Looking at CTR Prediction Again: Is Attention All You Need?
Research and Development in Information Retrieval(2021)
摘要
ABSTRACTClick-through rate (CTR) prediction is a critical problem in web search, recommendation systems and online advertisement displaying. Learning good feature interactions is essential to reflect user's preferences to items. Many CTR prediction models based on deep learning have been proposed, but researchers usually only pay attention to whether state-of-the-art performance is achieved, and ignore whether the entire framework is reasonable. In this work, we use the discrete choice model in economics to redefine the CTR prediction problem, and propose a general neural network framework built on self-attention mechanism. It is found that most existing CTR prediction models align with our proposed general framework. We also examine the expressive power and model complexity of our proposed framework, along with potential extensions to some existing models. And finally we demonstrate and verify our insights through some experimental results on public datasets.
更多查看译文
关键词
click-through rate prediction, neural networks, self-attention mechanism, factorization machines, discrete choice model
AI 理解论文
溯源树
样例
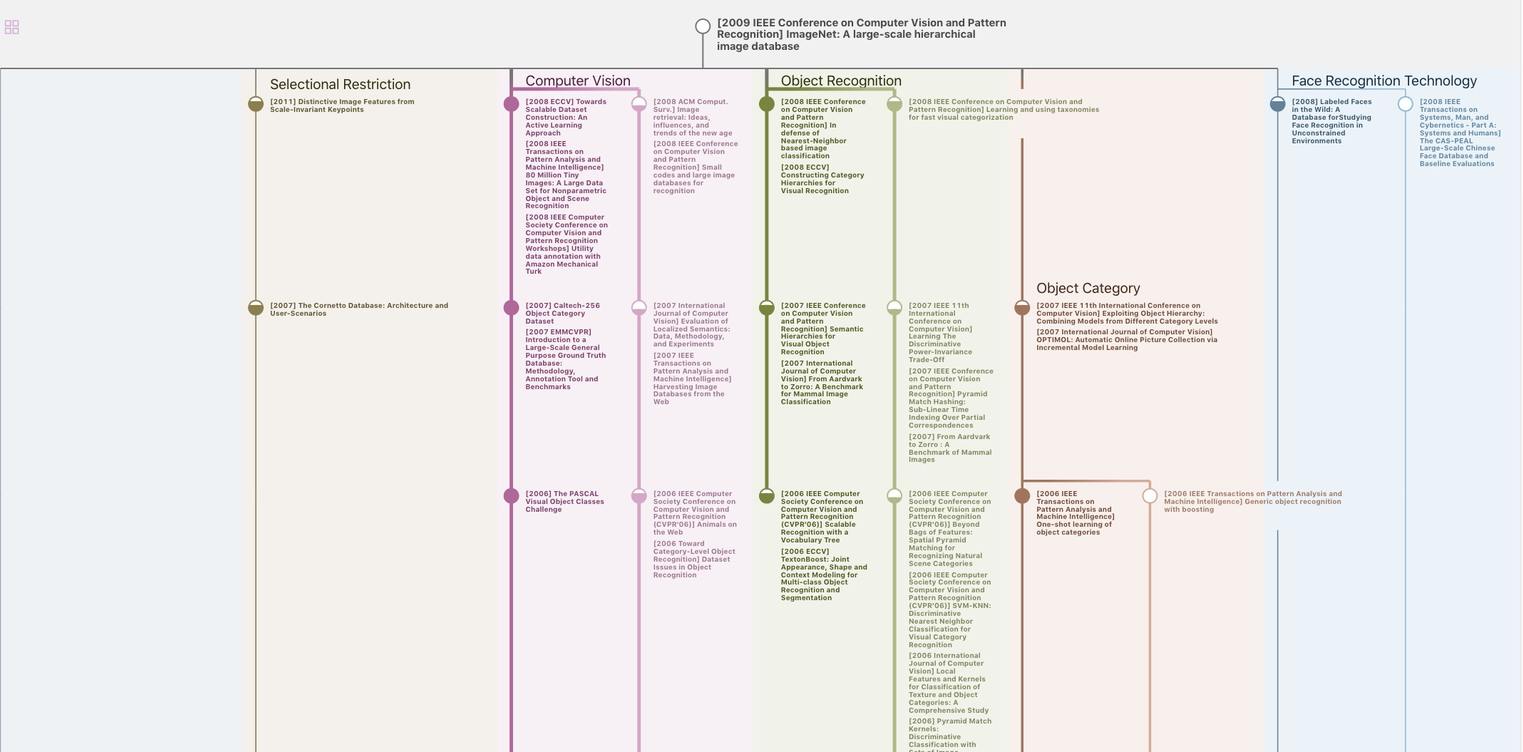
生成溯源树,研究论文发展脉络
Chat Paper
正在生成论文摘要