QAConv: Question Answering on Informative Conversations
PROCEEDINGS OF THE 60TH ANNUAL MEETING OF THE ASSOCIATION FOR COMPUTATIONAL LINGUISTICS (ACL 2022), VOL 1: (LONG PAPERS)(2022)
摘要
This paper introduces QAConv, 1, a new question answering (QA) dataset that uses conversations as a knowledge source. We focus on informative conversations, including business emails, panel discussions, and work channels. Unlike open-domain and task-oriented dialogues, these conversations are usually long, complex, asynchronous, and involve strong domain knowledge. In total, we collect 34,608 QA pairs from 10,259 selected conversations with both human-written and machine-generated questions. We use a question generator and a dialogue summarizer as auxiliary tools to collect and recommend questions. The dataset has two testing scenarios: chunk mode and full mode, depending on whether the grounded partial conversation is provided or retrieved. Experimental results show that state-of-the-art pretrained QA systems have limited zero-shot performance and tend to predict our questions as unanswerable. Our dataset provides a new training and evaluation testbed to facilitate QA on conversations research.
更多查看译文
关键词
conversations
AI 理解论文
溯源树
样例
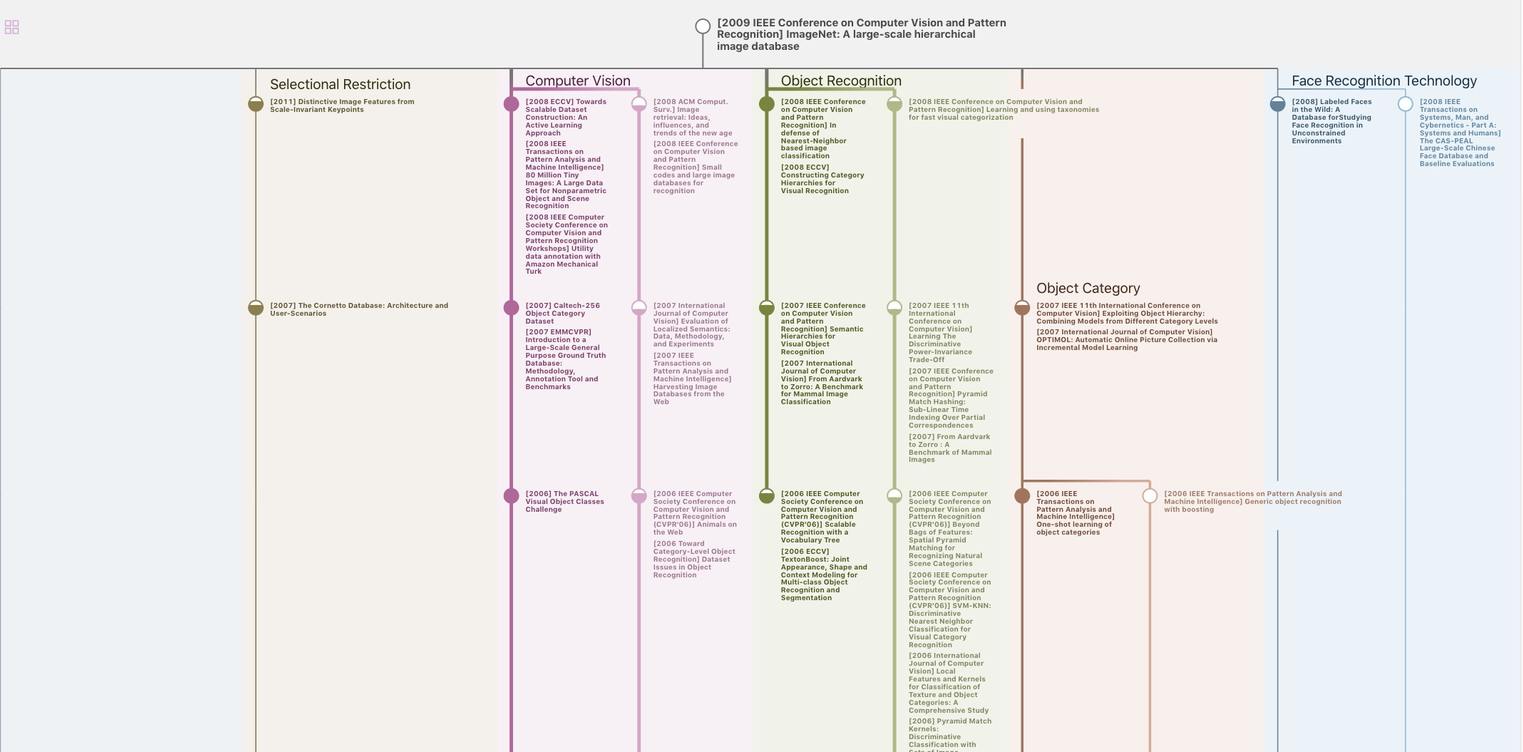
生成溯源树,研究论文发展脉络
Chat Paper
正在生成论文摘要