Unsupervised Learning of Adaptive Codebooks for Deep Feedback Encoding in FDD Systems
arxiv(2021)
摘要
In this work, we propose a joint adaptive codebook construction and feedback generation scheme in frequency divi-sion duplex (FDD) systems. Both unsupervised and supervised deep learning techniques are used for this purpose. Based on a recently discovered equivalence of uplink (UL) and downlink (DL) channel state information (CSI) in terms of neural network learning, the codebook and associated deep encoder for feedback signaling is based on UL data only. Subsequently, the feedback encoder can be offloaded to the mobile terminals (MTs) to generate channel feedback there as efficiently as possible, without any training effort at the terminals or corresponding transfer of training and codebook data. Numerical simulations demonstrate the promising performance of the proposed method.
更多查看译文
关键词
Projected gradient descent,Lloyd-Max quanti-zation,neural network classification,feedback codebook design,frequency division duplexing
AI 理解论文
溯源树
样例
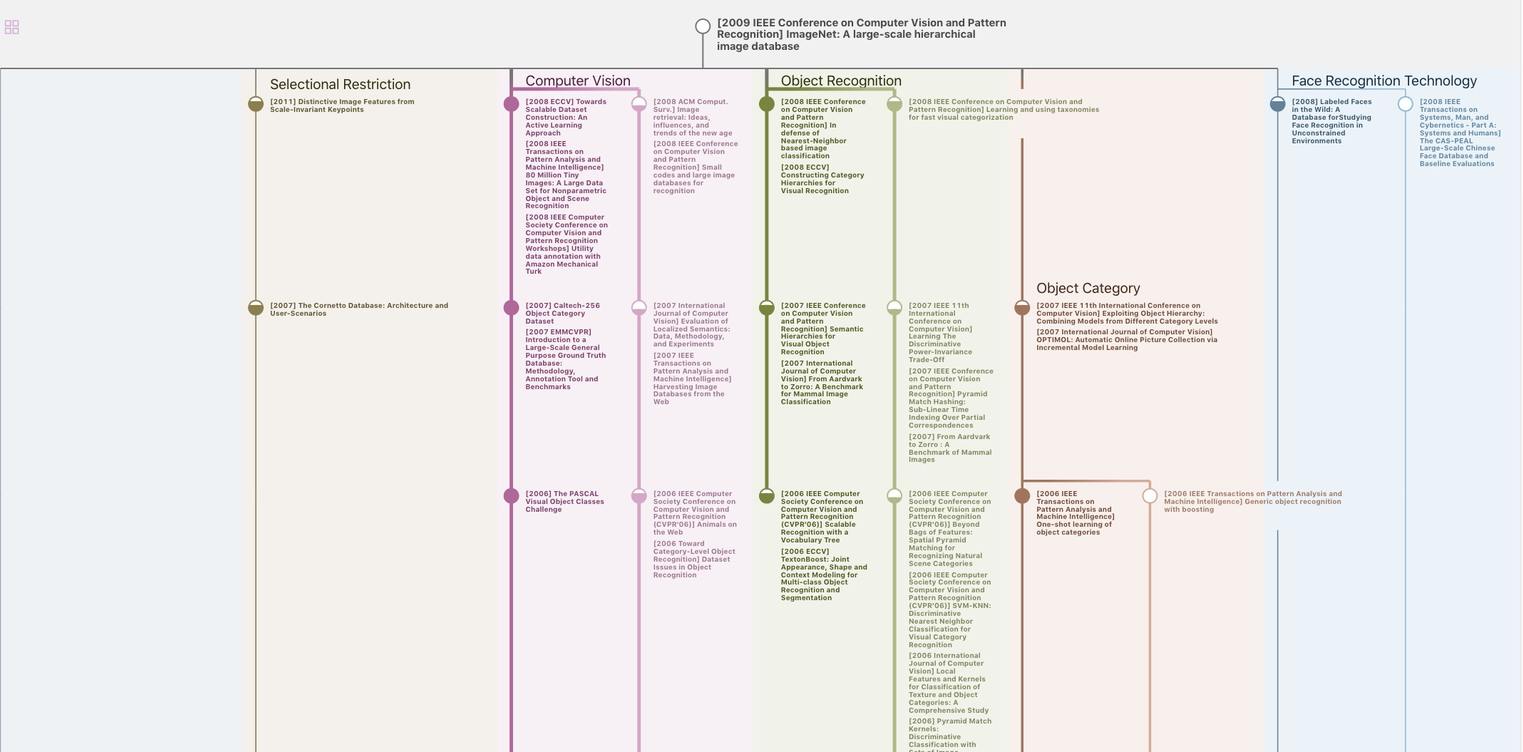
生成溯源树,研究论文发展脉络
Chat Paper
正在生成论文摘要