Microbiome Data Analysis By Symmetric Non-Negative Matrix Factorization With Local And Global Regularization
FRONTIERS IN MOLECULAR BIOSCIENCES(2021)
摘要
A network is an efficient tool to organize complicated data. The Laplacian graph has attracted more and more attention for its good properties and has been applied to many tasks including clustering, feature selection, and so on. Recently, studies have indicated that though the Laplacian graph can capture the global information of data, it lacks the power to capture fine-grained structure inherent in network. In contrast, a Vicus matrix can make full use of local topological information from the data. Given this consideration, in this paper we simultaneously introduce Laplacian and Vicus graphs into a symmetric non-negative matrix factorization framework (LVSNMF) to seek and exploit the global and local structure patterns that inherent in the original data. Extensive experiments are conducted on three real datasets (cancer, cell populations, and microbiome data). The experimental results show the proposed LVSNMF algorithm significantly outperforms other competing algorithms, suggesting its potential in biological data analysis.
更多查看译文
关键词
matrix factorization, Laplacian regularization, Vicus graph, microbiome, local structure
AI 理解论文
溯源树
样例
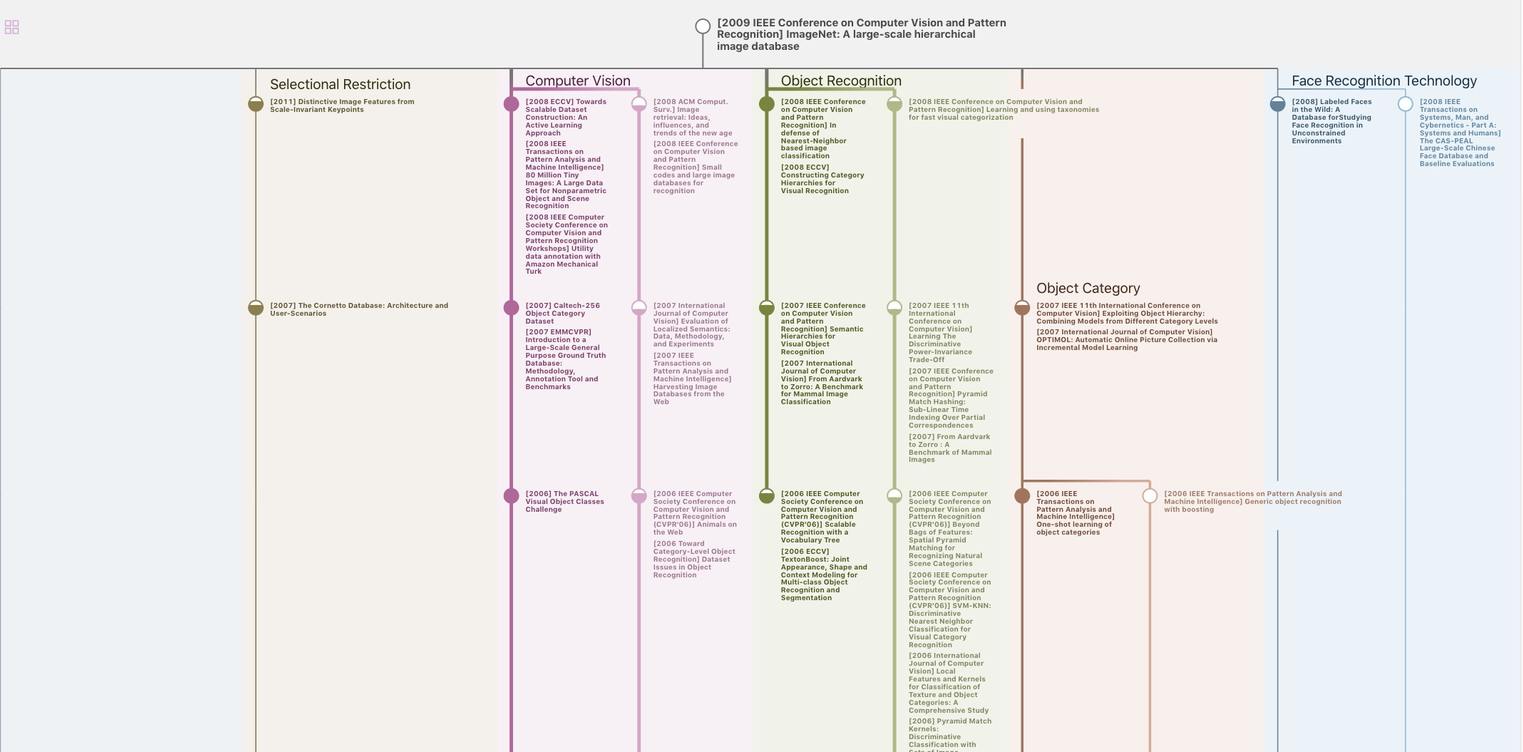
生成溯源树,研究论文发展脉络
Chat Paper
正在生成论文摘要