Group-wise shrinkage for multiclass Gaussian Graphical Models
arxiv(2021)
摘要
Gaussian Graphical Models are widely employed for modelling dependence among variables. Likewise, finite Gaussian mixtures are often the standard way to go for model-based clustering of continuous features. With the increasing availability of high-dimensional datasets, a methodological link between these two approaches has been established in order to provide a framework for performing penalized model-based clustering in the presence of large precision matrices. Notwithstanding, current methodologies do not account for the fact that groups may possess different degrees of association among the variables, thus implicitly assuming similar levels of sparsity across the classes. We overcome this limitation by deriving group-wise penalty factors, automatically enforcing under or over-connectivity in the estimated graphs. The approach is entirely data-driven and does not require any additional hyper-parameter specification. Simulated data experiments showcase the validity of our proposal.
更多查看译文
关键词
multiclass gaussian graphical models,group-wise
AI 理解论文
溯源树
样例
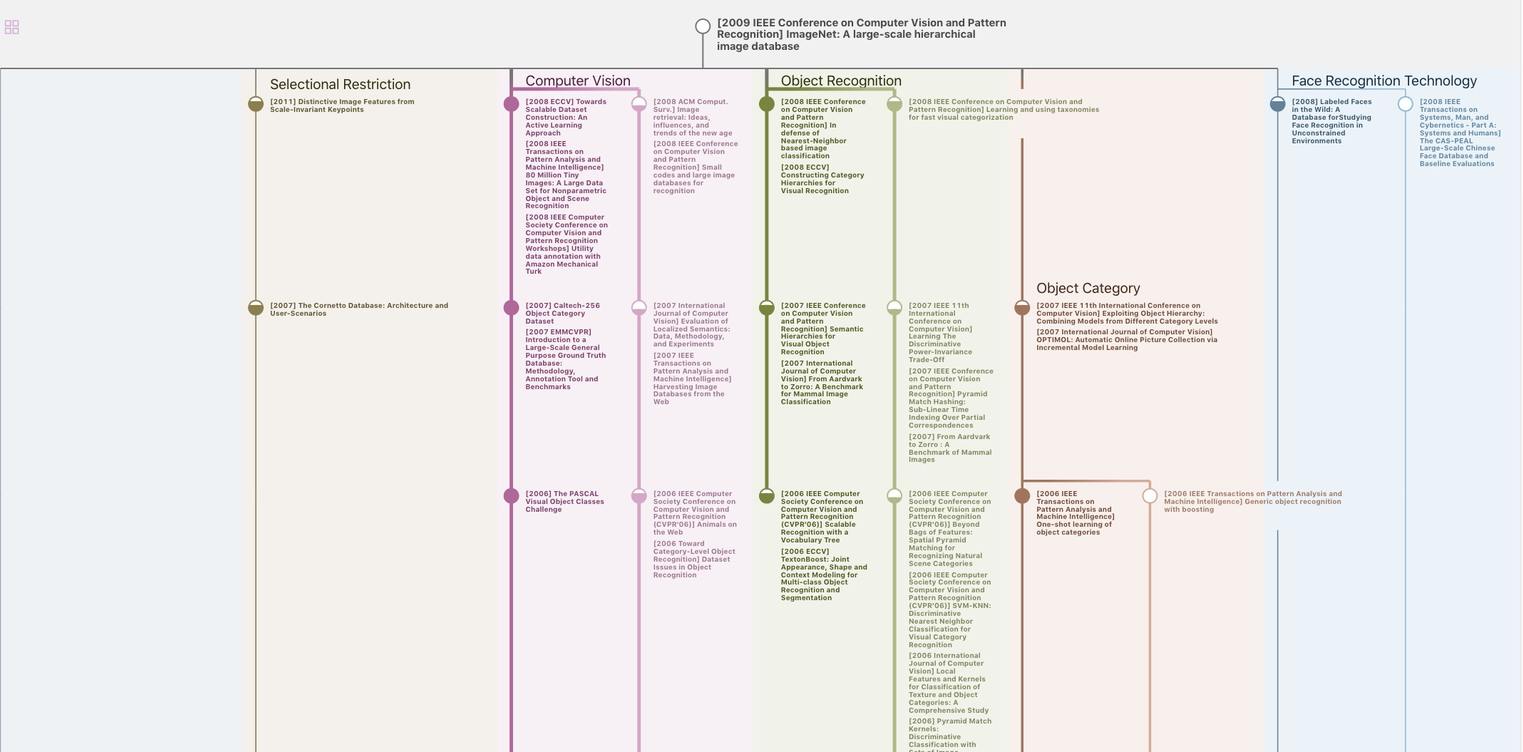
生成溯源树,研究论文发展脉络
Chat Paper
正在生成论文摘要