DISDi - Discontinuous Intervals in Subgroup Discovery.
RCIS(2021)
摘要
The subgroup discovery problem aims to identify, from data, a subset of objects which exhibit interesting characteristics according to a quality measure defined on a target attribute. Main approaches in this area make the implicit assumption that optimal subgroups emerge from continuous intervals. In this paper, we propose a new approach, called DISDi, for extracting subgroups in numerical data whose originality consists of searching for subgroups on discontinuous attribute intervals. The intuition behind this approach is that disjoint intervals allow refining the definition of subgroups and therefore the quality of the subgroups identified. Thus unlike the main algorithms in the field, the novelty of our proposal lies in the way it breaks down the intervals of the attributes during the subgroup research process. The algorithm also limits the exploration of the search space by exploiting the closure property and combining some branches. The efficiency of the proposal is demonstrated by comparing the results with two algorithms that are references in the field of several benchmark datasets.
更多查看译文
关键词
Data science,Subgroup discovery,Knowledge extraction,Algorithm
AI 理解论文
溯源树
样例
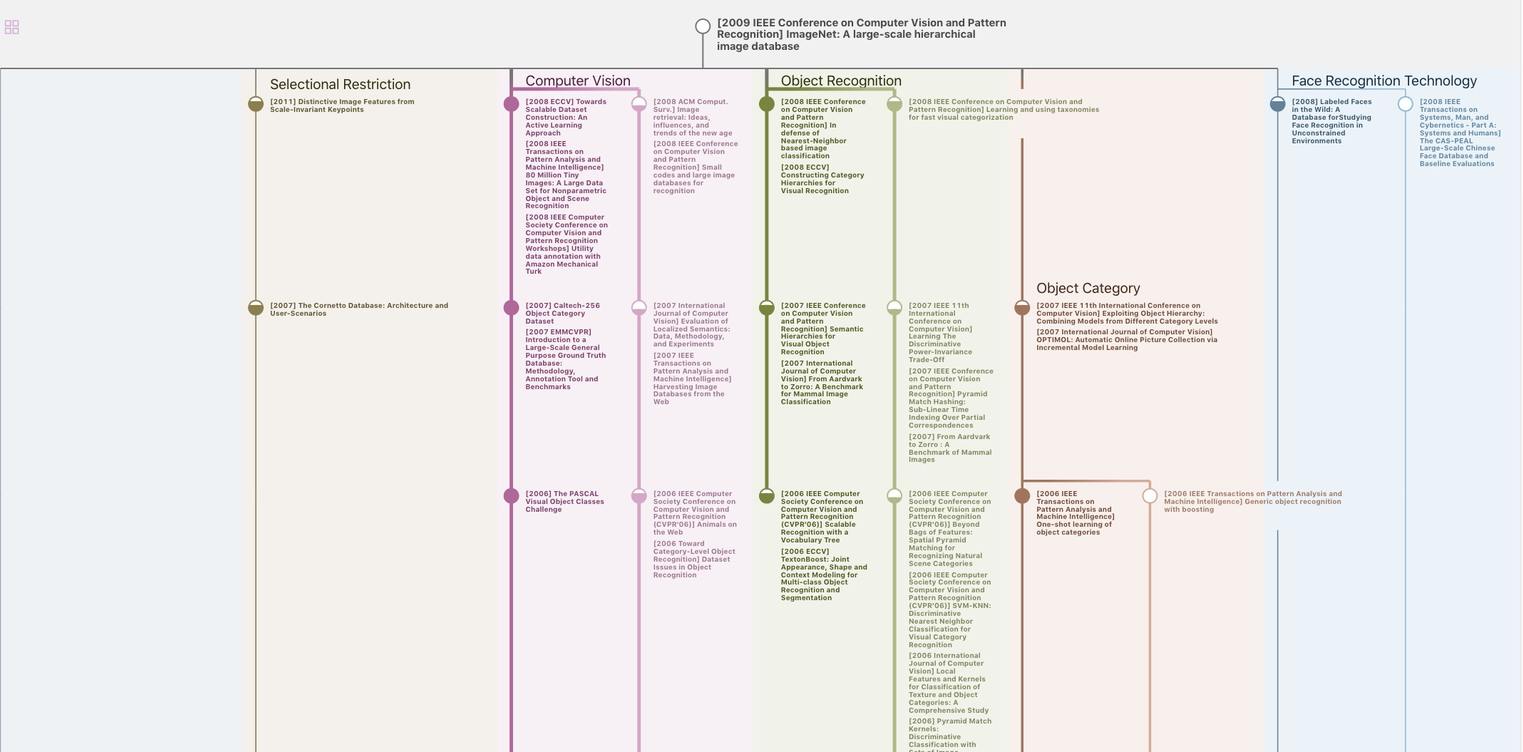
生成溯源树,研究论文发展脉络
Chat Paper
正在生成论文摘要