Sobolev Norm Learning Rates for Conditional Mean Embeddings
INTERNATIONAL CONFERENCE ON ARTIFICIAL INTELLIGENCE AND STATISTICS, VOL 151(2022)
摘要
We develop novel learning rates for conditional mean embeddings by applying the theory of interpolation for reproducing kernel Hilbert spaces (RKHS). We derive explicit, adaptive convergence rates for the sample estimator under the misspecifed setting, where the target operator is not Hilbert-Schmidt or bounded with respect to the input/output RKHSs. We demonstrate that in certain parameter regimes, we can achieve uniform convergence rates in the output RKHS. We hope our analyses will allow the much broader application of conditional mean embeddings to more complex ML/RL settings involving infinite dimensional RKHSs and continuous state spaces.
更多查看译文
关键词
conditional mean embeddings,learning
AI 理解论文
溯源树
样例
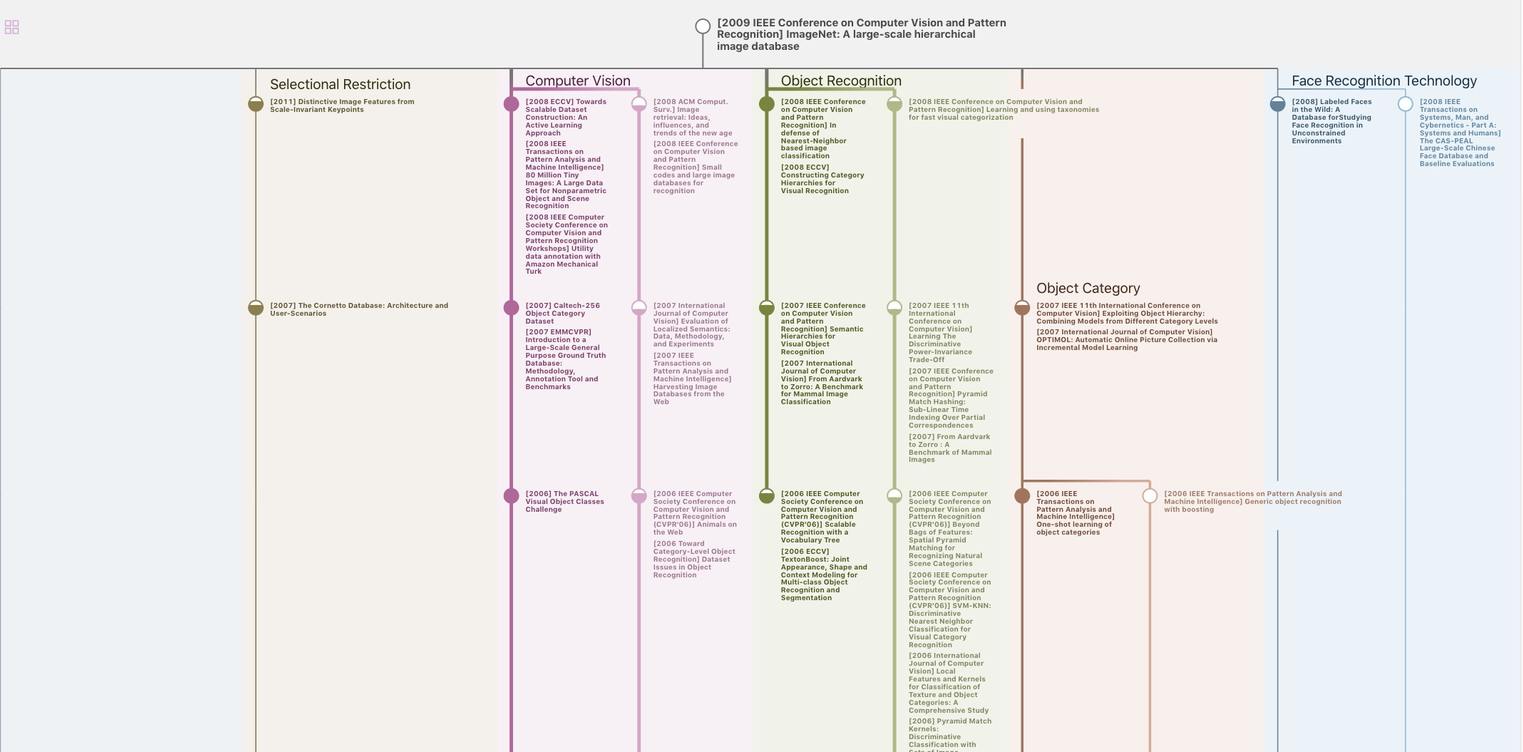
生成溯源树,研究论文发展脉络
Chat Paper
正在生成论文摘要