Distributed adaptive nearest neighbor classifier: algorithm and theory
arxiv(2023)
摘要
When data is of an extraordinarily large size or physically stored in different locations, the distributed nearest neighbor (NN) classifier is an attractive tool for classification. We propose a novel distributed adaptive NN classifier for which the number of nearest neighbors is a tuning parameter stochastically chosen by a data-driven criterion. An early stopping rule is proposed when searching for the optimal tuning parameter, which not only speeds up the computation but also improves the finite sample performance of the proposed algorithm. Convergence rate of excess risk of the distributed adaptive NN classifier is investigated under various sub-sample size compositions. In particular, we show that when the sub-sample sizes are sufficiently large, the proposed classifier achieves the nearly optimal convergence rate. Effectiveness of the proposed approach is demonstrated through simulation studies as well as an empirical application to a real-world dataset.
更多查看译文
关键词
adaptive nearest neighbor classifier,nearest neighbor,algorithm
AI 理解论文
溯源树
样例
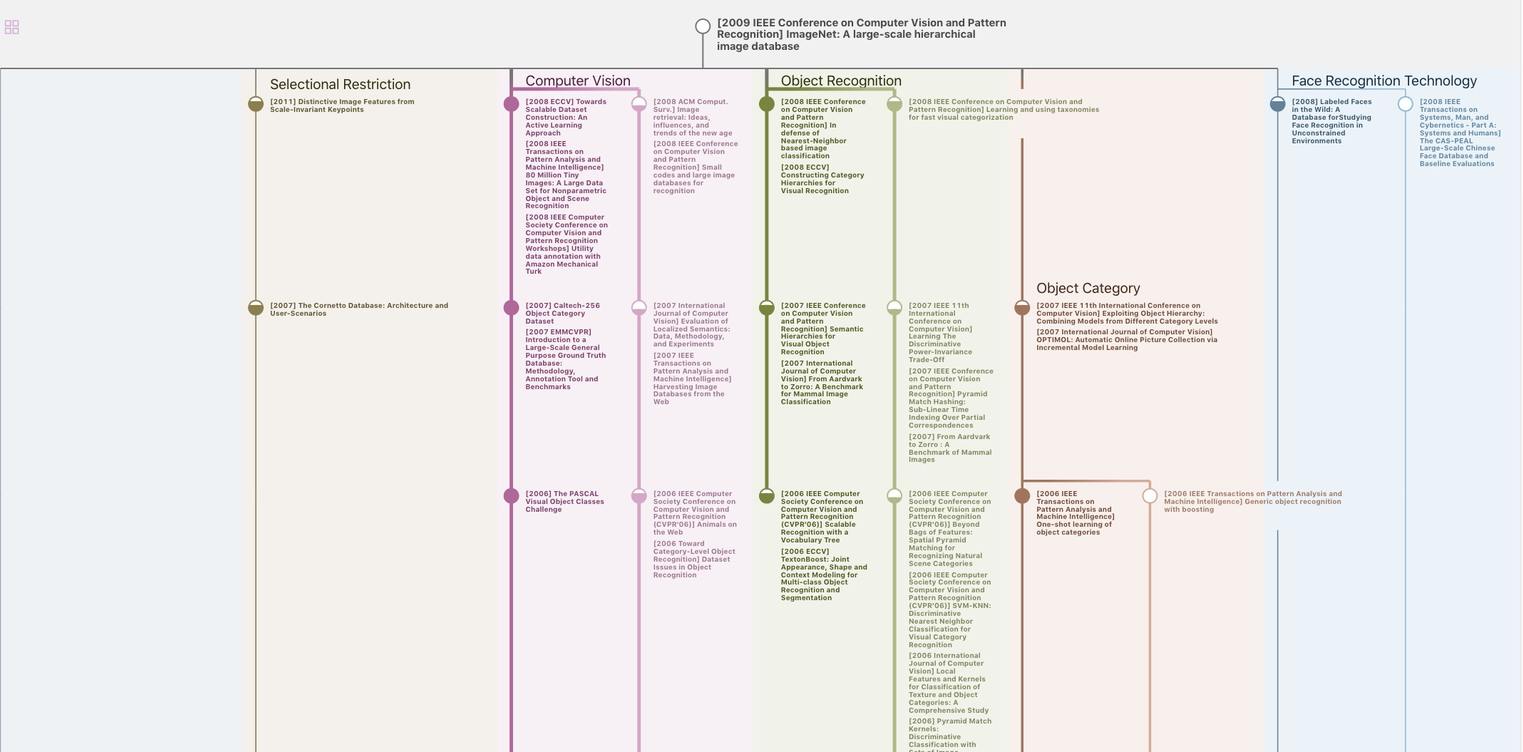
生成溯源树,研究论文发展脉络
Chat Paper
正在生成论文摘要