Deep Multi-Agent Reinforcement Learning for Highway On-Ramp Merging in Mixed Traffic
arXiv (Cornell University)(2023)
摘要
On-ramp merging is a challenging task for autonomous vehicles (AVs), especially in mixed traffic where AVs coexist with human-driven vehicles (HDVs). In this paper, we formulate the mixed-traffic highway on-ramp merging problem as a multi-agent reinforcement learning (MARL) problem, where the AVs (on both merge lane and through lane) collaboratively learn a policy to adapt to HDVs to maximize the traffic throughput. We develop an efficient and scalable MARL framework that can be used in dynamic traffic where the communication topology could be time-varying. Parameter sharing and local rewards are exploited to foster inter-agent cooperation while achieving great scalability. An action masking scheme is employed to improve learning efficiency by filtering out invalid/unsafe actions at each step. In addition, a novel priority-based safety supervisor is developed to significantly reduce collision rate and greatly expedite the training process. A gym-like simulation environment is developed and open-sourced with three different levels of traffic densities. We exploit curriculum learning to efficiently learn harder tasks from trained models under simpler settings. Comprehensive experimental results show the proposed MARL framework consistently outperforms several state-of-the-art benchmarks.
更多查看译文
关键词
Multi-agent deep reinforcement learning, connected autonomous vehicles, safety enhancement, on-ramp merging
AI 理解论文
溯源树
样例
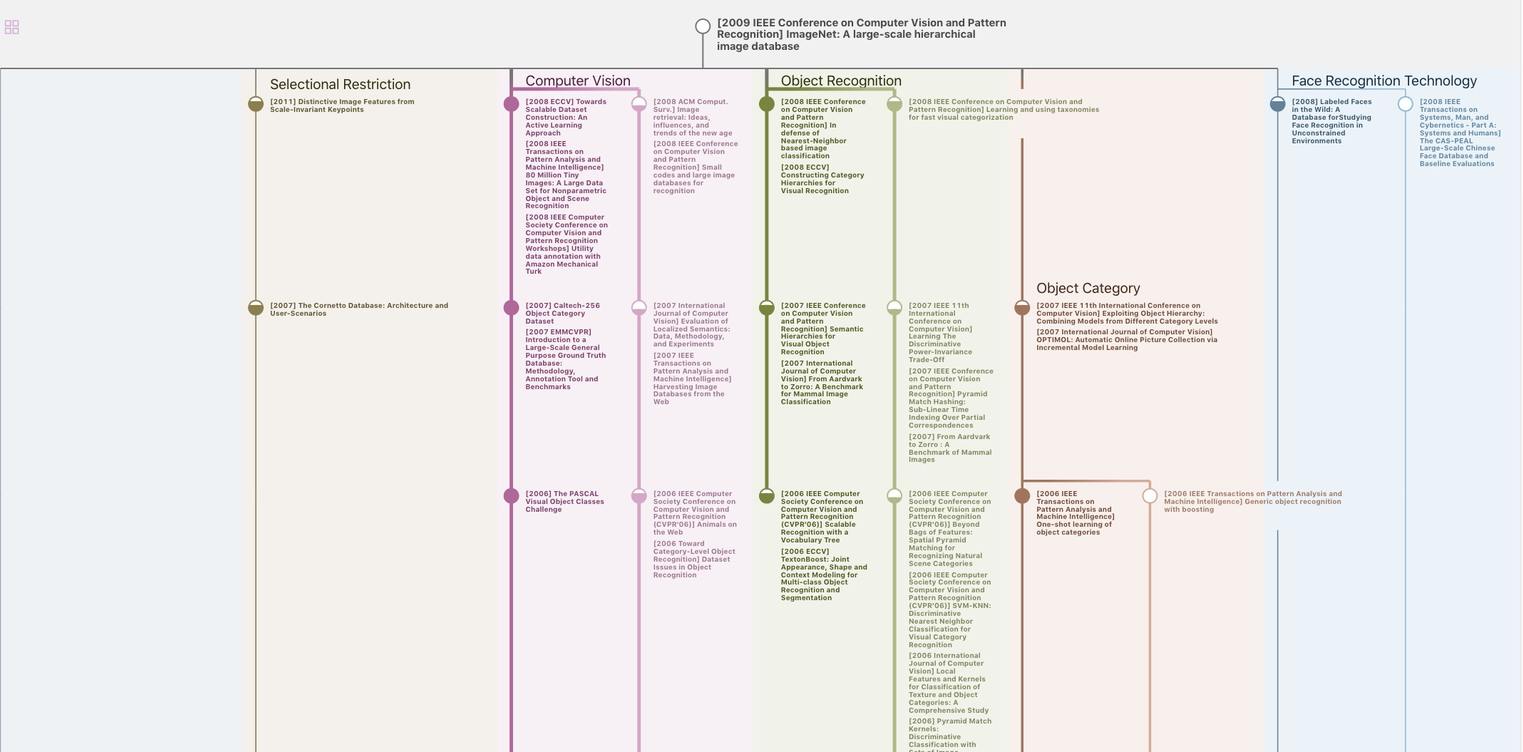
生成溯源树,研究论文发展脉络
Chat Paper
正在生成论文摘要