An efficient machine learning model for malicious activities recognition in water-based industrial internet of things
SECURITY AND PRIVACY(2021)
摘要
The Industrial Control System (ICS) has an important role to reduce the interaction between humans and the industrial system. Cyber-Physical Systems (CPSs) operate in critical infrastructures such as transport networks, gas, and water distribution networks, Unmanned Aerial Vehicle Systems (UASs), and nuclear power generation. In this paper, we provide a Machine Learning Model for Malicious Activities Recognition in Water-based Industrial Internet of Things (MARWIIoT). This model can help industrial operators and administrators to recognize any malicious activities against industrial infrastructure. To classify the malicious activity, various machine learning methods, such as Logistic Regression (LR), Linear Discriminant Analysis (LDA), k-nearest neighbors (KNN), Naive Bayes (NB), and Support Vector Machine (SVM) can be used. The proposed MARWIIoT model is based on Genetic-KNN with normalization to classify malicious activities efficiently. This model has been evaluated using a dataset covering 15 anomaly circumstances, including normal system behavior. The circumstances existing addressed various incidents from hardware failure to critical water infrastructure damage. The comprehensive results have indicated that the MARWIIoT model is superlative in compared existing machine learning models because it has the highest performance based on different evaluation metrics such as accuracy, precision, recall, and F1-Score.
更多查看译文
关键词
critical infrastructure, cyber-physical systems, industrial control system, machine learning
AI 理解论文
溯源树
样例
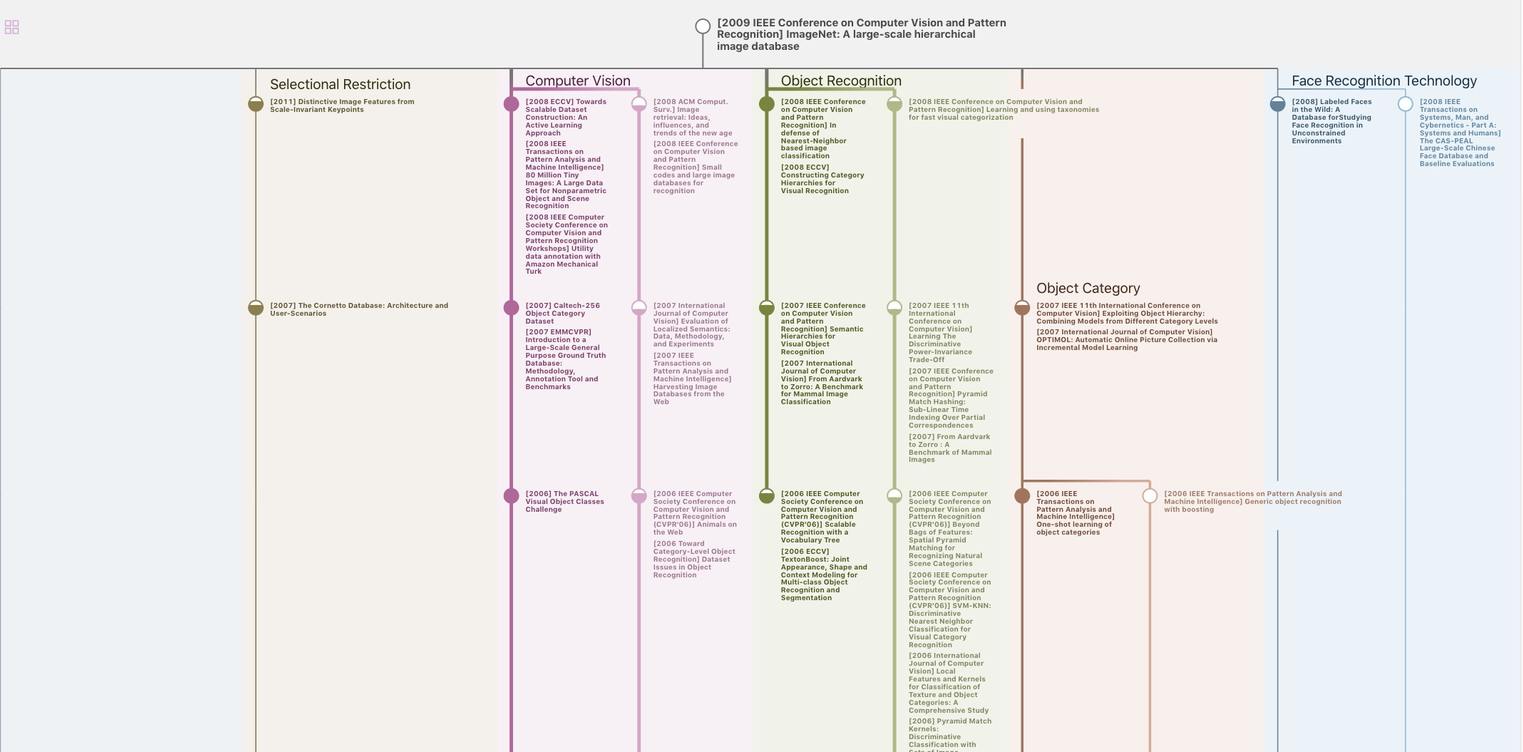
生成溯源树,研究论文发展脉络
Chat Paper
正在生成论文摘要