IntFormer: Predicting pedestrian intention with the aid of the Transformer architecture
arxiv(2021)
摘要
Understanding pedestrian crossing behavior is an essential goal in intelligent vehicle development, leading to an improvement in their security and traffic flow. In this paper, we developed a method called IntFormer. It is based on transformer architecture and a novel convolutional video classification model called RubiksNet. Following the evaluation procedure in a recent benchmark, we show that our model reaches state-of-the-art results with good performance ($\approx 40$ seq. per second) and size ($8\times $smaller than the best performing model), making it suitable for real-time usage. We also explore each of the input features, finding that ego-vehicle speed is the most important variable, possibly due to the similarity in crossing cases in PIE dataset.
更多查看译文
关键词
pedestrian intention,intformer,transformer architecture
AI 理解论文
溯源树
样例
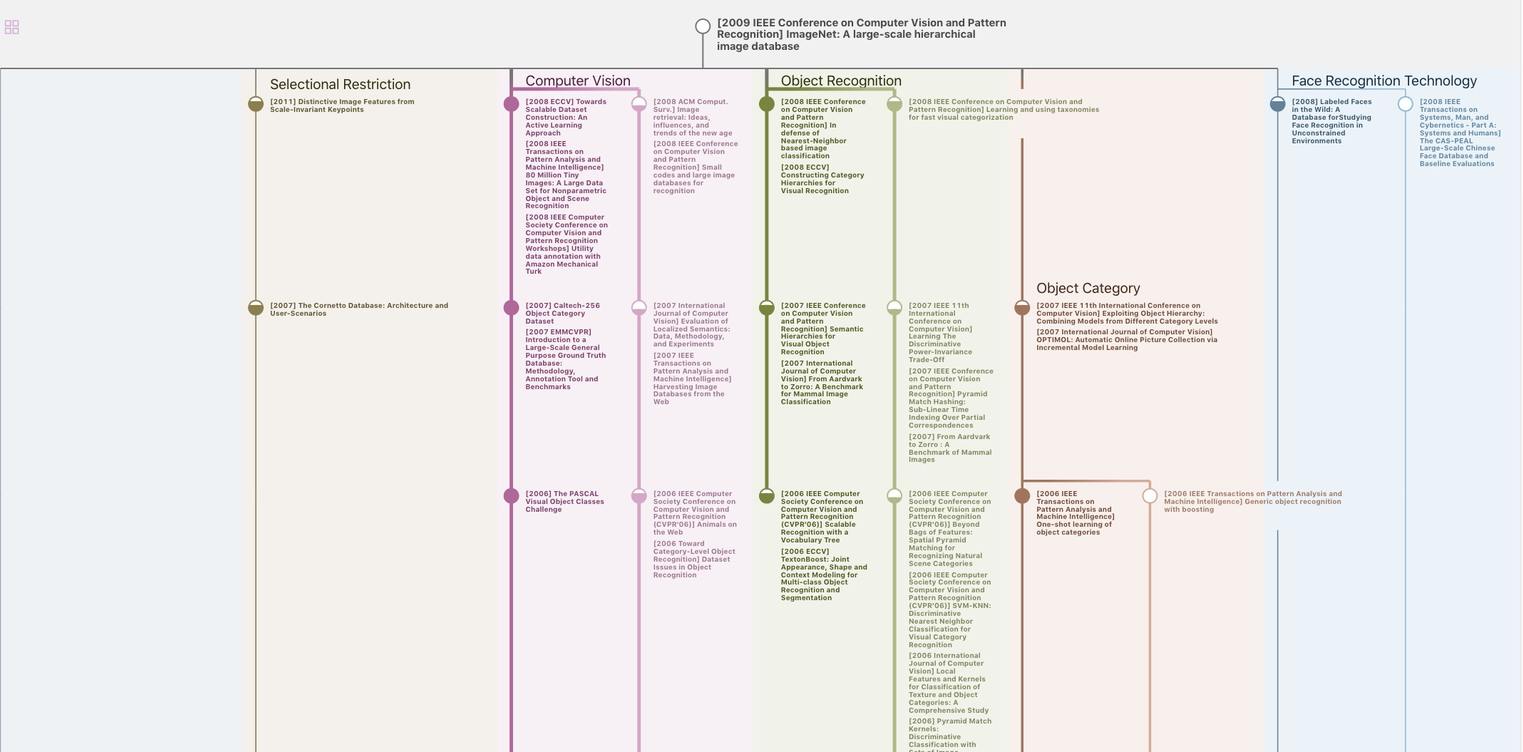
生成溯源树,研究论文发展脉络
Chat Paper
正在生成论文摘要