Frequent Pattern Mining in Continuous-Time Temporal Networks
IEEE TRANSACTIONS ON PATTERN ANALYSIS AND MACHINE INTELLIGENCE(2024)
摘要
Networks are used as highly expressive tools in different disciplines. In recent years, the analysis and mining of temporal networks have attracted substantial attention. Frequent pattern mining is considered an essential task in the network science literature. In addition to the numerous applications, the investigation of frequent pattern mining in networks directly impacts other analytical approaches, such as clustering, quasi-clique and clique mining, and link prediction. In nearly all the algorithms proposed for frequent pattern mining in temporal networks, the networks are represented as sequences of static networks. Then, the inter- or intra-network patterns are mined. This type of representation imposes a computation-expressiveness trade-off to the mining problem. In this paper, we propose a novel representation that can preserve the temporal aspects of the network losslessly. Then, we introduce the concept of constrained interval graphs ($CIG$CIGs). Next, we develop a series of algorithms for mining the complete set of frequent temporal patterns in a temporal network data set. We also consider four different definitions of isomorphism for accommodating minor variations in temporal data of networks. Implementing the algorithm for three real-world data sets proves the practicality of the proposed approach and its capability to discover unknown patterns in various settings.
更多查看译文
关键词
Continuous-time networks,frequent subgraphs,pattern mining,temporal networks
AI 理解论文
溯源树
样例
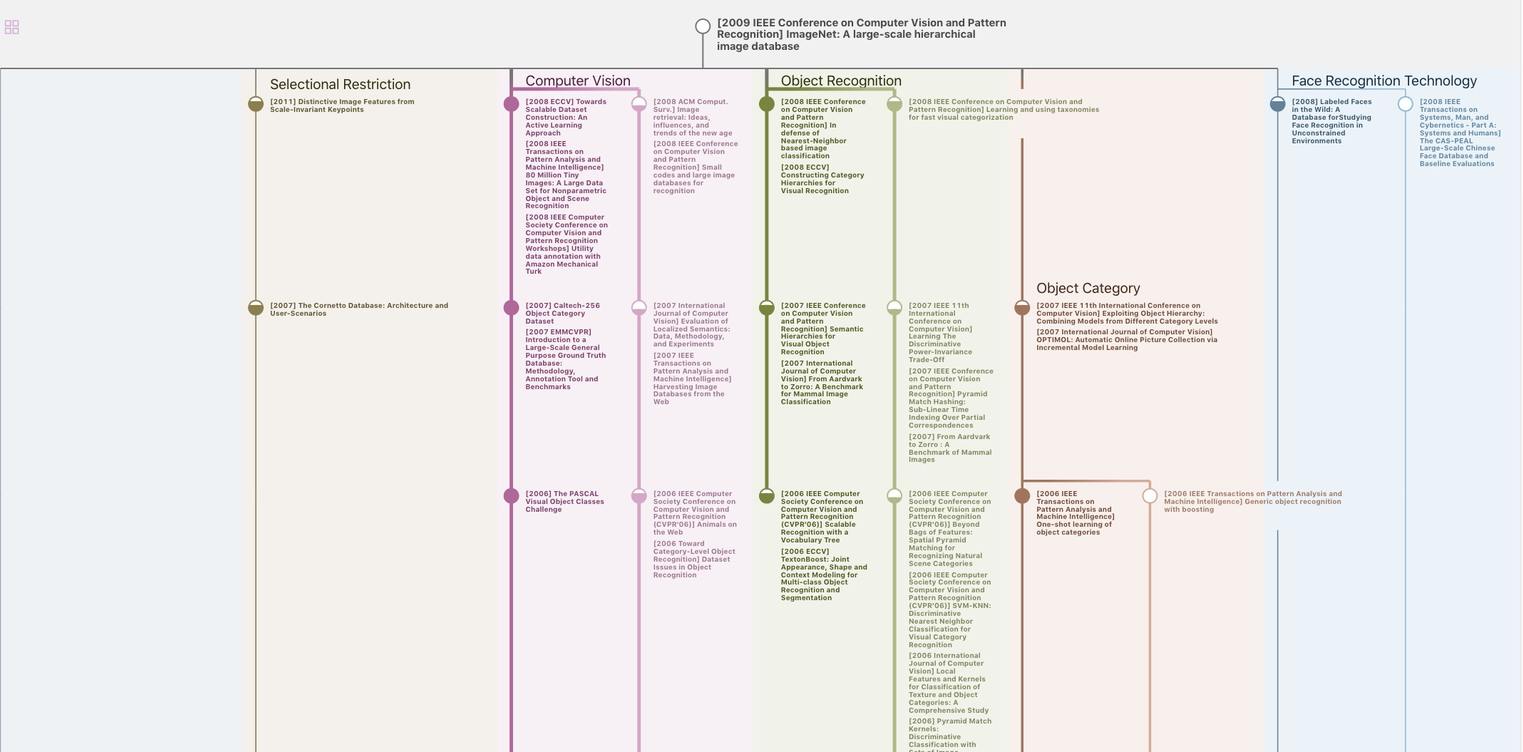
生成溯源树,研究论文发展脉络
Chat Paper
正在生成论文摘要