Detecting Spatiotemporal Extents Of Traffic Congestion: A Density-Based Moving Object Clustering Approach
INTERNATIONAL JOURNAL OF GEOGRAPHICAL INFORMATION SCIENCE(2021)
摘要
Traffic congestion detection poses challenges in spatiotemporal data mining and intelligent transportation research. Existing studies primarily detect traffic congestion based on the speed estimation of traffic flows. Such detection techniques may overlook the formation of traffic congestion in space and time. This research proposes a density-based approach to moving object clustering that extracts the spatiotemporal extents of traffic congestion in three steps. The first step applies a map-matching strategy to project original trajectory points in a planar space onto a road network space and segments the trajectories into consecutive time windows. In the second step, we statistically detect moving clusters with significantly high-density subject to network constrained clustering. The final third step determines moving clusters indicative of traffic congestion through the analysis of both vehicle speed and time spans. Comparative experiments on both simulated trajectories and the real-life taxi trajectories in Wuchang demonstrate that the proposed method outperforms other methods through quantitative evaluations using three indicators, i.e. the precision, recall and F1 value. The proposed approach can illustrate the spatiotemporal regularities of traffic congestion, which can inform dynamic route planning and network design optimization.
更多查看译文
关键词
Traffic congestion, road network space, density-based clustering, moving clusters
AI 理解论文
溯源树
样例
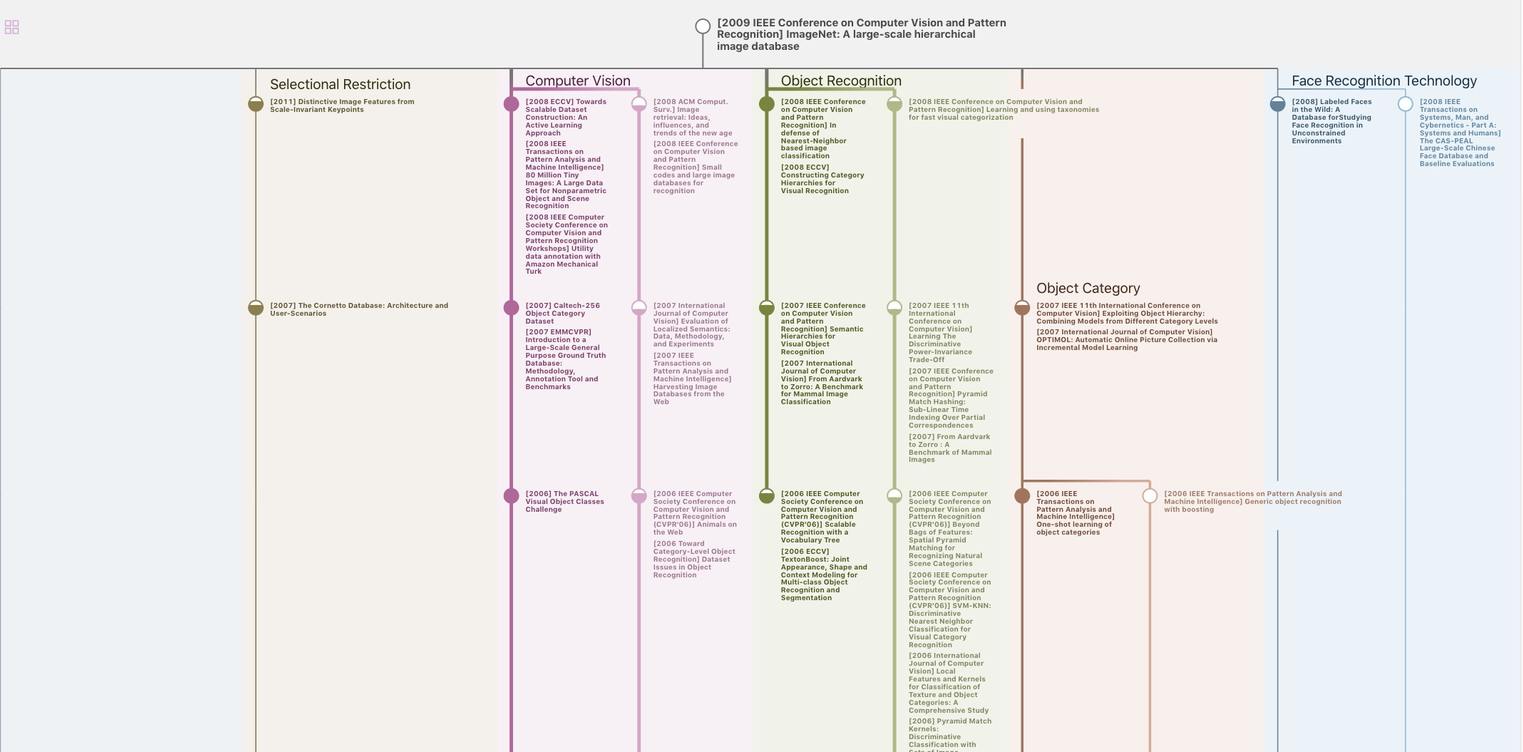
生成溯源树,研究论文发展脉络
Chat Paper
正在生成论文摘要