THP: Topological Hawkes Processes for Learning Granger Causality on Event Sequences
arxiv(2021)
摘要
Learning Granger causality among event types on multi-type event sequences is an important but challenging task. Existing methods, such as the Multivariate Hawkes processes, mostly assumed that each sequence is independent and identically distributed. However, in many real-world applications, it is commonplace to encounter a topological network behind the event sequences such that an event is excited or inhibited not only by its history but also by its topological neighbors. Consequently, the failure in describing the topological dependency among the event sequences leads to the error detection of the causal structure. By considering the Hawkes processes from the view of temporal convolution, we propose a Topological Hawkes processes (THP) to draw a connection between the graph convolution in topology domain and the temporal convolution in time domains. We further propose a Granger causality learning method on THP in a likelihood framework. The proposed method is featured with the graph convolution-based likelihood function of THP and a sparse optimization scheme with an Expectation-Maximization of the likelihood function. Theoretical analysis and experiments on both synthetic and real-world data demonstrate the effectiveness of the proposed method.
更多查看译文
关键词
topological hawkes processes,learning granger causality,event sequences
AI 理解论文
溯源树
样例
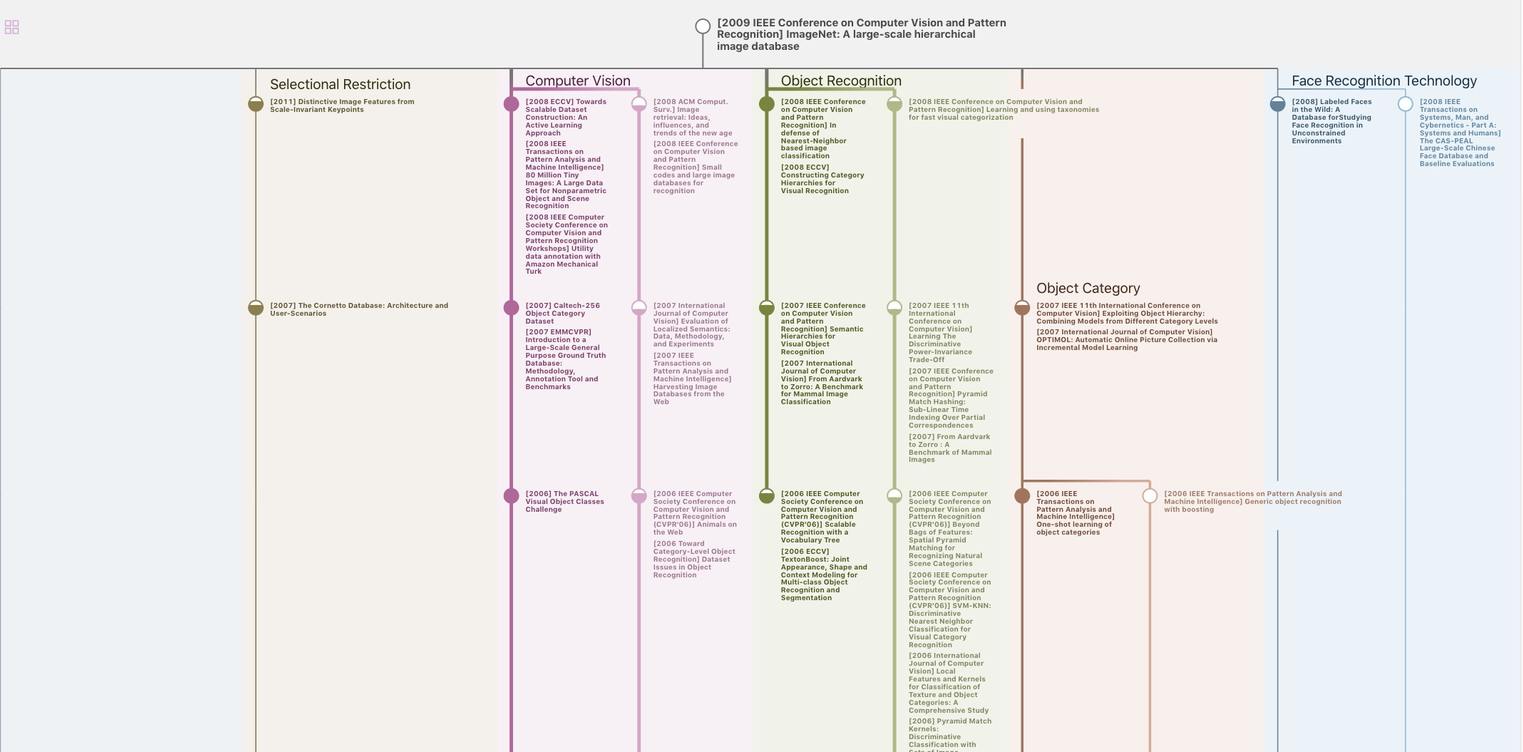
生成溯源树,研究论文发展脉络
Chat Paper
正在生成论文摘要