Accountable Error Characterization
arxiv(2021)
摘要
Customers of machine learning systems demand accountability from the companies employing these algorithms for various prediction tasks. Accountability requires understanding of system limit and condition of erroneous predictions, as customers are often interested in understanding the incorrect predictions, and model developers are absorbed in finding methods that can be used to get incremental improvements to an existing system. Therefore, we propose an accountable error characterization method, AEC, to understand when and where errors occur within the existing black-box models. AEC, as constructed with human-understandable linguistic features, allows the model developers to automatically identify the main sources of errors for a given classification system. It can also be used to sample for the set of most informative input points for a next round of training. We perform error detection for a sentiment analysis task using AEC as a case study. Our results on the sample sentiment task show that AEC is able to characterize erroneous predictions into human understandable categories and also achieves promising results on selecting erroneous samples when compared with the uncertainty-based sampling.
更多查看译文
关键词
characterization
AI 理解论文
溯源树
样例
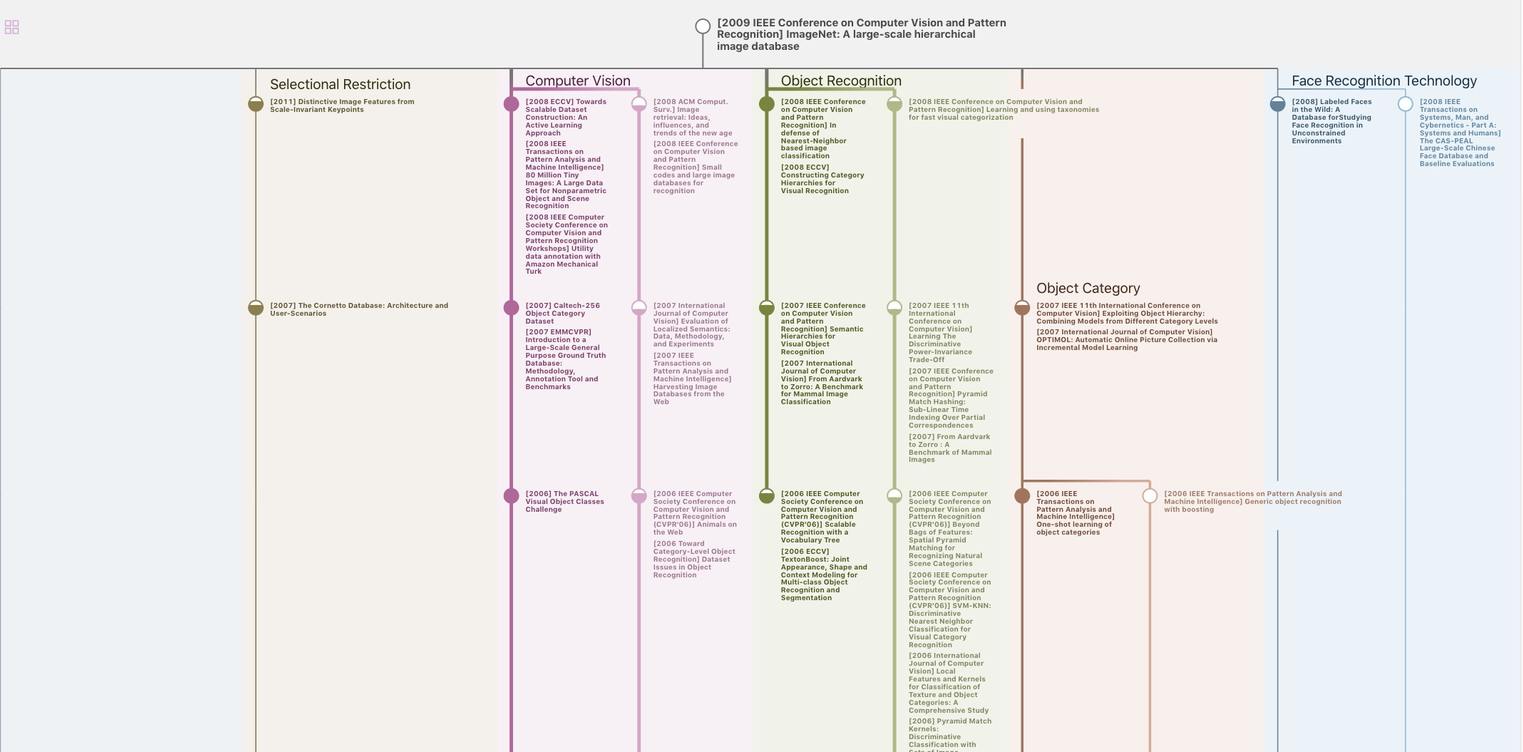
生成溯源树,研究论文发展脉络
Chat Paper
正在生成论文摘要