Deep-Learned Event Variables for Collider Phenomenology
arxiv(2021)
摘要
The choice of optimal event variables is crucial for achieving the maximal sensitivity of experimental analyses. Over time, physicists have derived suitable kinematic variables for many typical event topologies in collider physics. Here we introduce a deep learning technique to design good event variables, which are sensitive over a wide range of values for the unknown model parameters. We demonstrate that the neural networks trained with our technique on some simple event topologies are able to reproduce standard event variables like invariant mass, transverse mass, and stransverse mass. The method is automatable, completely general, and can be used to derive sensitive, previously unknown, event variables for other, more complex event topologies.
更多查看译文
关键词
collider phenomenology,event,deep-learned
AI 理解论文
溯源树
样例
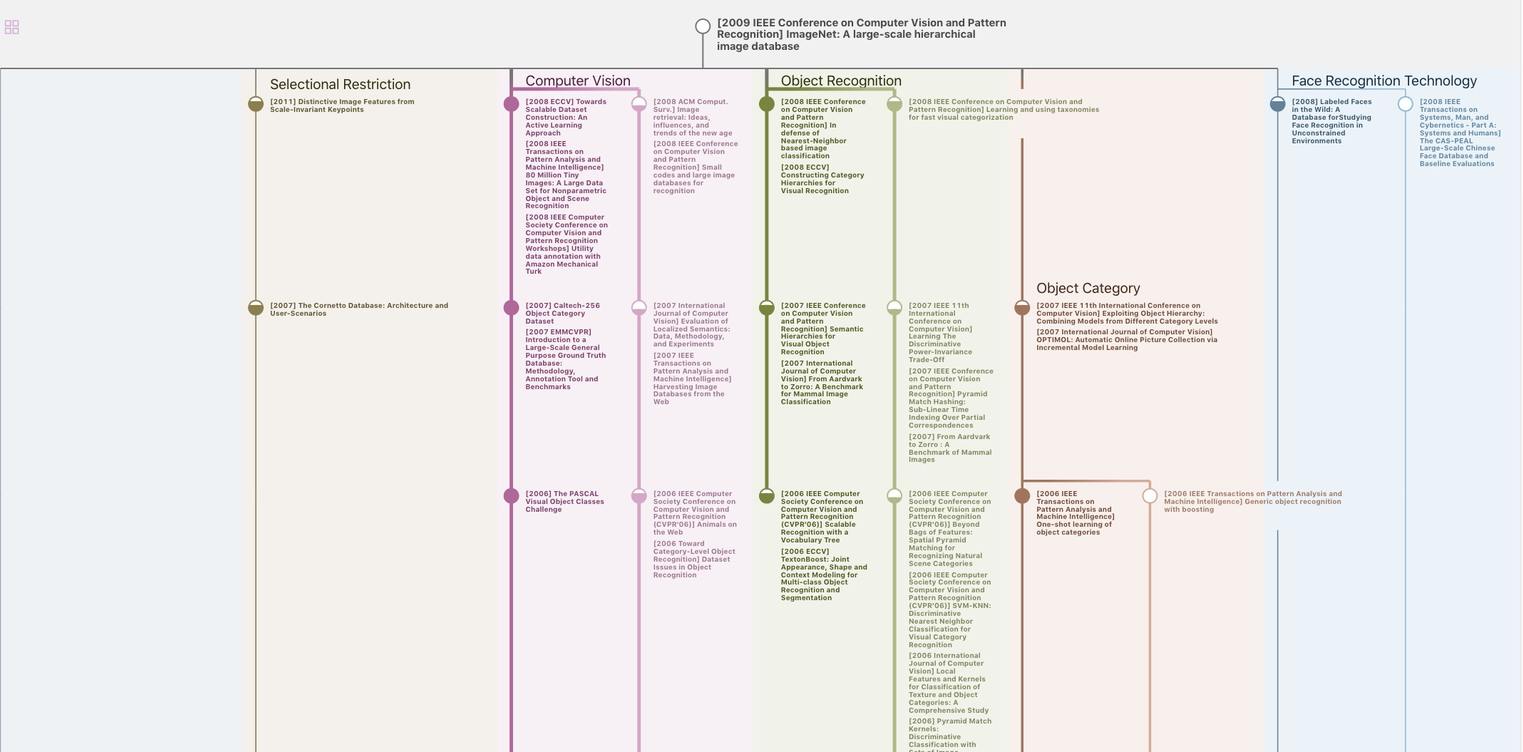
生成溯源树,研究论文发展脉络
Chat Paper
正在生成论文摘要