Hermitian Symmetric Spaces for Graph Embeddings
arxiv(2021)
摘要
Learning faithful graph representations as sets of vertex embeddings has become a fundamental intermediary step in a wide range of machine learning applications. The quality of the embeddings is usually determined by how well the geometry of the target space matches the structure of the data. In this work we learn continuous representations of graphs in spaces of symmetric matrices over C. These spaces offer a rich geometry that simultaneously admits hyperbolic and Euclidean subspaces, and are amenable to analysis and explicit computations. We implement an efficient method to learn embeddings and compute distances, and develop the tools to operate with such spaces. The proposed models are able to automatically adapt to very dissimilar arrangements without any apriori estimates of graph features. On various datasets with very diverse structural properties and reconstruction measures our model ties the results of competitive baselines for geometrically pure graphs and outperforms them for graphs with mixed geometric features, showcasing the versatility of our approach.
更多查看译文
关键词
hermitian symmetric spaces,graph embeddings
AI 理解论文
溯源树
样例
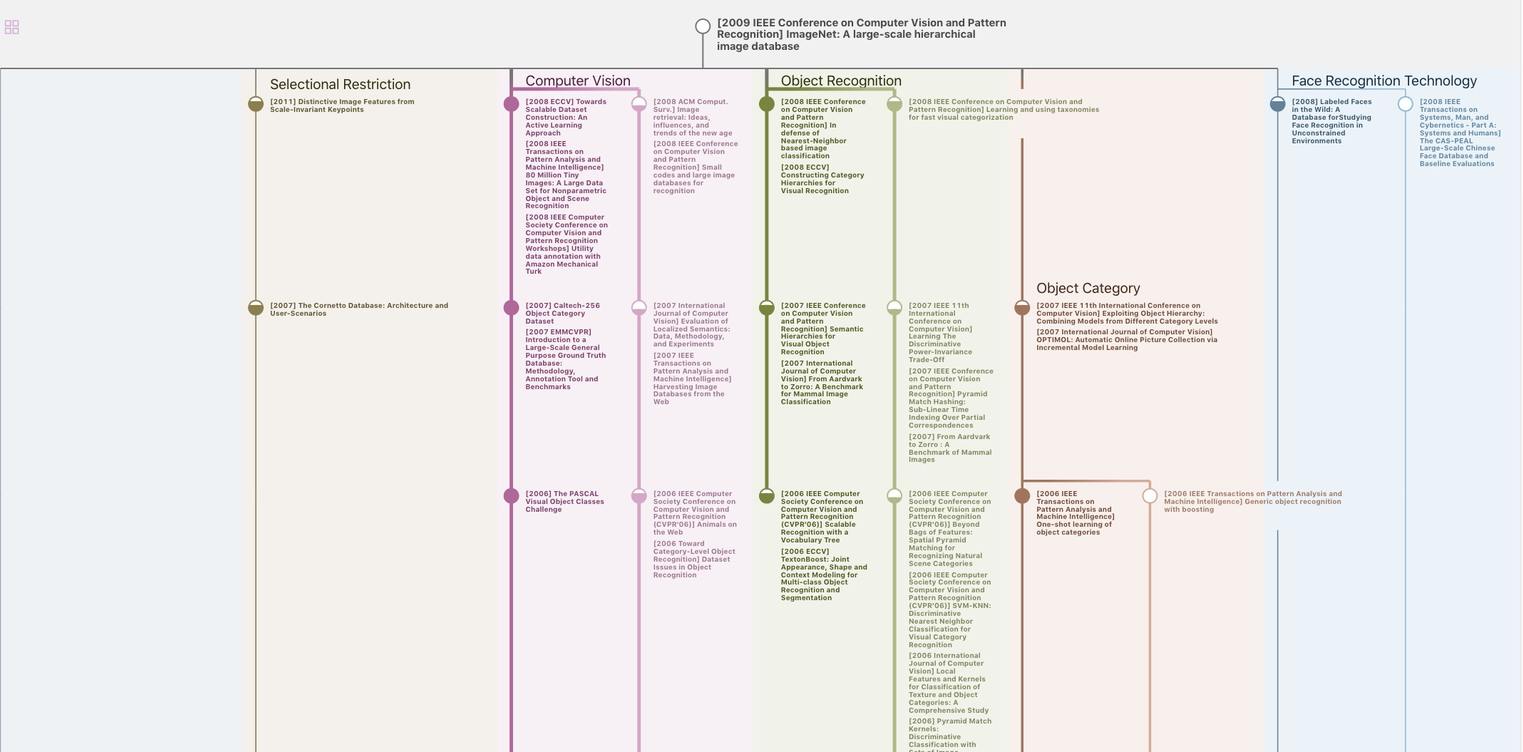
生成溯源树,研究论文发展脉络
Chat Paper
正在生成论文摘要