Manual Evaluation Matters - Reviewing Test Protocols of Distantly Supervised Relation Extraction.
ACL/IJCNLP(2021)
摘要
Distantly supervised (DS) relation extraction (RE) has attracted much attention in the past few years as it can utilize large-scale auto-labeled data. However, its evaluation has long been a problem: previous works either took costly and inconsistent methods to manually examine a small sample of model predictions, or directly test models on auto-labeled data -- which, by our check, produce as much as 53% wrong labels at the entity pair level in the popular NYT10 dataset. This problem has not only led to inaccurate evaluation, but also made it hard to understand where we are and what's left to improve in the research of DS-RE. To evaluate DS-RE models in a more credible way, we build manually-annotated test sets for two DS-RE datasets, NYT10 and Wiki20, and thoroughly evaluate several competitive models, especially the latest pre-trained ones. The experimental results show that the manual evaluation can indicate very different conclusions from automatic ones, especially some unexpected observations, e.g., pre-trained models can achieve dominating performance while being more susceptible to false-positives compared to previous methods. We hope that both our manual test sets and novel observations can help advance future DS-RE research.
更多查看译文
关键词
relation,distantly
AI 理解论文
溯源树
样例
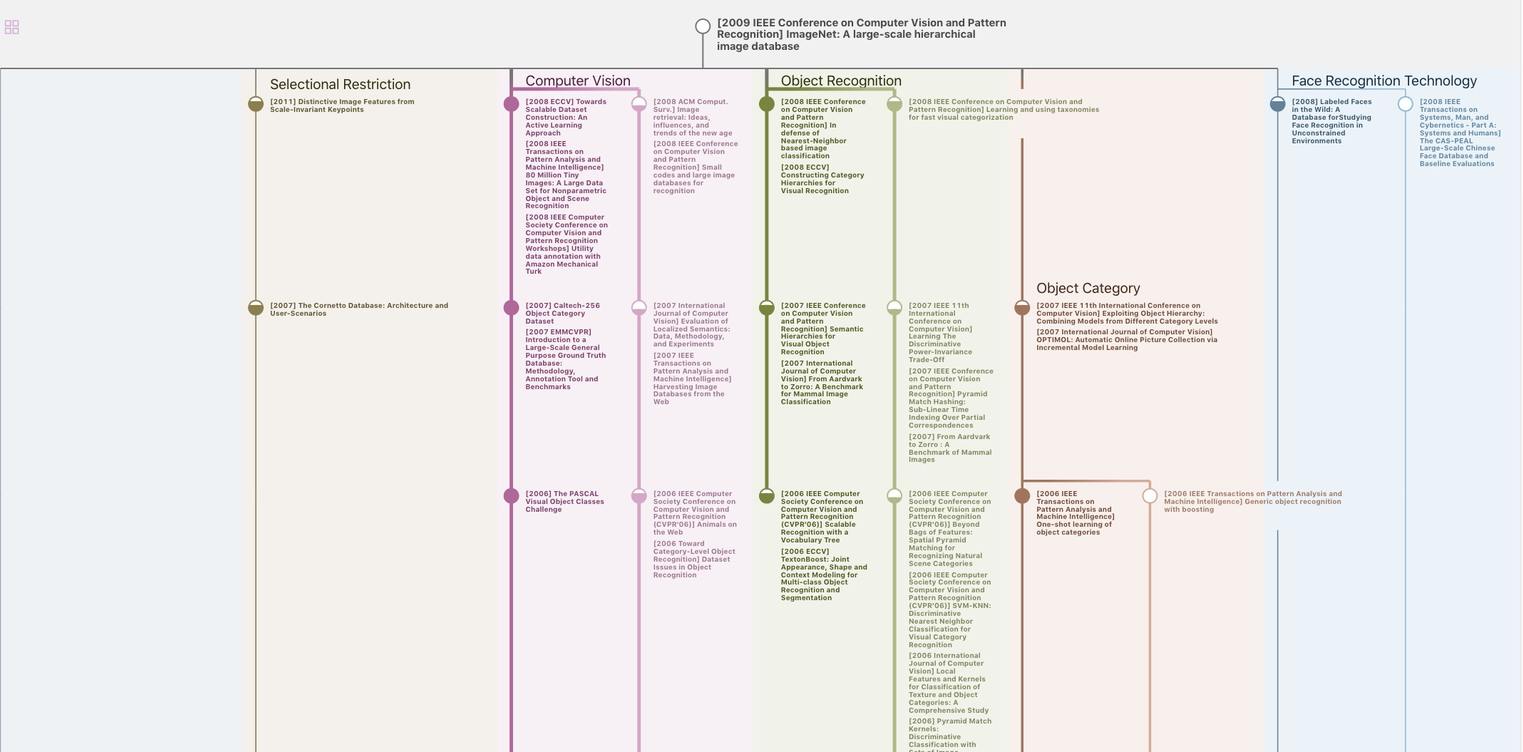
生成溯源树,研究论文发展脉络
Chat Paper
正在生成论文摘要