Posterior Contraction for Deep Gaussian Process Priors
JOURNAL OF MACHINE LEARNING RESEARCH(2023)
摘要
We study posterior contraction rates for a class of deep Gaussian process priors in the nonparametric regression setting under a general composition assumption on the regression function. It is shown that the contraction rates can achieve the minimax convergence rate (up to log n factors), while being adaptive to the underlying structure and smoothness of the target function. The proposed framework extends the Bayesian nonparametric theory for Gaussian process priors.
更多查看译文
关键词
Bayesian nonparametric regression,contraction rates,deep Gaussian processes,uncertainty quantification,neural networks
AI 理解论文
溯源树
样例
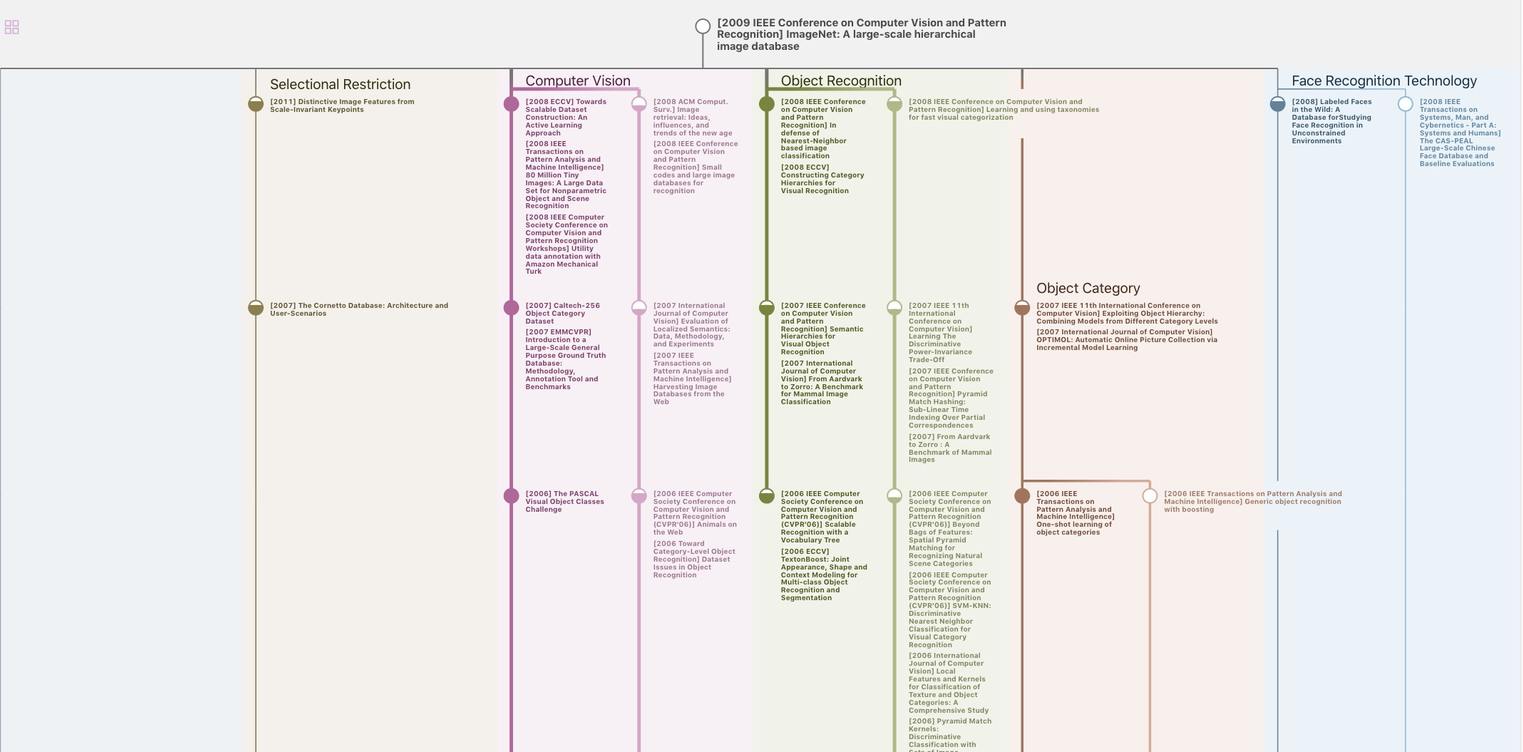
生成溯源树,研究论文发展脉络
Chat Paper
正在生成论文摘要