Joint Optimization of Hadamard Sensing and Reconstruction in Compressed Sensing Fluorescence Microscopy
MEDICAL IMAGE COMPUTING AND COMPUTER ASSISTED INTERVENTION - MICCAI 2021, PT VI(2021)
摘要
Compressed sensing fluorescence microscopy (CS-FM) proposes a scheme whereby less measurements are collected during sensing and reconstruction is performed to recover the image. Much work has gone into optimizing the sensing and reconstruction portions separately. We propose a method of jointly optimizing both sensing and reconstruction end-to-end under a total measurement constraint, enabling learning of the optimal sensing scheme concurrently with the parameters of a neural network-based reconstruction network. We train our model on a rich dataset of confocal, two-photon, and wide-field microscopy images comprising of a variety of biological samples. We show that our method outperforms several baseline sensing schemes and a regularized regression reconstruction algorithm. Our code is publicly available at https://github.com/alanqrwang/csfm.
更多查看译文
关键词
Fluorescence microscopy, Compressed sensing, Joint optimization
AI 理解论文
溯源树
样例
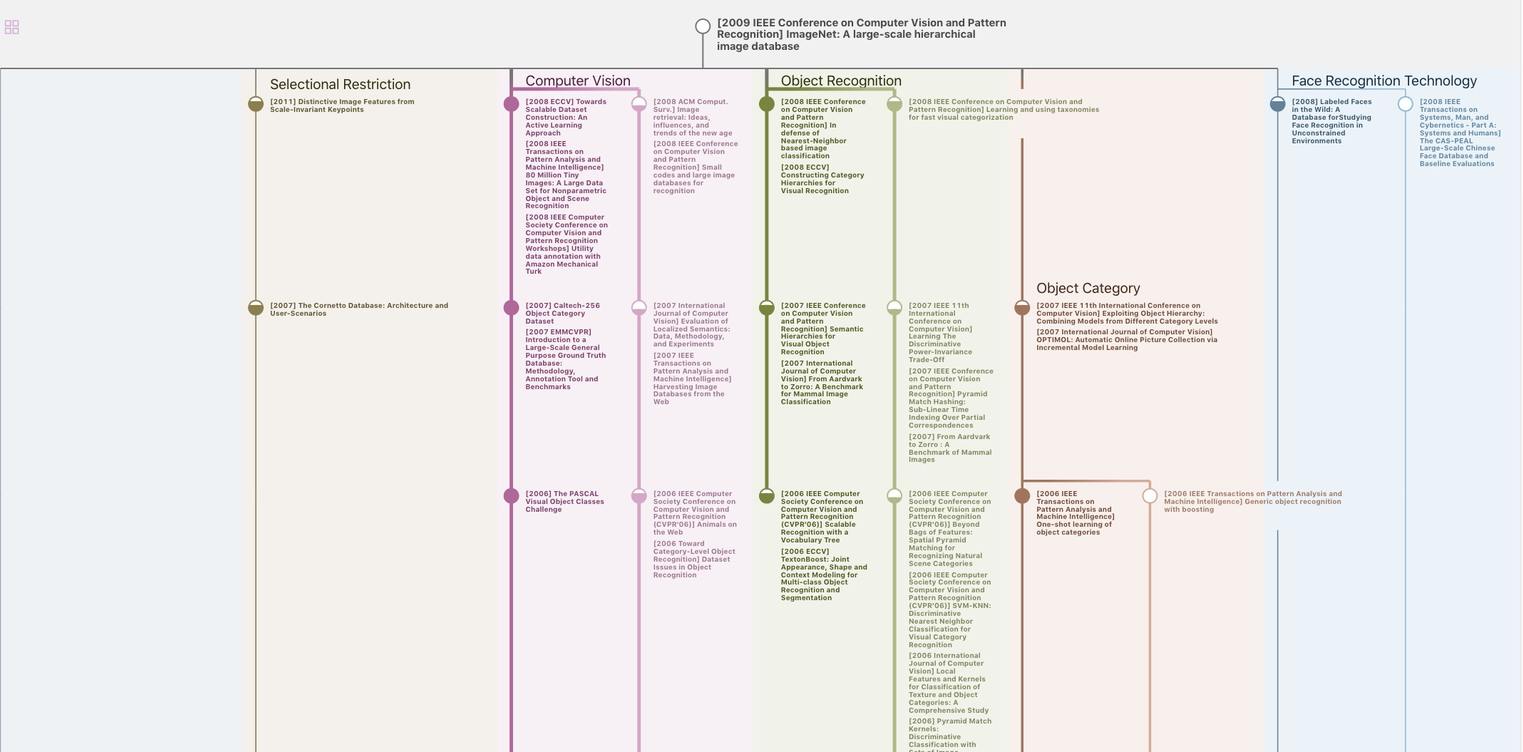
生成溯源树,研究论文发展脉络
Chat Paper
正在生成论文摘要