Deep Learning-Based Prediction Method On Performance Change Of Air Source Heat Pump System Under Frosting Conditions
ENERGY(2021)
摘要
Since frost on an outdoor heat exchanger in winter reduces the performance of an air source heat pump (ASHP), a defrosting process is necessary to restore the degraded performance. Therefore, frosting and defrosting are crucial challenges. For a more efficient defrosting process, many researchers have conducted studies on demand-based defrosting control so far. Recently, various researches on frost growth prediction using neural networks have been conducted. Here, we propose a novel method to quantitatively predict changes in the performance (heating capacity, power consumption, and COP) of ASHPs due to frost growth using a single model based on deep learning. Based on prediction results, this method can be utilized to optimize the defrosting start control strategy. With multiple outputs regression models, we can predict three performance parameters simultaneously. They are models trained with only the initially installed sensors without additional sensors. Besides, we compared the prediction accuracy differences depending on three deep learning structures, such as a fully-connected deep neural network (FCDNN), convolutional neural network (CNN), and long short-term memory (LSTM). Summarizing the results, the optimal FCDNN-based model achieved a root-mean-square (RMS) error of 2.8% for the prediction of heating capacity, 2.4% for power consumption, and 3.4% for COP of ASHPs. (c) 2021 Published by Elsevier Ltd.
更多查看译文
关键词
Air source heat pump, Frost growth, Defrosting start control, Deep learning, Quantitative prediction, Multiple outputs regression
AI 理解论文
溯源树
样例
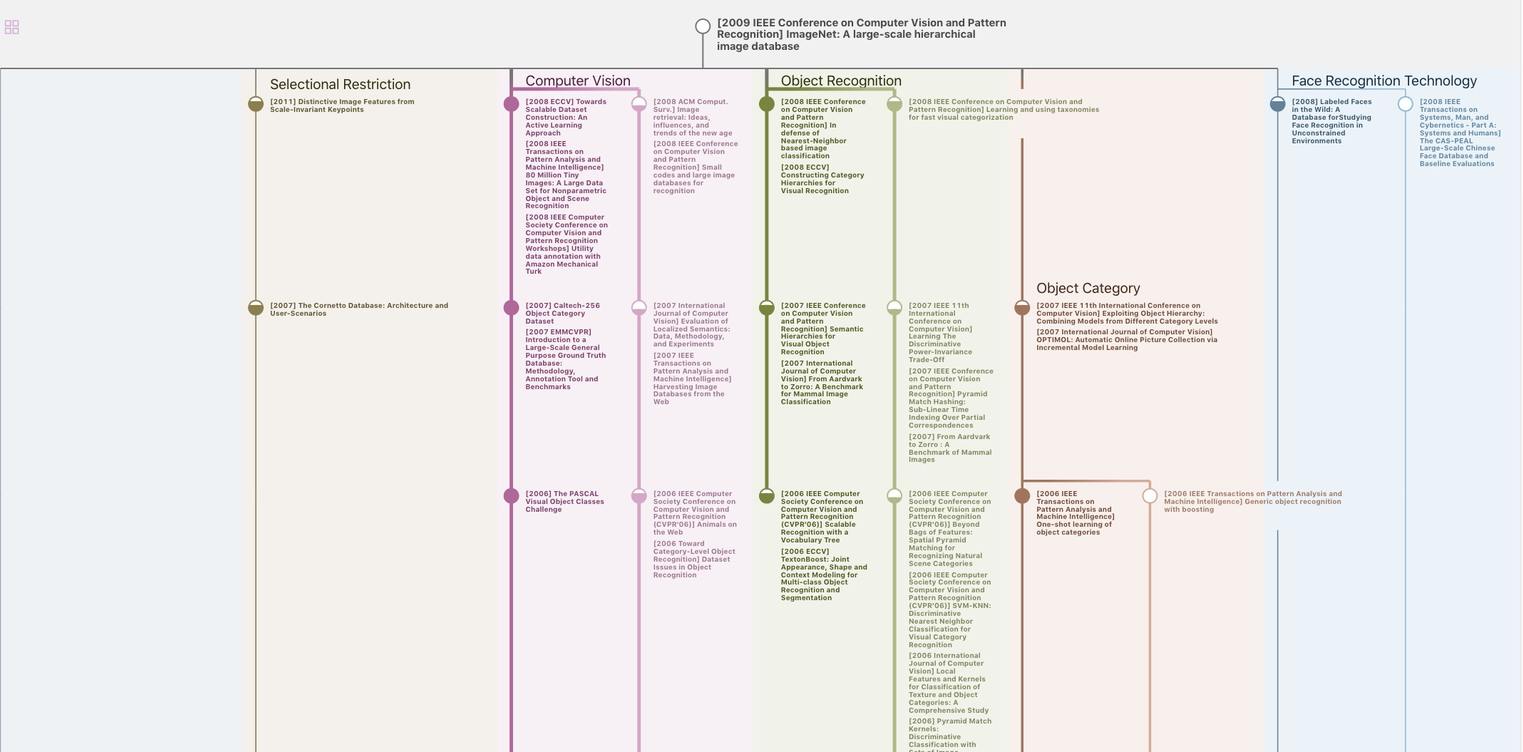
生成溯源树,研究论文发展脉络
Chat Paper
正在生成论文摘要