Finding Proper Time Intervals For Dynamic Network Extraction
JOURNAL OF STATISTICAL MECHANICS-THEORY AND EXPERIMENT(2021)
摘要
Extracting a proper dynamic network for modeling a time-dependent complex system is an important issue. Building a correct model is related to finding out critical time points where a system exhibits considerable change. In this work, we propose to measure network similarity to detect proper time intervals. We develop three similarity metrics, node, link, and neighborhood similarities, for any consecutive snapshots of a dynamic network. Rather than a label or a user-defined threshold, we use statistically expected values of proposed similarities under a null-model to state whether the system changes critically. We experimented on two different data sets with different temporal dynamics: the Wi-Fi access points logs of a university campus and Enron emails. Results show that, first, proposed similarities reflect similar signal trends with network topological properties with less noisy signals, and their scores are scale invariant. Second, proposed similarities generate better signals than adjacency correlation with optimal noise and diversity. Third, using statistically expected values allows us to find different time intervals for a system, leading to the extraction of non-redundant snapshots for dynamic network modeling.
更多查看译文
关键词
network dynamics, communication, supply and information networks, information technology networks, systemic stability
AI 理解论文
溯源树
样例
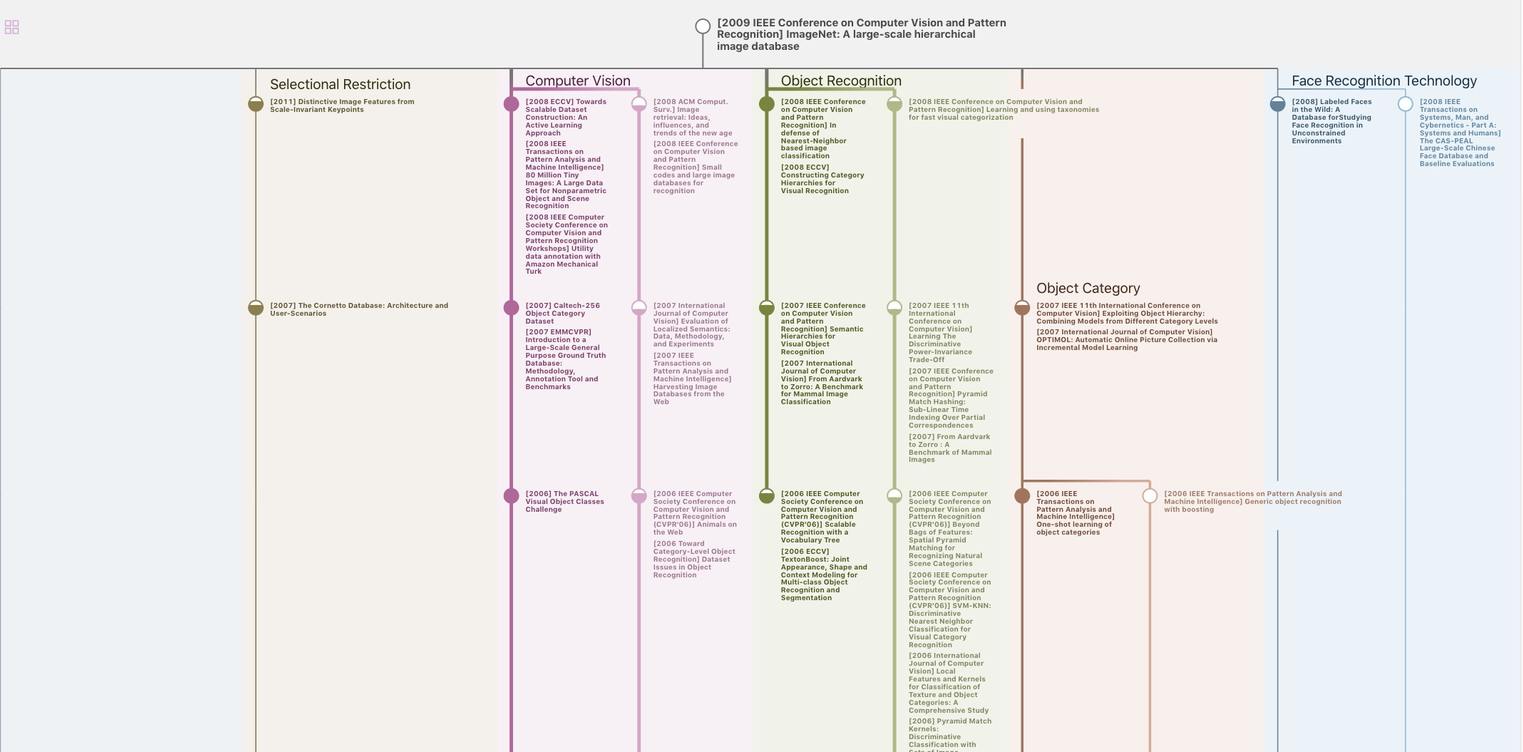
生成溯源树,研究论文发展脉络
Chat Paper
正在生成论文摘要