Differentiable Factor Graph Optimization for Learning Smoothers
2021 IEEE/RSJ INTERNATIONAL CONFERENCE ON INTELLIGENT ROBOTS AND SYSTEMS (IROS)(2021)
摘要
A recent line of work has shown that end-to-end optimization of Bayesian filters can be used to learn state estimators for systems whose underlying models are difficult to hand-design or tune, while retaining the core advantages of probabilistic state estimation. As an alternative approach for state estimation in these settings, we present an end-to-end approach for learning state estimators modeled as factor graph-based smoothers. By unrolling the optimizer we use for maximum a posteriori inference in these probabilistic graphical models, our method is able to learn probabilistic system models in the full context of an overall state estimator, while also taking advantage of the distinct accuracy and runtime advantages that smoothers offer over recursive filters. We study our approach using two fundamental state estimation problems, object tracking and visual odometry, where we demonstrate a significant improvement over existing baselines. Our work comes with an extensive code release, which includes training and evaluation scripts, as well as Python libraries for Lie theory and factor graph optimization: https://sites.google.com/view/diffsmoothing/.
更多查看译文
关键词
differentiable factor graph optimization,end-to-end optimization,probabilistic state estimation,factor graph-based smoothers,probabilistic graphical models,probabilistic system models,learning smoothers,Bayesian filters,visual odometry,object tracking,Python libraries,Lie theory,factor graph optimization
AI 理解论文
溯源树
样例
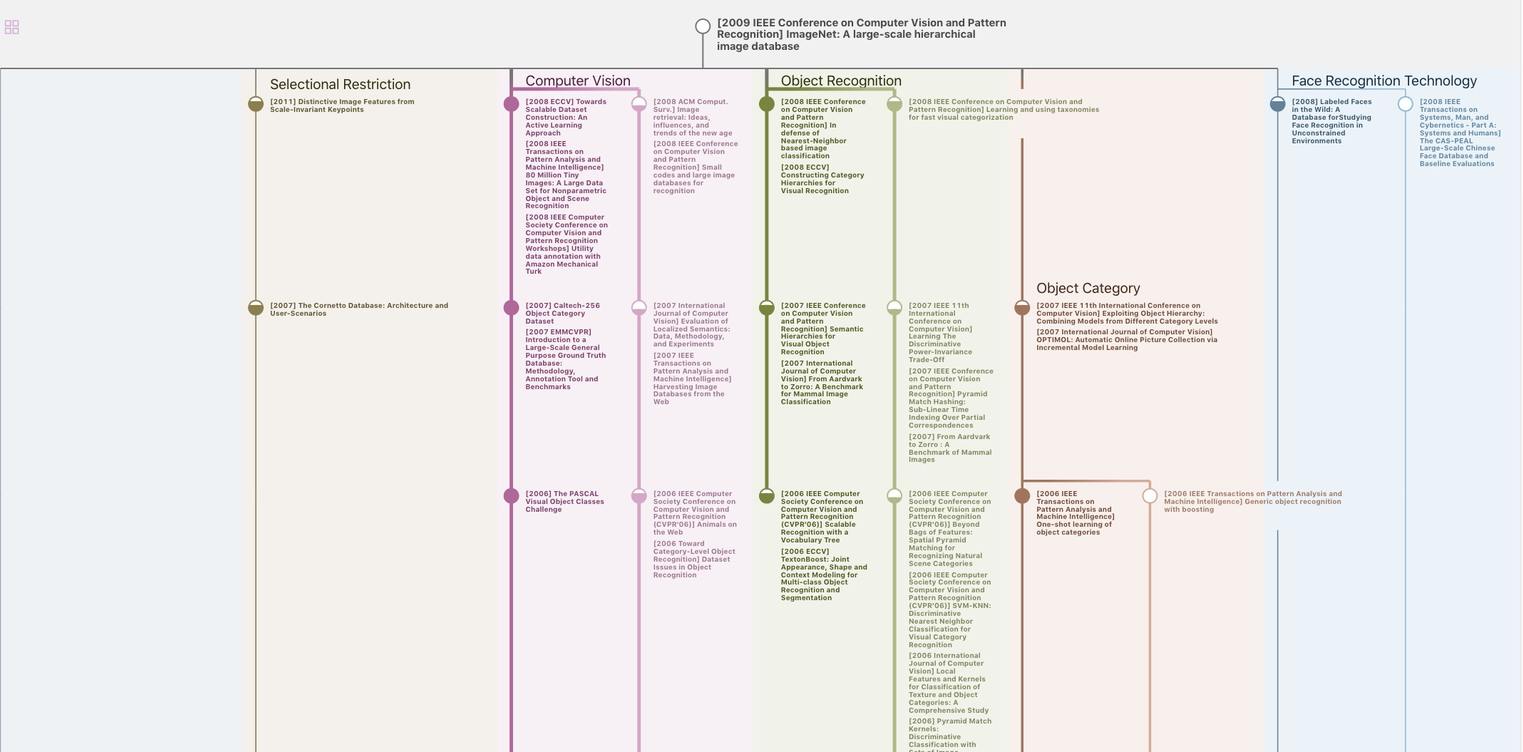
生成溯源树,研究论文发展脉络
Chat Paper
正在生成论文摘要