The Low-Dimensional Linear Geometry of Contextualized Word Representations.
Conference on Computational Natural Language Learning(2021)
摘要
Black-box probing models can reliably extract linguistic features like tense, number, and syntactic role from pretrained word representations. However, the manner in which these features are encoded in representations remains poorly understood. We present a systematic study of the linear geometry of contextualized word representations in ELMO and BERT. We show that a variety of linguistic features (including structured dependency relationships) are encoded in low-dimensional subspaces. We then refine this geometric picture, showing that there are hierarchical relations between the subspaces encoding general linguistic categories and more specific ones, and that low-dimensional feature encodings are distributed rather than aligned to individual neurons. Finally, we demonstrate that these linear subspaces are causally related to model behavior, and can be used to perform fine-grained manipulation of BERT's output distribution.
更多查看译文
关键词
contextualized word
AI 理解论文
溯源树
样例
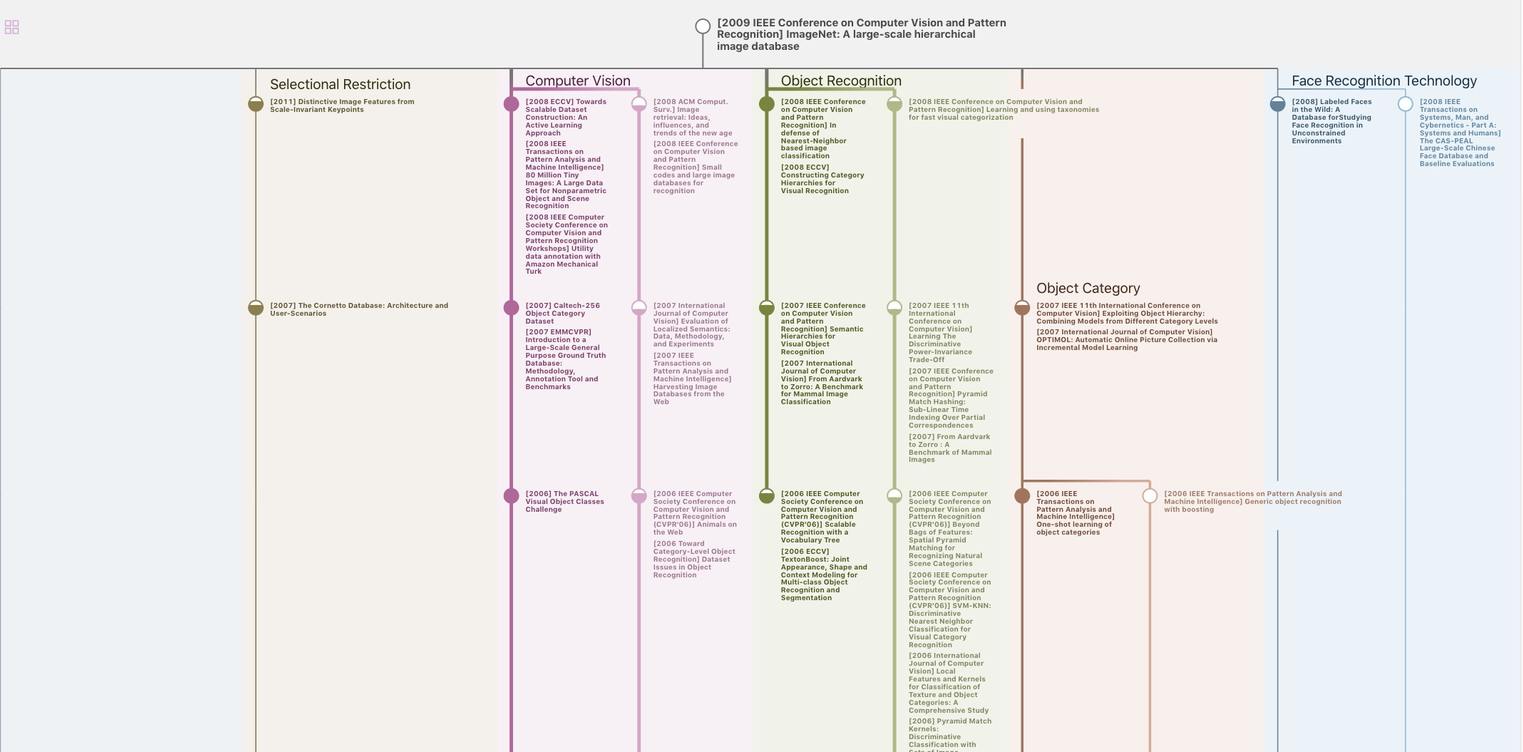
生成溯源树,研究论文发展脉络
Chat Paper
正在生成论文摘要