Rapid enthalpy prediction of transition states using molecular graph convolutional network
Aiche Journal(2023)
摘要
The rapid development of machine learning has provided low-cost and high-throughput molecular energy prediction methods; however, the direct energy prediction of transition states (TSs) in radical reactions has received little attention, which can facilitate the rapid construction of potential energy surface (PES) and chemical reactivity inference. Here, we present a generic descriptor of TSs, molecules and radicals to realize the simultaneous prediction of their enthalpy of formation through the augmented molecular graph convolutional network (MGCN). It is proved that the network can rapidly (within milliseconds per prediction) and accurately predict the enthalpy of various types of samples, of which the mean absolute error (MAE) of the held-out test set is 1.44 kcal/mol (>50% samples less than 1.0 kcal/mol) with high R-2 (0.925-0.998) among all categories. The PES constructed from predictions is close to the calculated results by density functional theory (DFT) and conforms to the trend of chemical reactivity.
更多查看译文
关键词
computational chemistry,enthalpy of formation,machine learning,molecular graph convolutional network,transition states
AI 理解论文
溯源树
样例
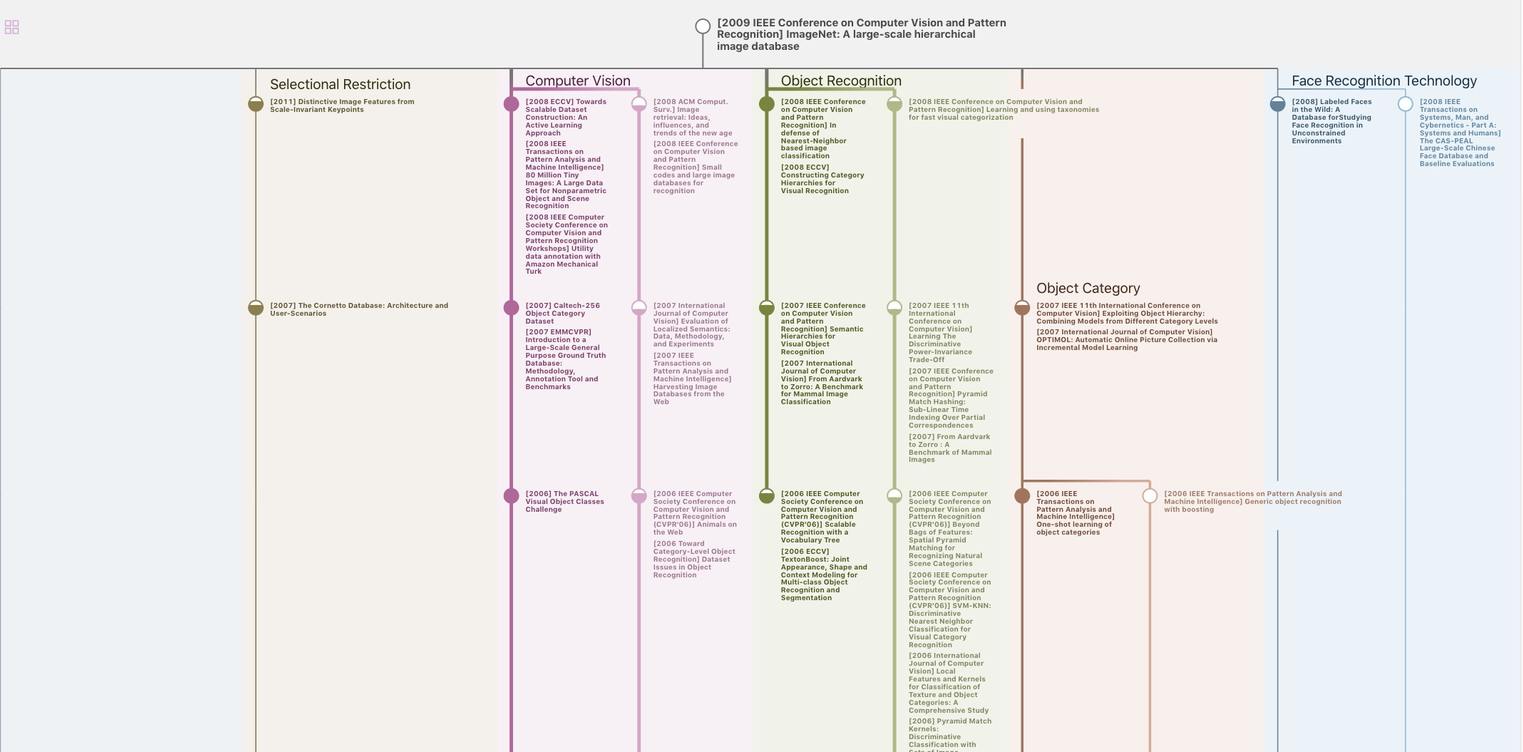
生成溯源树,研究论文发展脉络
Chat Paper
正在生成论文摘要