Cause and Effect - Concept-based Explanation of Neural Networks.
SMC(2021)
摘要
In many scenarios, human decisions are explained based on some high-level concepts. In this work, we take a step in the interpretability of neural networks by examining their internal representation or neuron's activations against concepts. A concept is characterized by a set of samples that have specific features in common. We propose a framework to check the existence of a causal relationship between a concept (or its negation) and task classes. While the previous methods focus on the importance of a concept to a task class, we go further and introduce four measures to quantitatively determine the order of causality. Through experiments, we demonstrate the effectiveness of the proposed method in explaining the relationship between a concept and the predictive behaviour of a neural network.
更多查看译文
关键词
predictive behaviour,causality order,neuron activations,task classes,causal relationship,internal representation,high-level concepts,human decisions,neural network,concept-based explanation
AI 理解论文
溯源树
样例
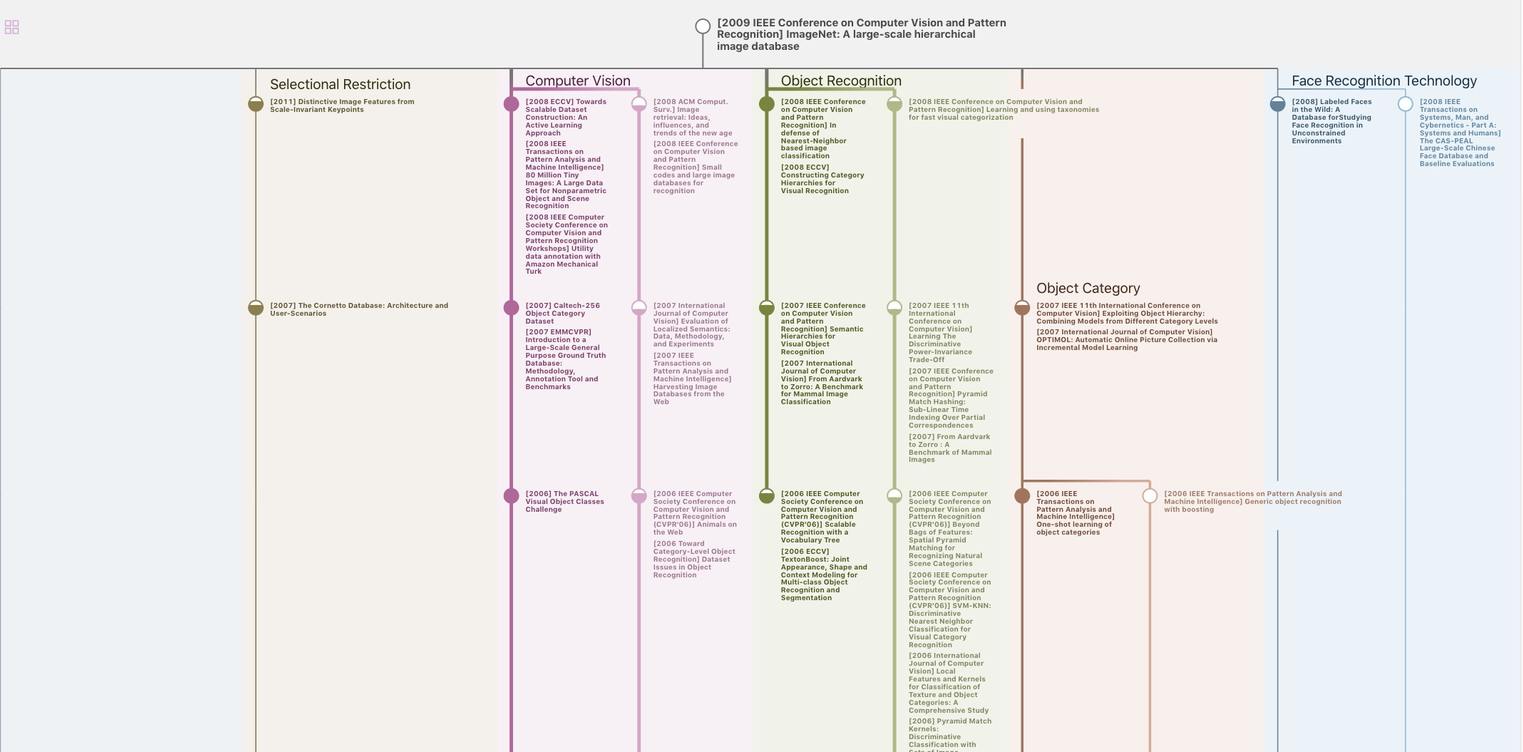
生成溯源树,研究论文发展脉络
Chat Paper
正在生成论文摘要