Effective Attention Sheds Light On Interpretability.
ACL/IJCNLP(2021)
摘要
An attention matrix of a transformer self-attention sublayer can provably be decomposed into two components and only one of them (effective attention) contributes to the model output. This leads us to ask whether visualizing effective attention gives different conclusions than interpretation of standard attention. Using a subset of the GLUE tasks and BERT, we carry out an analysis to compare the two attention matrices, and show that their interpretations differ. Effective attention is less associated with the features related to the language modeling pretraining such as the separator token, and it has more potential to illustrate linguistic features captured by the model for solving the end-task. Given the found differences, we recommend using effective attention for studying a transformer's behavior since it is more pertinent to the model output by design.
更多查看译文
关键词
interpretability,effective attention
AI 理解论文
溯源树
样例
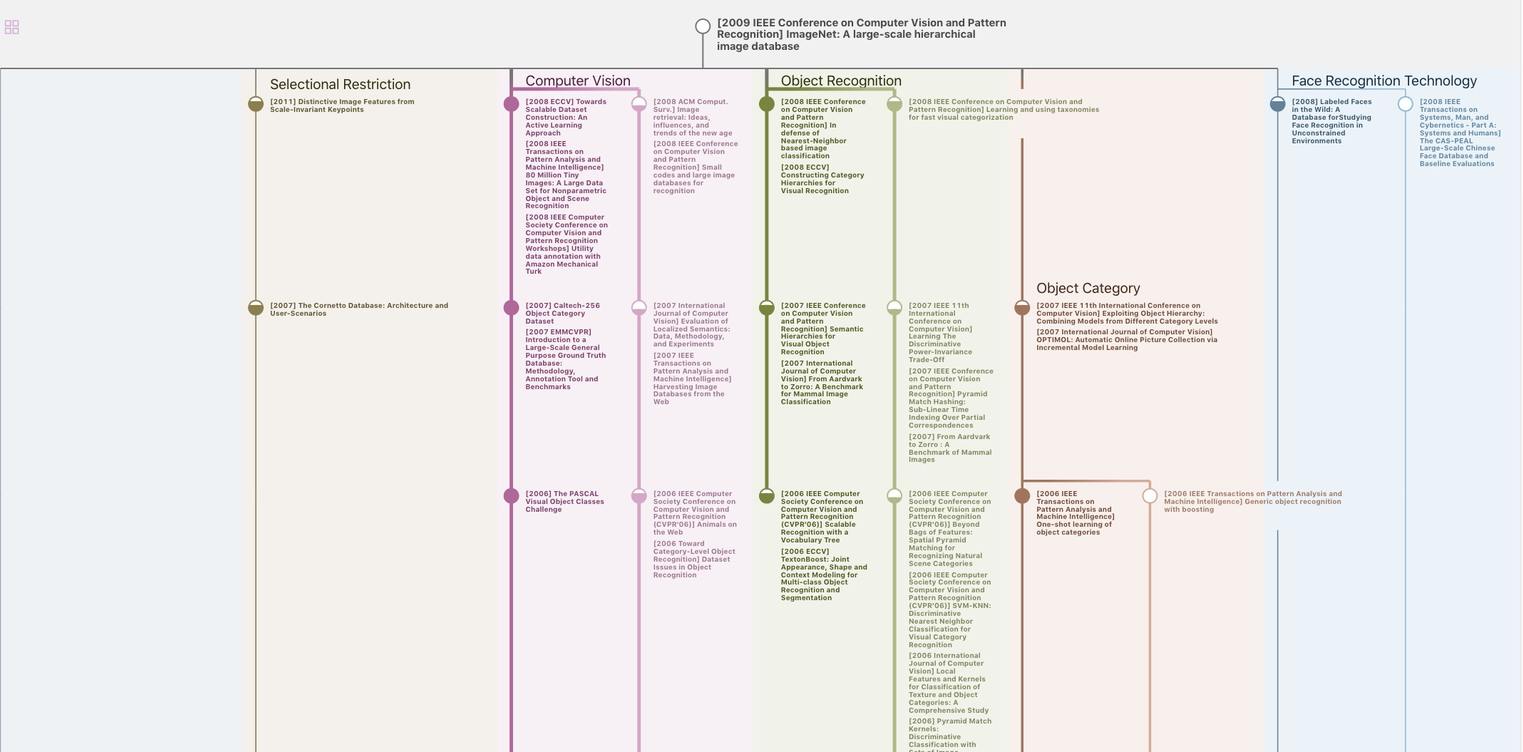
生成溯源树,研究论文发展脉络
Chat Paper
正在生成论文摘要