The Computational Complexity of ReLU Network Training Parameterized by Data Dimensionality
JOURNAL OF ARTIFICIAL INTELLIGENCE RESEARCH(2022)
摘要
Understanding the computational complexity of training simple neural networks with rectified linear units (ReLUs) has recently been a subject of intensive research. Closing gaps and complementing results from the literature, we present several results on the parameterized complexity of training two-layer ReLU networks with respect to various loss functions. After a brief discussion of other parameters, we focus on analyzing the influence of the dimension d of the training data on the computational complexity. We provide running time lower bounds in terms of W[1]-hardness for parameter d and prove that known brute-force strategies are essentially optimal (assuming the Exponential Time Hypothesis). In comparison with previous work, our results hold for a broad(er) range of loss functions, including l(p)-loss for all p is an element of [0, infinity]. In particular, we improve a known polynomial-time algorithm for constant d and convex loss functions to a more general class of loss functions, matching our running time lower bounds also in these cases.
更多查看译文
AI 理解论文
溯源树
样例
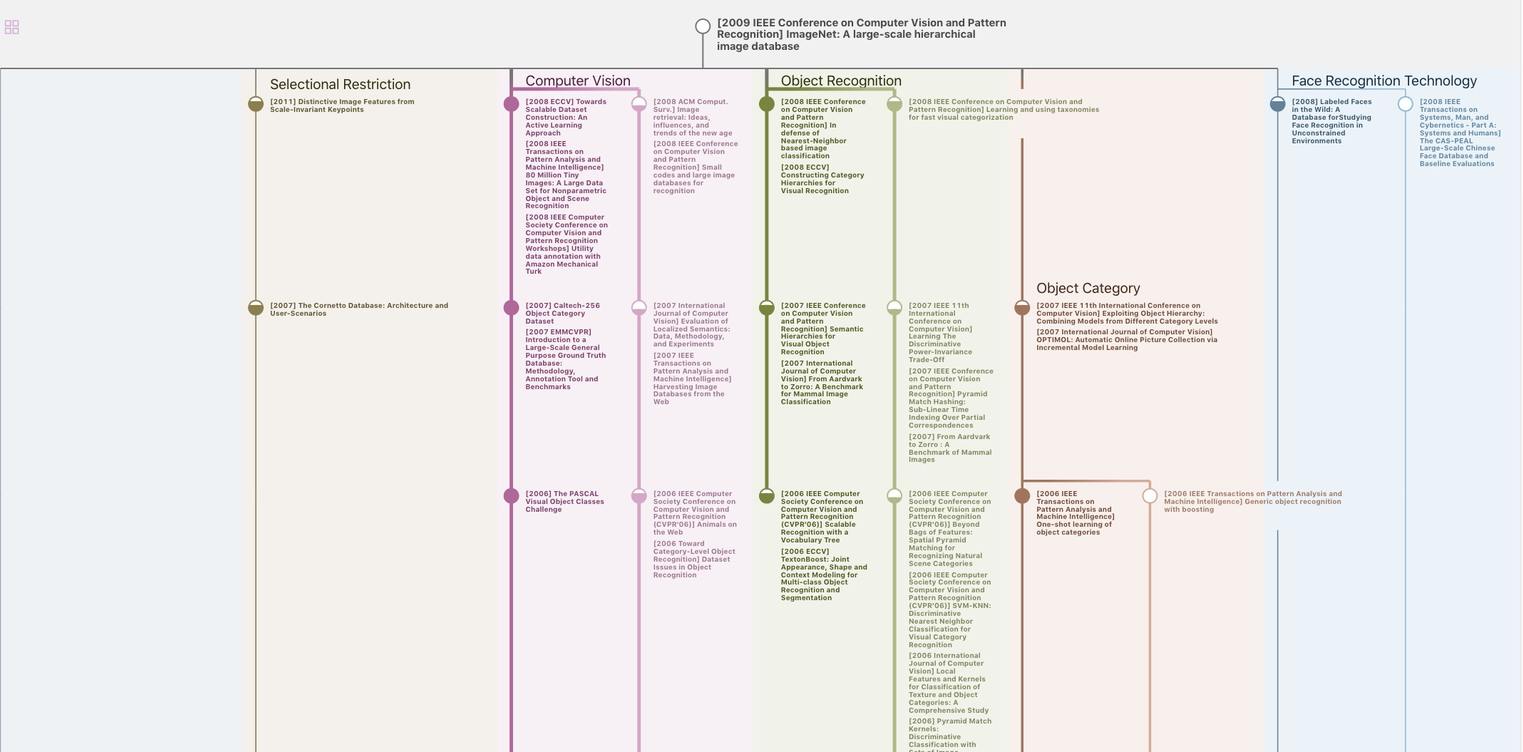
生成溯源树,研究论文发展脉络
Chat Paper
正在生成论文摘要