Importance Weighted Adversarial Discriminative Transfer for Anomaly Detection
arxiv(2021)
摘要
Previous transfer methods for anomaly detection generally assume the availability of labeled data in source or target domains. However, such an assumption is not valid in most real applications where large-scale labeled data are too expensive. Therefore, this paper proposes an importance weighted adversarial autoencoder-based method to transfer anomaly detection knowledge in an unsupervised manner, particularly for a rarely studied scenario where a target domain has no labeled normal/abnormal data while only normal data from a related source domain exist. Specifically, the method learns to align the distributions of normal data in both source and target domains, but leave the distribution of abnormal data in the target domain unchanged. In this way, an obvious gap can be produced between the distributions of normal and abnormal data in the target domain, therefore enabling the anomaly detection in the domain. Extensive experiments on multiple synthetic datasets and the UCSD benchmark demonstrate the effectiveness of our approach. The code is available at https://github.com/fancangning/anomaly_detection_transfer.
更多查看译文
关键词
anomaly
AI 理解论文
溯源树
样例
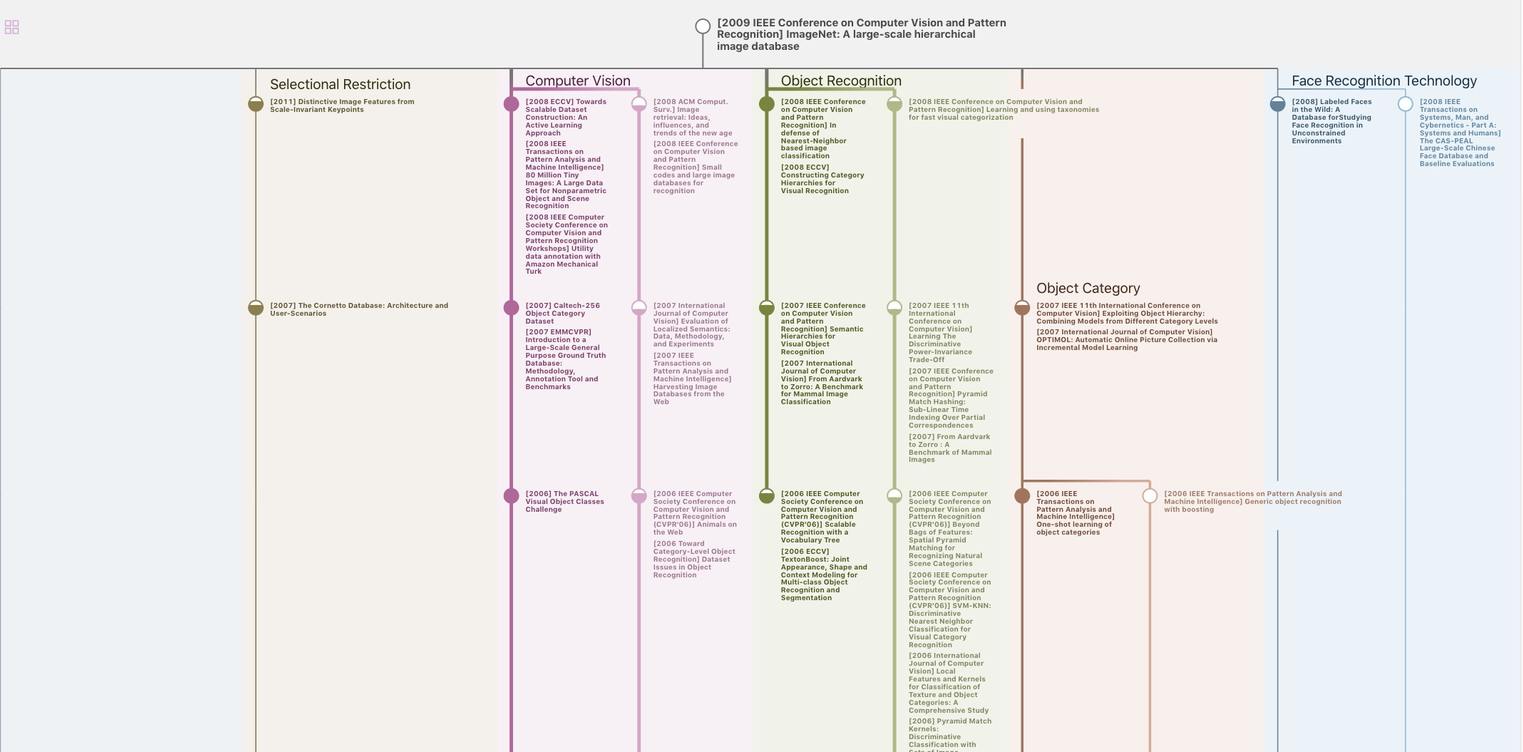
生成溯源树,研究论文发展脉络
Chat Paper
正在生成论文摘要