
生成溯源树,研究论文发展脉络
Chat Paper
正在生成论文摘要