An Early Fault Diagnosis Method Of Common-Rail Injector Based On Improved Cycbd And Hierarchical Fluctuation Dispersion Entropy
DIGITAL SIGNAL PROCESSING(2021)
摘要
Early fault diagnosis of common rail injectors is essential to reduce diesel engine testing and maintenance costs. Therefore, this paper proposes a new common rail injector early fault diagnosis method, which combines the Maximum Second-order Cyclostationary Blind Deconvolution (CYCBD) optimized by the Seagull Optimization Algorithm (SOA) and Hierarchical Fluctuation Dispersion Entropy (HFDE). First, we use SOA adaptively to seek the optimal filter length of CYCBD and use the optimal CYCBD to filter the fuel pressure signal of the high-pressure fuel pipe. Then, in order to make up for the shortcomings of Multi-scale Fluctuation Dispersion Entropy (MFDE) ignoring high-frequency component information, this paper proposes HFDE to extract the fault characteristics after filtering. Finally, we input the fault characteristics into Least Squares Support Vector Machines (LSSVM) for classification and recognition. Through the analysis of experimental data, the method proposed in this paper can effectively identify the early failure state of the common rail injector. Compared with the existing methods, the proposed method has a higher fault recognition rate. (C) 2021 Elsevier Inc. All rights reserved.
更多查看译文
关键词
Maximum second-order cyclostationarity blind deconvolution, Hierarchical fluctuation dispersion entropy, Seagull optimization algorithm, Early fault diagnosis, Common-rail injector
AI 理解论文
溯源树
样例
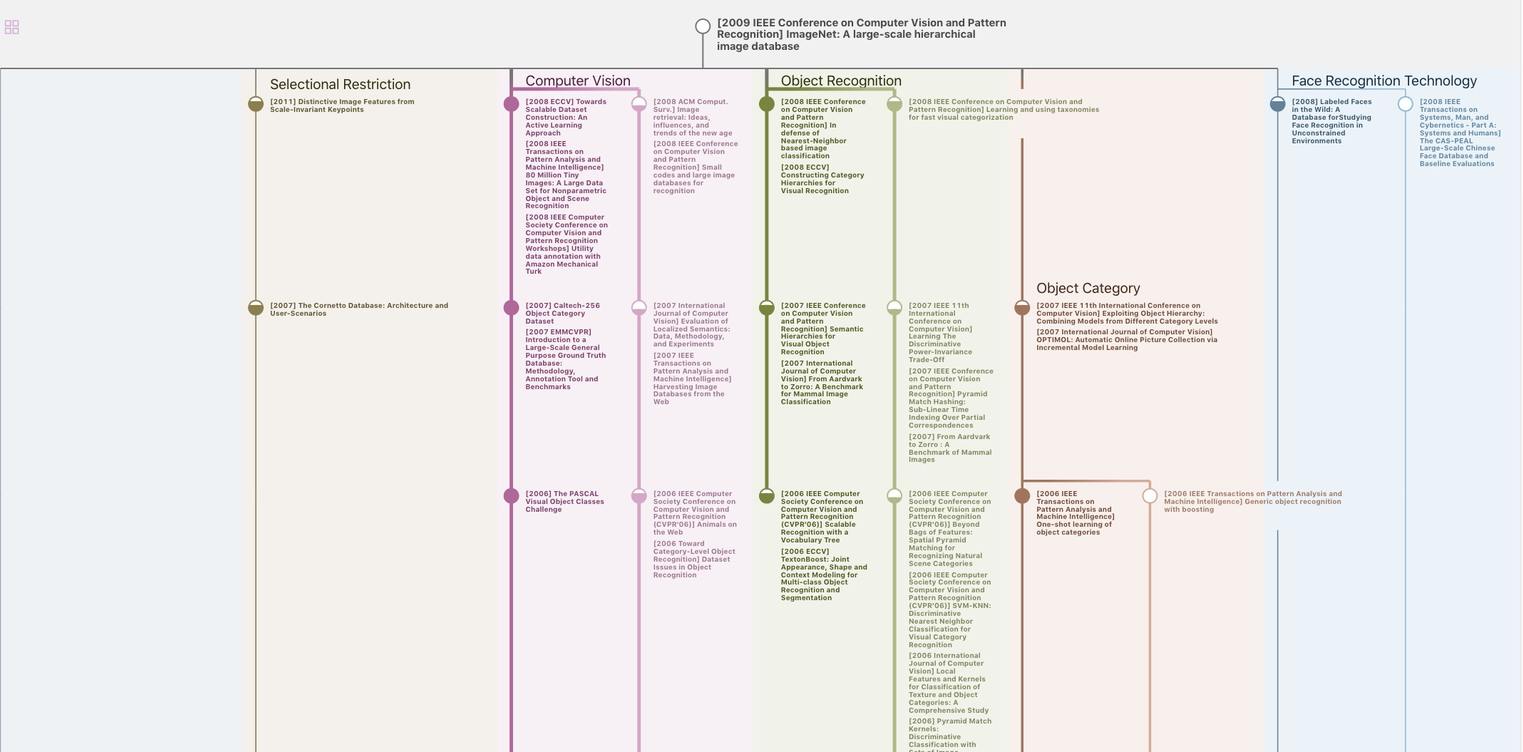
生成溯源树,研究论文发展脉络
Chat Paper
正在生成论文摘要