Deep learning approach to coal and gas outburst recognition employing modified AE and EMR signal from empirical mode decomposition and time-frequency analysis
Journal of Natural Gas Science and Engineering(2021)
摘要
Acoustic emission (AE) and electromagnetic radiation (EMR) monitoring technology have been widely used in coal and rock dynamic disaster monitoring. However, the prediction of coal and gas outburst using original signal directly may cause the false and missing alarms due to the failure to identify valuable potential information, which may lead to the decrease of prediction accuracy. This paper presents a new method to identify the precursor features of coal and gas outburst using two-dimensional Convolutional Neural Network (CNN). Empirical mode decomposition (EMD) was performed for AE or EMR signals, and the Intrinsic Mode Functions (IMFs) with Variance Contribution Rate (VCR) greater than 90% were merged into modified signal. Since the frequency of AE or EMR signal can change with different coal and rock fracture stages, one-dimensional modified signal was transformed into a two-dimensional time-frequency graph using the short-time Fourier transform (STFT). The time-frequency graph was input into CNN that recognizes the time-frequency characteristics of AE or EMR using SoftMax regression at the end of the network. Compared with the original signal, the precursor characteristics of modified AE or EMR signals can be better learned by CNN. The proposed method was applied to the Jinjia Coal Mine database which was identified as having outburst risk, and it was found that the recognition accuracy was better than other approaches. The proposed method achieves maximum recognition accuracy of 98.00% (AE), and 97.20% (EMR) in Jinjia Coal Mine database, respectively.
更多查看译文
关键词
Coal and gas outbursts,Empirical mode decomposition,Time-frequency characteristics,Convolution neural network,Feature recognition
AI 理解论文
溯源树
样例
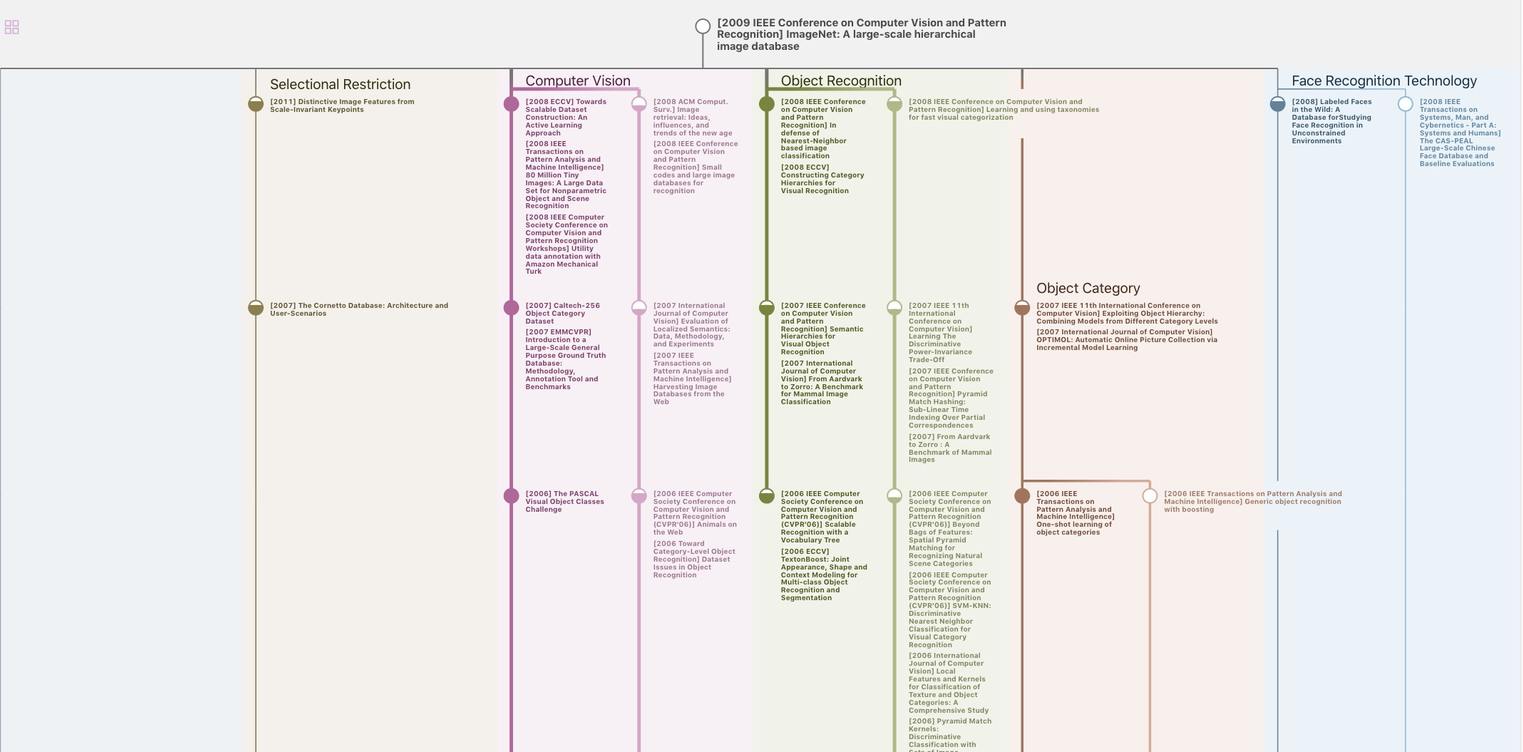
生成溯源树,研究论文发展脉络
Chat Paper
正在生成论文摘要