Substitutional Neural Image Compression
arxiv(2021)
摘要
We describe Substitutional Neural Image Compression (SNIC), a general approach for enhancing any neural image compression model, that requires no data or additional tuning of the trained model. It boosts compression performance toward a flexible distortion metric and enables bit-rate control using a single model instance. The key idea is to replace the image to be compressed with a substitutional one that outperforms the original one in a desired way. Finding such a substitute is inherently difficult for conventional codecs, yet surprisingly favorable for neural compression models thanks to their fully differentiable structures. With gradients of a particular loss backpropogated to the input, a desired substitute can be efficiently crafted iteratively. We demonstrate the effectiveness of SNIC, when combined with various neural compression models and target metrics, in improving compression quality and performing bit-rate control measured by rate-distortion curves. Empirical results of control precision and generation speed are also discussed.
更多查看译文
关键词
Image Compression,Online Training,substitution
AI 理解论文
溯源树
样例
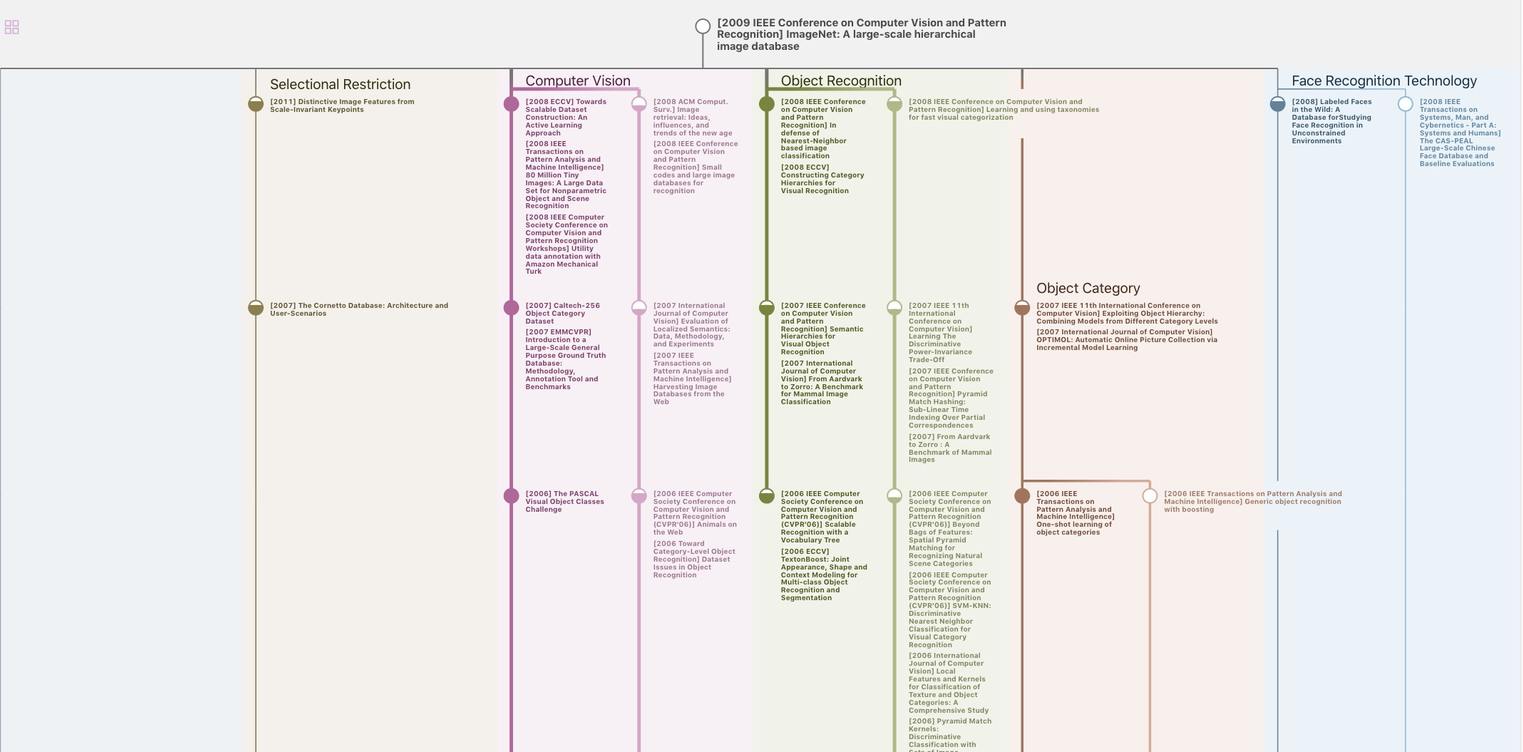
生成溯源树,研究论文发展脉络
Chat Paper
正在生成论文摘要