Contrastive Model Invertion for Data-Free Knolwedge Distillation.
IJCAI(2021)
摘要
Model inversion, whose goal is to recover training data from a pre-trained model, has been recently proved feasible. However, existing inversion methods usually suffer from the mode collapse problem, where the synthesized instances are highly similar to each other and thus show limited effectiveness for downstream tasks, such as knowledge distillation. In this paper, we propose Contrastive Model Inversion~(CMI), where the data diversity is explicitly modeled as an optimizable objective, to alleviate the mode collapse issue. Our main observation is that, under the constraint of the same amount of data, higher data diversity usually indicates stronger instance discrimination. To this end, we introduce in CMI a contrastive learning objective that encourages the synthesizing instances to be distinguishable from the already synthesized ones in previous batches. Experiments of pre-trained models on CIFAR-10, CIFAR-100, and Tiny-ImageNet demonstrate that CMI not only generates more visually plausible instances than the state of the arts, but also achieves significantly superior performance when the generated data are used for knowledge distillation. Code is available at \url{https://github.com/zju-vipa/DataFree}.
更多查看译文
关键词
inversion,knowledge,model,data-free
AI 理解论文
溯源树
样例
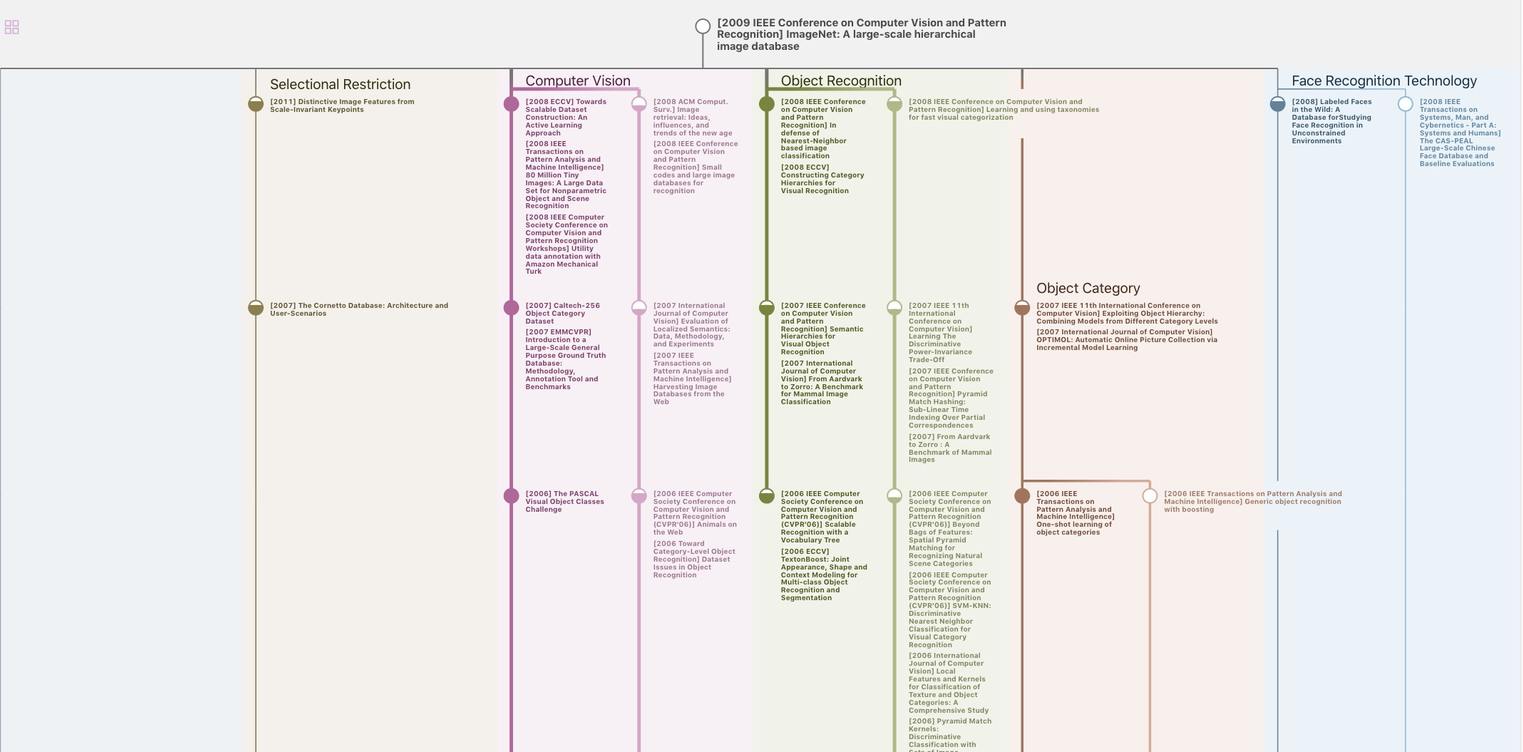
生成溯源树,研究论文发展脉络
Chat Paper
正在生成论文摘要