Comparative performance of linear regression, polynomial regression and generalized additive model for canopy cover estimation in the dry deciduous forest of West Bengal
Remote Sensing Applications: Society and Environment(2021)
摘要
Canopy cover is an important biophysical indicator of forested habitats, but quantifying and monitoring with the accurate and cost-efficient method is always difficult in fragmented canopy cover areas. Therefore, this study was designed to explore the comparative performance of linear regression, polynomial regression and generalized additive model (GAM), and assess the important predictors for canopy cover estimation in the dry deciduous forest of West Bengal. We used the Sentinel-2 based individual bands and spectral indices as predictor variables from the different composition of imagery in the growing season (June–September) between 2018 and 2020. Canopy cover of 667 plots were measured using collect earth online (CEO) from high resolution imagery. Univariate and multiple models were fitted and assessed by 10 fold cross-validation. Root mean square error (RMSE) and the coefficient of determination (R2) was used for the evaluation of model performance. The result showed that univariate models using GAM were fitted better followed polynomial regression and linear regression model. SWIR bands and SWIR bands based indices were fitted well than other groups of variables. Individual bands based multiple prediction models performed better followed by NIR, SWIR, atmospheric and red edge indices based multiple prediction models for multiple linear regression, multiple polynomial regression and multiple GAM. Multiple GAM performed better (RMSE = 0.163, R2 = 0.777) than multiple polynomial regression (RMSE = 0.167, R2 = 0.772) and multiple linear regression model (RMSE = 0.182, R2 = 0.721). Although, the difference of performance between multiple polynomial regression model and multiple GAM was negligible. But in respect to prediction range, and overestimation and underestimation multiple GAM was superior to multiple polynomial regression model. The study demonstrates that the potential utility of GAM for canopy cover estimation and GAM can be superior to linear and polynomial regression model using suitable predictor variables.
更多查看译文
关键词
Sentinel-2,Google earth engine,Multiple prediction model,Generalized additive model,Canopy cover,Vegetation indices
AI 理解论文
溯源树
样例
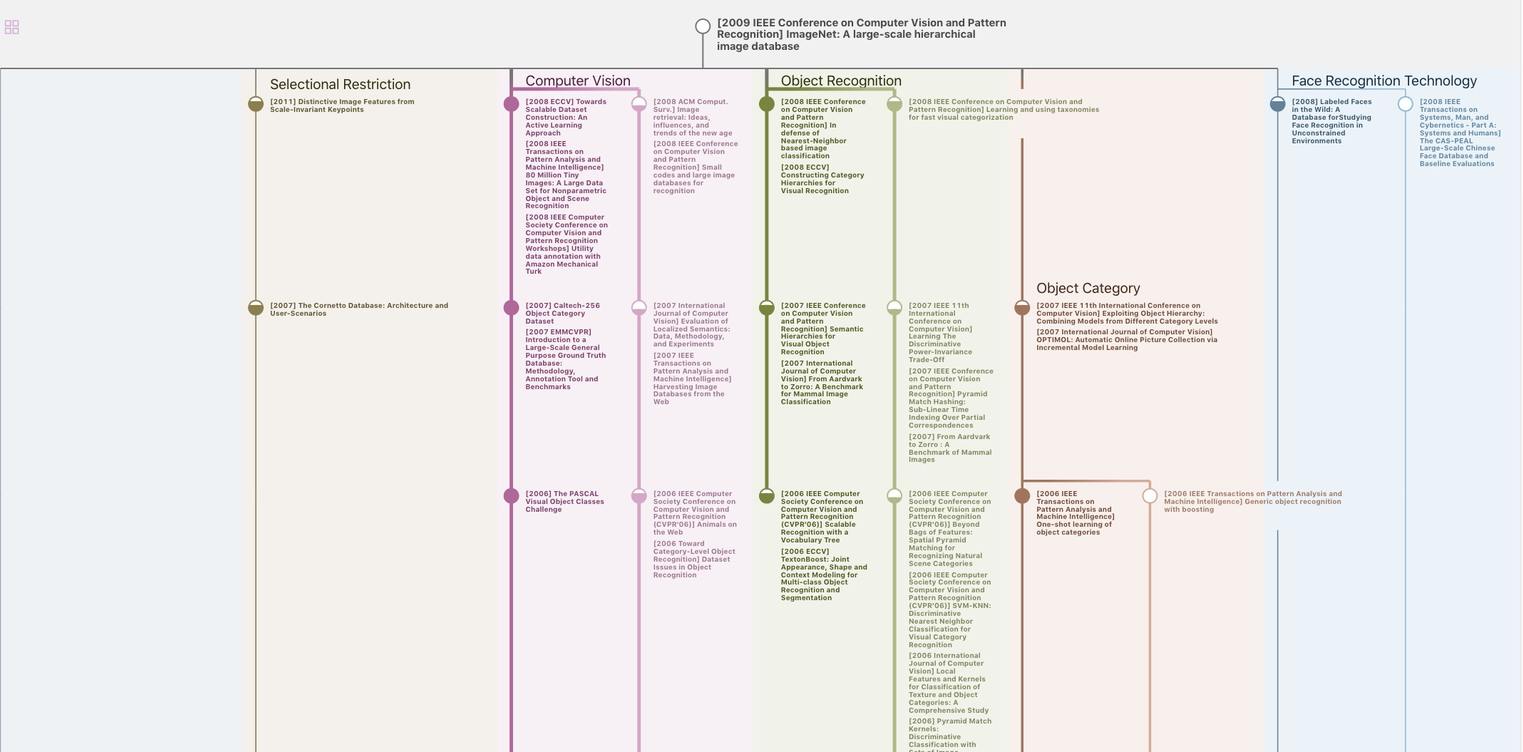
生成溯源树,研究论文发展脉络
Chat Paper
正在生成论文摘要