Finite-Memory Strategies in POMDPs with Long-Run Average Objectives
MATHEMATICS OF OPERATIONS RESEARCH(2022)
摘要
Partially observable Markov decision processes (POMDPs) are standard models for dynamic systems with probabilistic and nondeterministic behaviour in uncertain environments. We prove that in POMDPs with long-run average objective, the decision maker has approximately optimal strategies with finite memory. This implies notably that approximating the long-run value is recursively enumerable, as well as a weak continuity property of the value with respect to the transition function.
更多查看译文
关键词
finite state, Markov, dynamic programming, computational complexity, analysis of algorithms
AI 理解论文
溯源树
样例
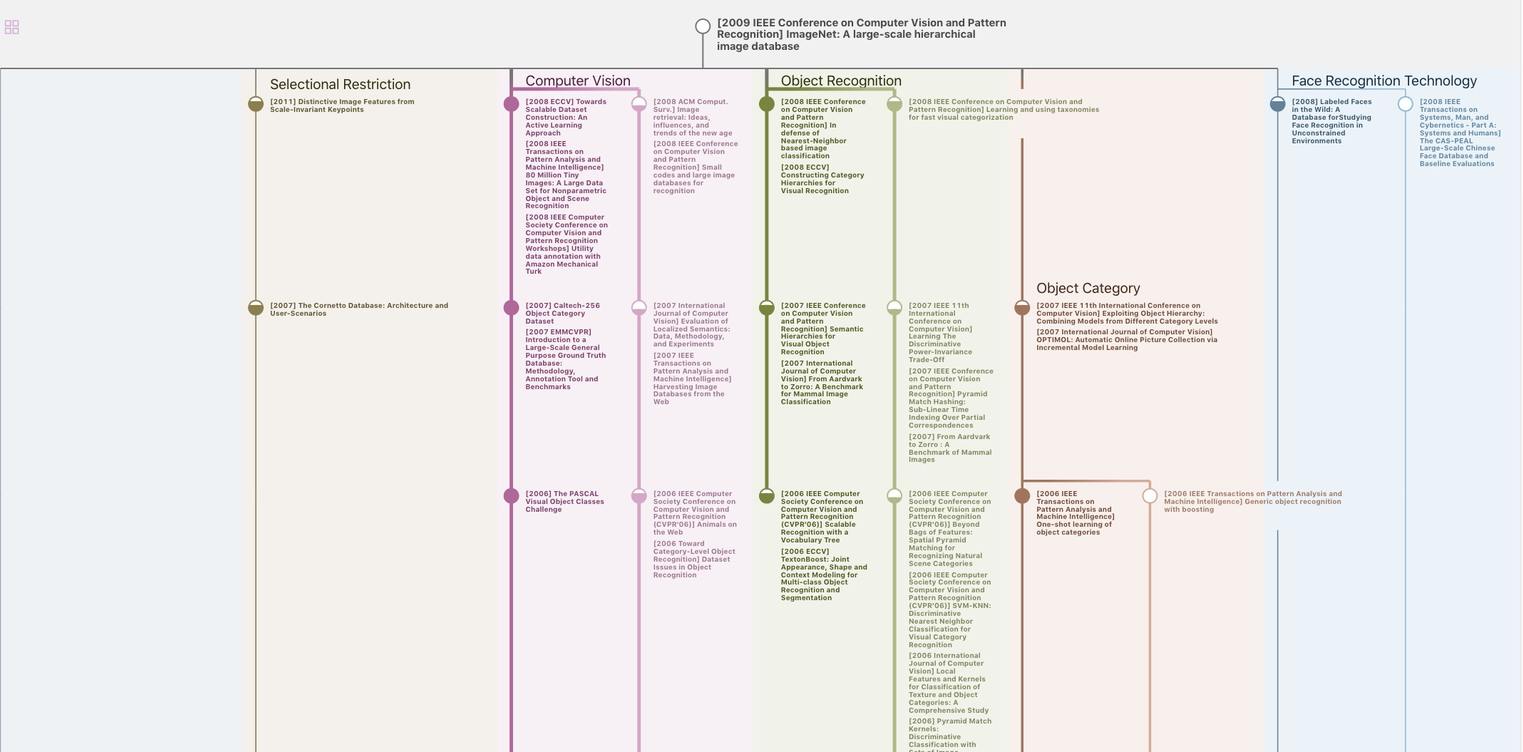
生成溯源树,研究论文发展脉络
Chat Paper
正在生成论文摘要