Reducing the Complexity of Model-Based MRI Reconstructions via Sparsification
IEEE Transactions on Medical Imaging(2021)
摘要
Model-based reconstruction methods have emerged as a powerful alternative to classical Fourier-based MRI techniques, largely because of their ability to explicitly model (and therefore, potentially overcome) moderate field inhomogeneities, streamline reconstruction from non-Cartesian sampling, and even allow for the use of custom designed non-Fourier encoding methods. Their application in such scenarios, however, often comes with a substantial increase in computational cost, owing to the fact that the corresponding forward model in such settings no longer possesses a direct Fourier Transform based implementation. This paper introduces an algorithmic framework designed to reduce the computational burden associated with model-based MRI reconstruction tasks. The key innovation is the strategic sparsification of the corresponding forward operators for these models, giving rise to approximations of the forward models (and their adjoints) that admit low computational complexity application. This enables overall a reduced computational complexity application of popular iterative first-order reconstruction methods for these reconstruction tasks. Computational results obtained on both synthetic and experimental data illustrate the viability and efficiency of the approach.
更多查看译文
关键词
Algorithms,Fourier Analysis,Image Processing, Computer-Assisted,Magnetic Resonance Imaging,Tomography, X-Ray Computed
AI 理解论文
溯源树
样例
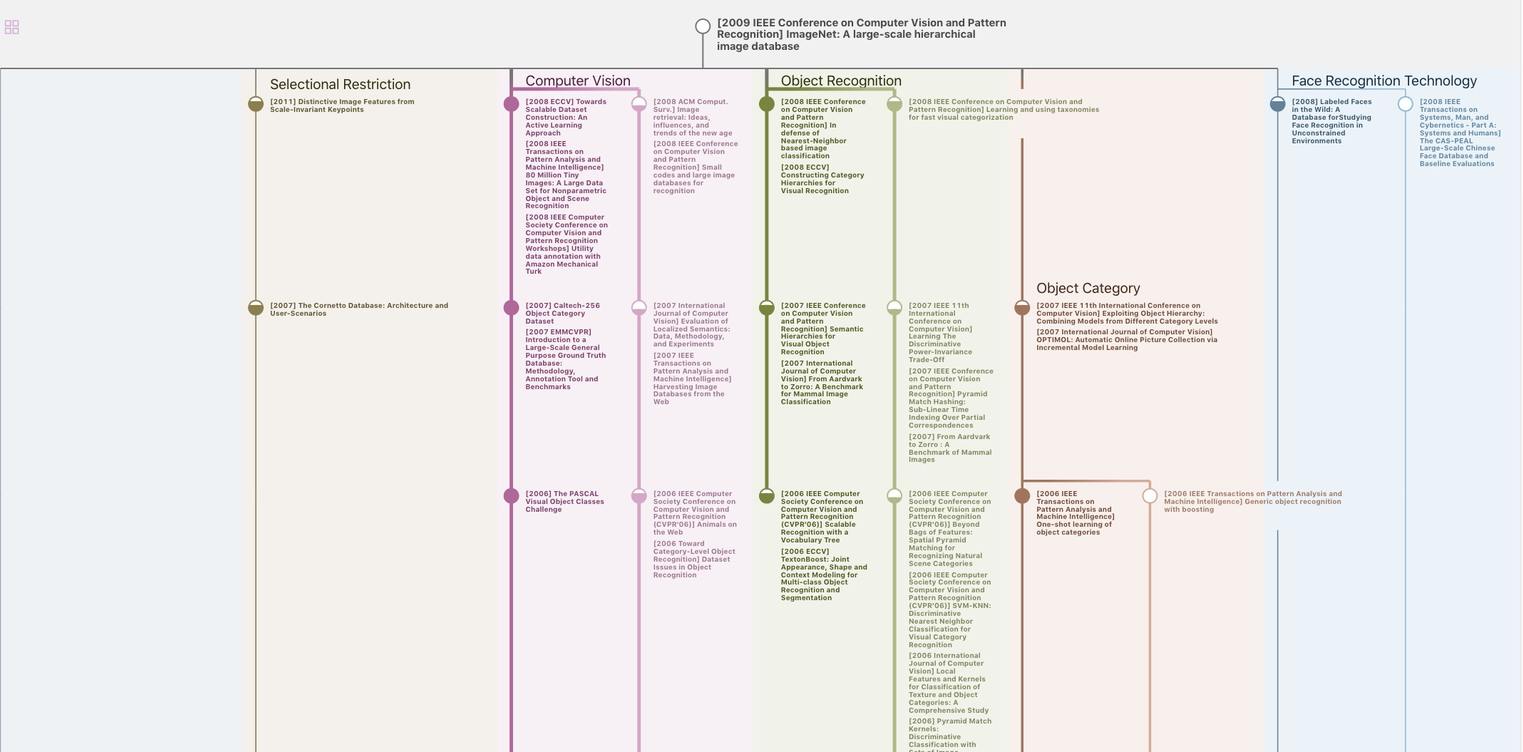
生成溯源树,研究论文发展脉络
Chat Paper
正在生成论文摘要