The Image Classification Method With Cnn-Xgboost Model Based On Adaptive Particle Swarm Optimization
INFORMATION(2021)
摘要
CNN is particularly effective in extracting spatial features. However, the single-layer classifier constructed by activation function in CNN is easily interfered by image noise, resulting in reduced classification accuracy. To solve the problem, the advanced ensemble model XGBoost is used to overcome the deficiency of a single classifier to classify image features. To further distinguish the extracted image features, a CNN-XGBoost image classification model optimized by APSO is proposed, where APSO optimizes the hyper-parameters on the overall architecture to promote the fusion of the two-stage model. The model is mainly composed of two parts: feature extractor CNN, which is used to automatically extract spatial features from images; feature classifier XGBoost is applied to classify features extracted after convolution. In the process of parameter optimization, to overcome the shortcoming that traditional PSO algorithm easily falls into a local optimal, the improved APSO guide the particles to search for optimization in space by two different strategies, which improves the diversity of particle population and prevents the algorithm from becoming trapped in local optima. The results on the image set show that the proposed model gets better results in image classification. Moreover, the APSO-XGBoost model performs well on the credit data, which indicates that the model has a good ability of credit scoring.
更多查看译文
关键词
figure classification, binary classification, hyper-parameters optimization, credit scoring
AI 理解论文
溯源树
样例
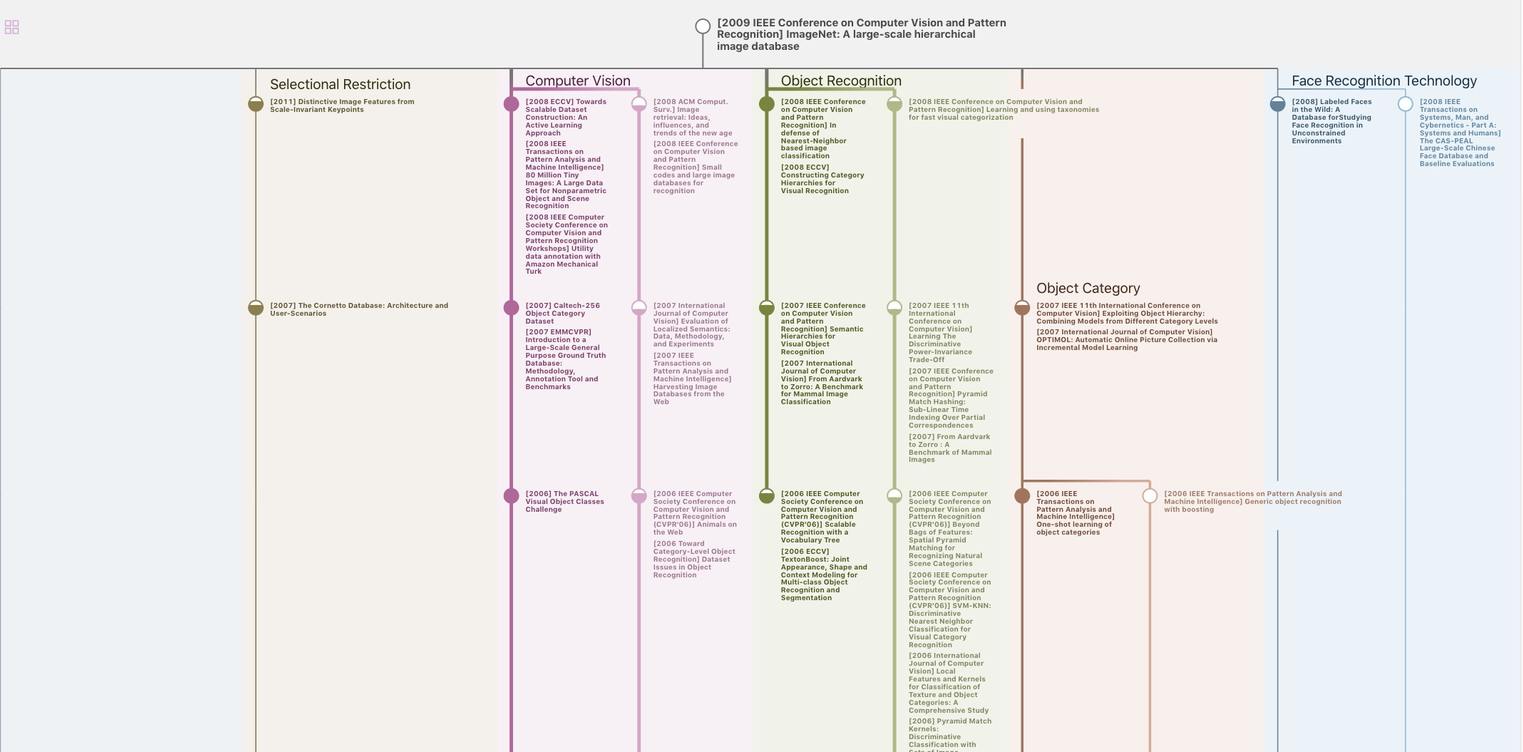
生成溯源树,研究论文发展脉络
Chat Paper
正在生成论文摘要