Return-based Scaling: Yet Another Normalisation Trick for Deep RL
arxiv(2021)
摘要
Scaling issues are mundane yet irritating for practitioners of reinforcement learning. Error scales vary across domains, tasks, and stages of learning; sometimes by many orders of magnitude. This can be detrimental to learning speed and stability, create interference between learning tasks, and necessitate substantial tuning. We revisit this topic for agents based on temporal-difference learning, sketch out some desiderata and investigate scenarios where simple fixes fall short. The mechanism we propose requires neither tuning, clipping, nor adaptation. We validate its effectiveness and robustness on the suite of Atari games. Our scaling method turns out to be particularly helpful at mitigating interference, when training a shared neural network on multiple targets that differ in reward scale or discounting.
更多查看译文
关键词
normalisation trick,scaling,deep,return-based
AI 理解论文
溯源树
样例
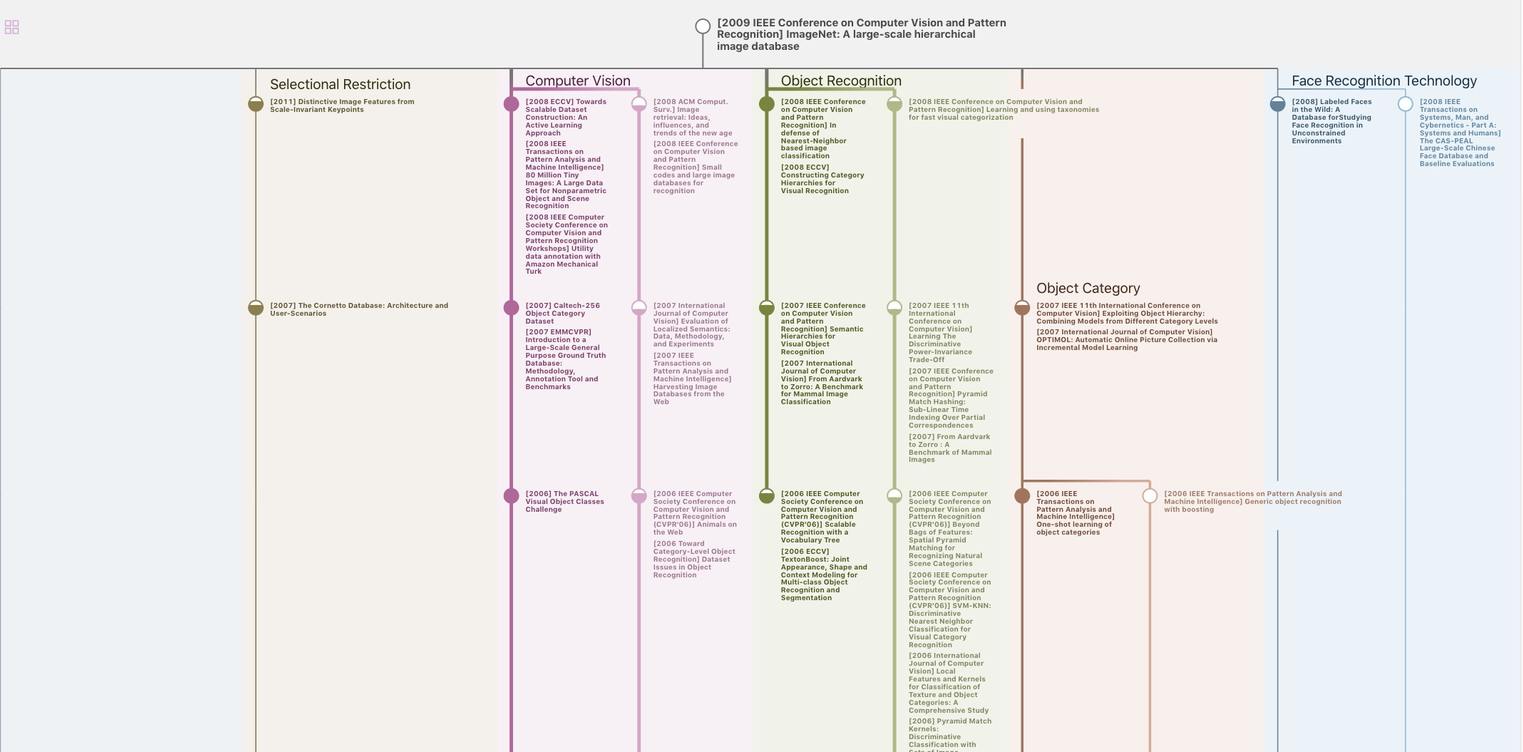
生成溯源树,研究论文发展脉络
Chat Paper
正在生成论文摘要