Integrating Gate And Attention Modules For High-Resolution Image Semantic Segmentation
IEEE JOURNAL OF SELECTED TOPICS IN APPLIED EARTH OBSERVATIONS AND REMOTE SENSING(2021)
摘要
Semantic segmentation of high-resolution (HR) remote sensing images achieved great progress by utilizing deep convolutional neural networks (DCNNs) in recent years. However, the decrease of resolution in the feature map of DCNNs brings about the loss of spatial information and thus leads to the blurring of object boundary and misclassification of small objects. In addition, the class imbalance and the high diversity of geographic objects in HR images exacerbate the performance. To deal with the above problems, we proposed an end-to-end DCNN network named GAMNet to balance the contradiction between global semantic information and local details. An integration of attention and gate module (GAM) is specially designed to simultaneously realize multiscale feature extraction and boundary recovery. The integration module can be inserted in an encoder-decoder network with skip connection. Meanwhile, a composite loss function is designed to achieve deep supervision of GAM by adding an auxiliary loss, which can help improve the effectiveness of the integration module. The performance of GAMNet is quantitatively evaluated on the ISPRS 2-D semantic labeling datasets and achieves state-of-the-art performance in comparison with other representative methods.
更多查看译文
关键词
Semantics, Image segmentation, Feature extraction, Decoding, Remote sensing, Spatial resolution, Logic gates, Attention module (AM), gate module (GM), high-resolution (HR) remote sensing imagery, semantic segmentation
AI 理解论文
溯源树
样例
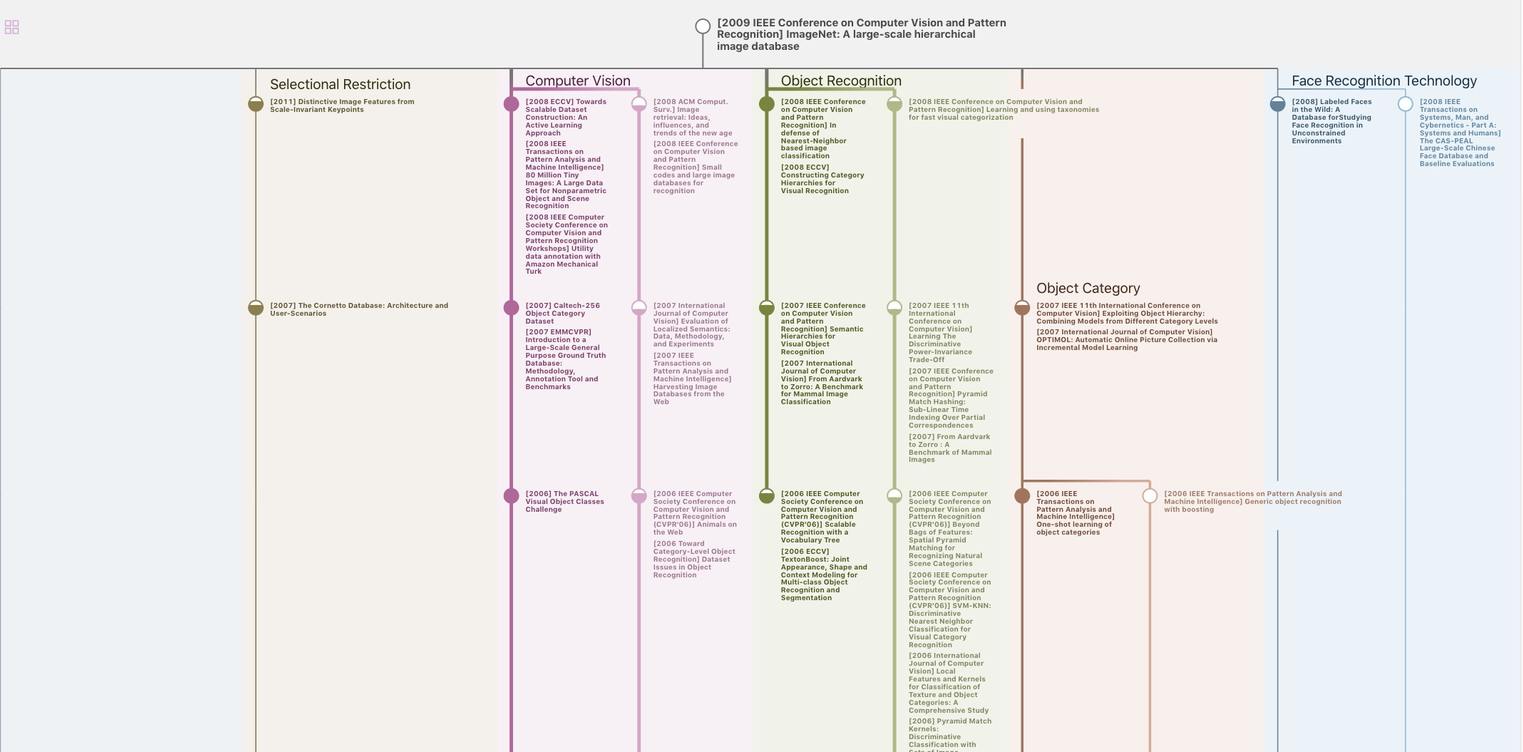
生成溯源树,研究论文发展脉络
Chat Paper
正在生成论文摘要