Mean Shift for Self-Supervised Learning.
ICCV(2021)
摘要
Most recent self-supervised learning (SSL) algorithms learn features by contrasting between instances of images or by clustering the images and then contrasting between the image clusters. We introduce a simple mean-shift algorithm that learns representations by grouping images together without contrasting between them or adopting much of prior on the structure of the clusters. We simply "shift" the embedding of each image to be close to the "mean" of its neighbors. Since in our setting, the closest neighbor is always another augmentation of the same image, our model will be identical to BYOL when using only one nearest neighbor instead of 5 as used in our experiments. Our model achieves 72.4% on ImageNet linear evaluation with ResNet50 at 200 epochs outperforming BYOL. Our code is available here: https://github.com/UMBCvision/MSF
更多查看译文
关键词
learning,mean,self-supervised
AI 理解论文
溯源树
样例
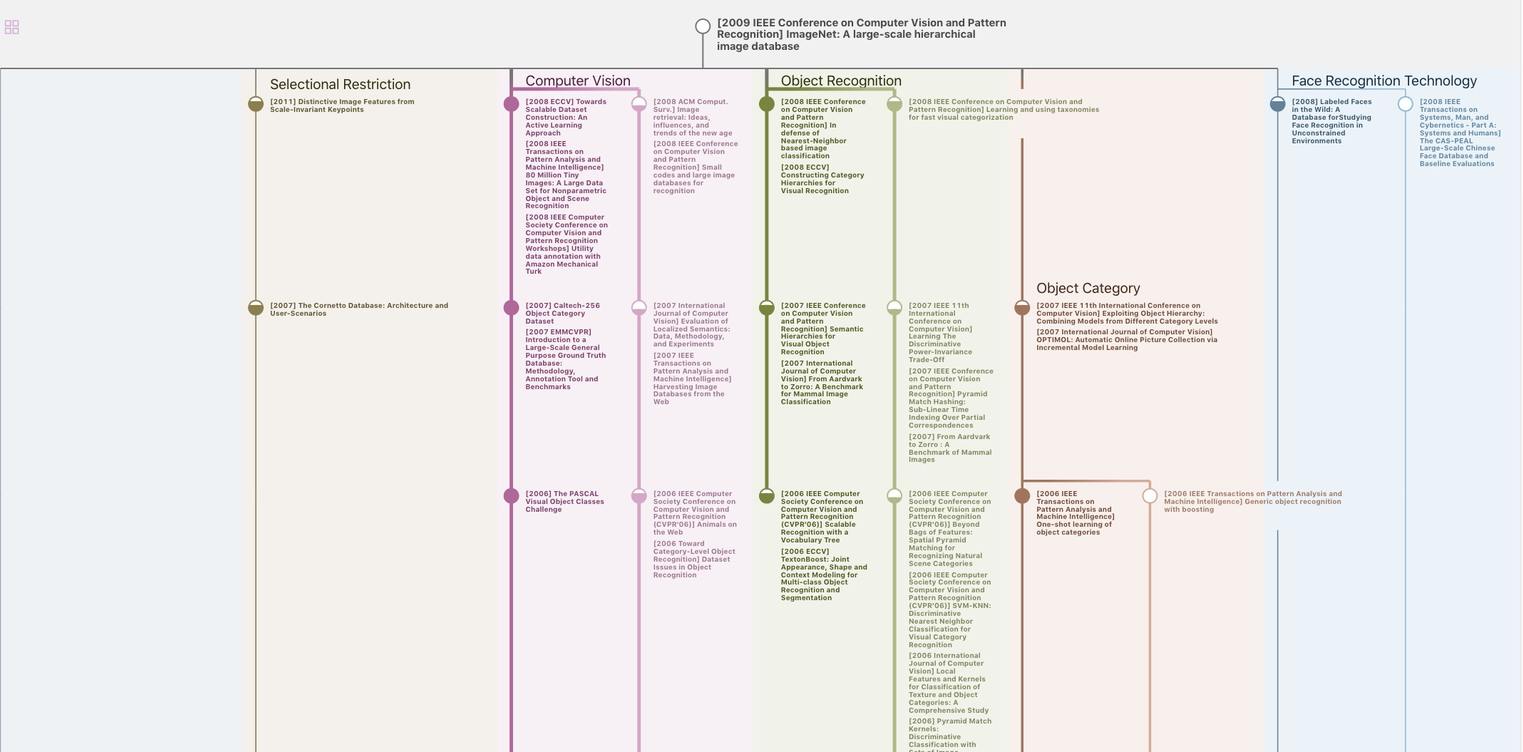
生成溯源树,研究论文发展脉络
Chat Paper
正在生成论文摘要