Exploring Robustness of Unsupervised Domain Adaptation in Semantic Segmentation.
ICCV(2021)
摘要
Recent studies imply that deep neural networks are vulnerable to adversarial examples -- inputs with a slight but intentional perturbation are incorrectly classified by the network. Such vulnerability makes it risky for some security-related applications (e.g., semantic segmentation in autonomous cars) and triggers tremendous concerns on the model reliability. For the first time, we comprehensively evaluate the robustness of existing UDA methods and propose a robust UDA approach. It is rooted in two observations: (i) the robustness of UDA methods in semantic segmentation remains unexplored, which pose a security concern in this field; and (ii) although commonly used self-supervision (e.g., rotation and jigsaw) benefits image tasks such as classification and recognition, they fail to provide the critical supervision signals that could learn discriminative representation for segmentation tasks. These observations motivate us to propose adversarial self-supervision UDA (or ASSUDA) that maximizes the agreement between clean images and their adversarial examples by a contrastive loss in the output space. Extensive empirical studies on commonly used benchmarks demonstrate that ASSUDA is resistant to adversarial attacks.
更多查看译文
关键词
Transfer/Low-shot/Semi/Unsupervised Learning,Segmentation,grouping and shape
AI 理解论文
溯源树
样例
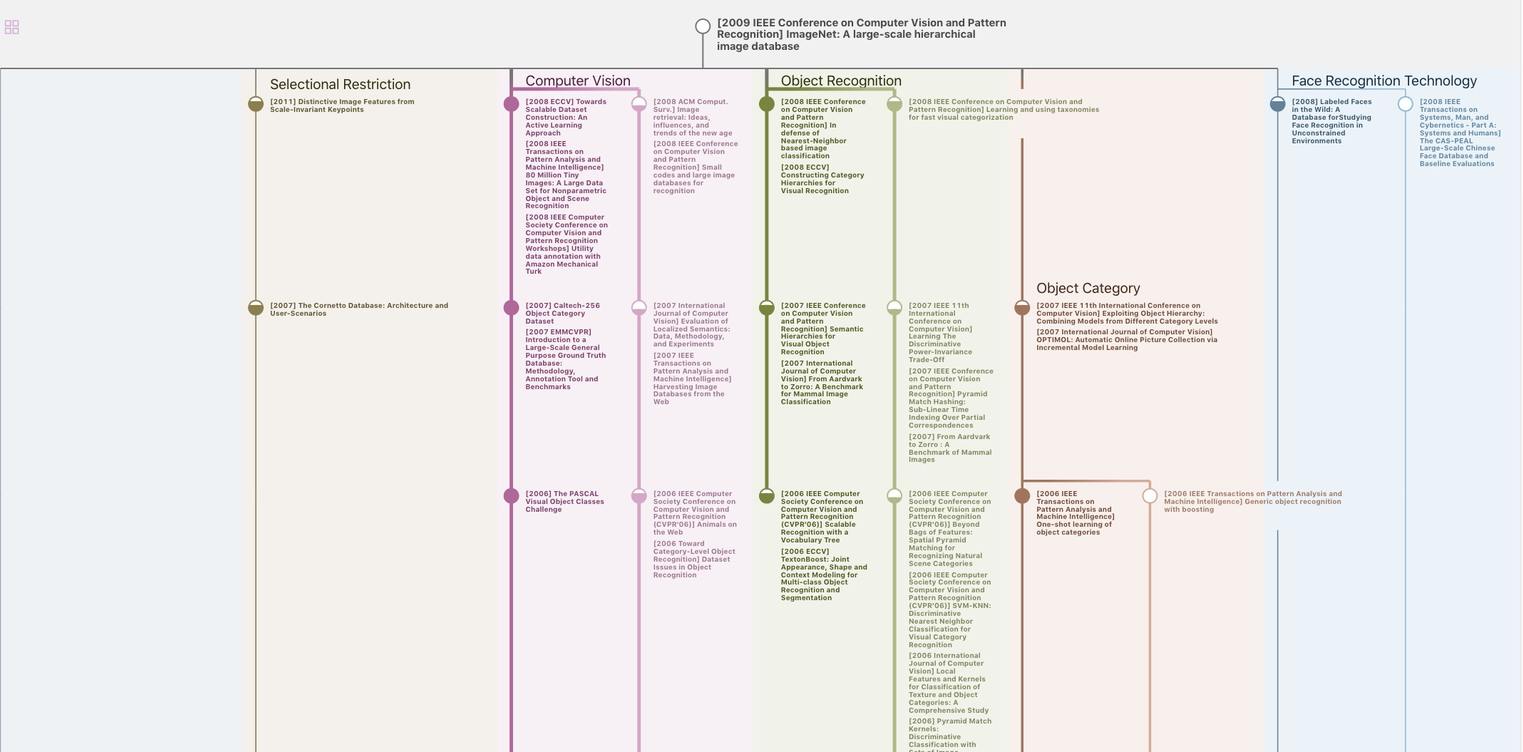
生成溯源树,研究论文发展脉络
Chat Paper
正在生成论文摘要