Novel Autosegmentation Spatial Similarity Metrics Capture the Time Required to Correct Segmentations Better Than Traditional Metrics in a Thoracic Cavity Segmentation Workflow
JOURNAL OF DIGITAL IMAGING(2021)
摘要
Automated segmentation templates can save clinicians time compared to de novo segmentation but may still take substantial time to review and correct. It has not been thoroughly investigated which automated segmentation-corrected segmentation similarity metrics best predict clinician correction time. Bilateral thoracic cavity volumes in 329 CT scans were segmented by a UNet-inspired deep learning segmentation tool and subsequently corrected by a fourth-year medical student. Eight spatial similarity metrics were calculated between the automated and corrected segmentations and associated with correction times using Spearman’s rank correlation coefficients. Nine clinical variables were also associated with metrics and correction times using Spearman’s rank correlation coefficients or Mann–Whitney U tests. The added path length, false negative path length, and surface Dice similarity coefficient correlated better with correction time than traditional metrics, including the popular volumetric Dice similarity coefficient (respectively ρ = 0.69, ρ = 0.65, ρ = − 0.48 versus ρ = − 0.25; correlation p values < 0.001). Clinical variables poorly represented in the autosegmentation tool’s training data were often associated with decreased accuracy but not necessarily with prolonged correction time. Metrics used to develop and evaluate autosegmentation tools should correlate with clinical time saved. To our knowledge, this is only the second investigation of which metrics correlate with time saved. Validation of our findings is indicated in other anatomic sites and clinical workflows. Novel spatial similarity metrics may be preferable to traditional metrics for developing and evaluating autosegmentation tools that are intended to save clinicians time.
更多查看译文
关键词
Image segmentation, "Computer-assisted image analysis" [MeSH], "AI artificial intelligence" [MeSH], "Medical imaging" [MeSH], "Clinical informatics" [MeSH]
AI 理解论文
溯源树
样例
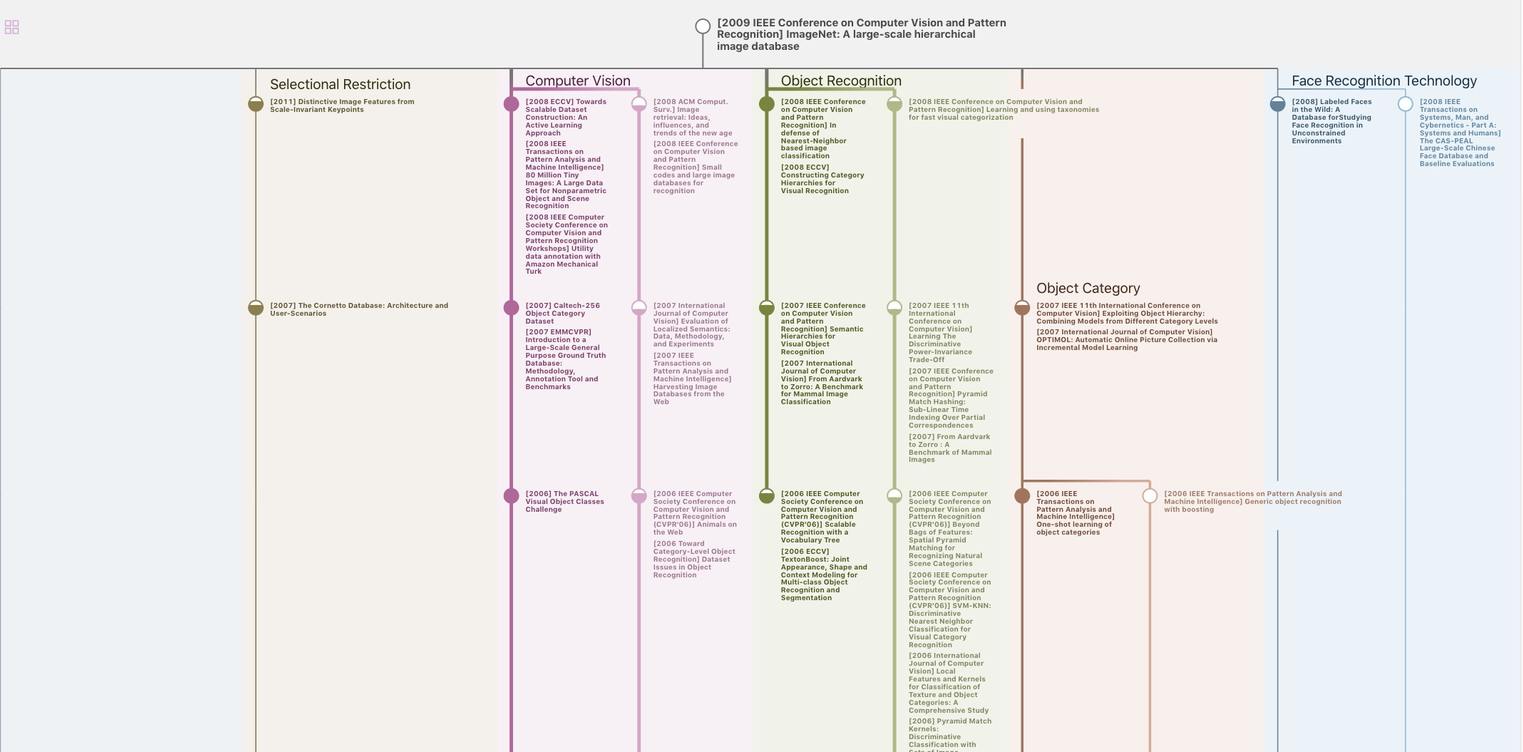
生成溯源树,研究论文发展脉络
Chat Paper
正在生成论文摘要