Unsupervised Monocular Visual Odometry With Decoupled Camera Pose Estimation
DIGITAL SIGNAL PROCESSING(2021)
摘要
Drift or error accumulation is an inevitable challenge in visual odometry (VO). To alleviate this issue, most of learning-based VO methods focus on various long and short term sequential learning schemes, while lose sight of the fact that inaccurate rotation estimate is the main source of VO drift. They usually estimate the six degrees of freedom (DoFs) of the camera motion simultaneously, without considering the inherent rotation-translation ambiguity. In this paper, we start from the designs of a cascade decoupled structure and a residual-based decoupled pose refinement scheme for accurate pose estimation. Then we extend them to an unsupervised monocular VO framework, which estimates the 3D camera poses by decoupling the estimations of rotation, translation and scale. Our VO model consists of three components: monocular depth estimation, a decoupled pose estimation and a decoupled pose refinement. The first component learns the metric scale and depth cues by using stereo pairs for training, and predicts the absolute depth of monocular inputs. The latter two separate the estimation and refinement of rotation and translation. To improve the robustness of the rotation estimation, we use the unit quaternion, instead of the Euler angles, to represent 3D rotation. We have evaluated our model on the KITTI Visual Odometry Evaluation benchmark. Comparison experiments demonstrate that our method is superior to the stateof-the-art unsupervised VO methods, and can achieve comparable results with the supervised ones. (C) 2021 Elsevier Inc. All rights reserved.
更多查看译文
关键词
Visual odometry, Unsupervised learning, Drift, Decoupled pose estimation, Unit quaternion
AI 理解论文
溯源树
样例
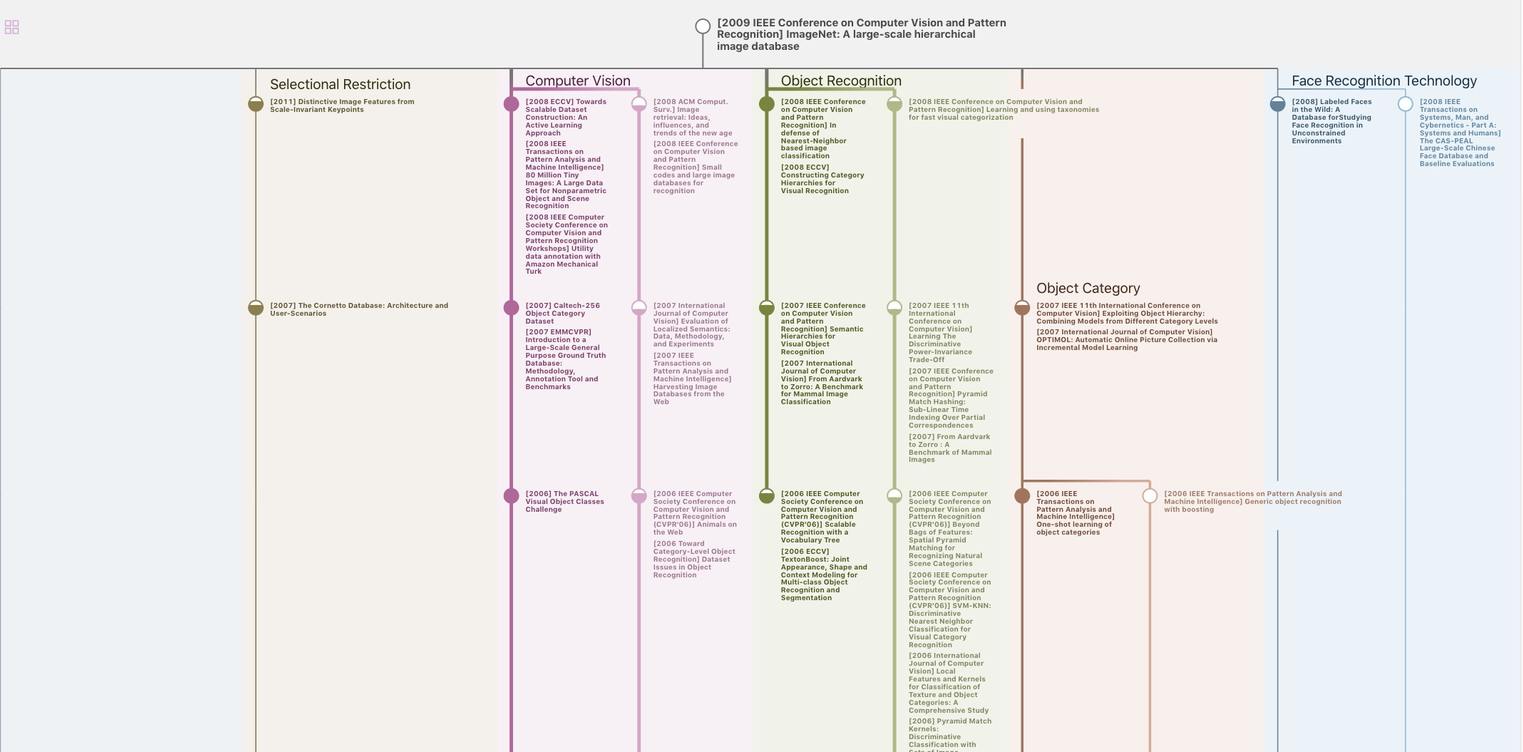
生成溯源树,研究论文发展脉络
Chat Paper
正在生成论文摘要