Content-Invariant Dual Learning for Change Detection in Remote Sensing Images
IEEE TRANSACTIONS ON GEOSCIENCE AND REMOTE SENSING(2022)
摘要
With the introduction of artificial intelligence (AI), current deep learning-driven change detection methods generally regard changes as a type of specific land cover information and try to detect them simply by using existing semantic labeling models. In practice, the changes that occur on different land cover categories may appear completely different for remote sensing images, making it difficult to detect multiple categories of changes with an end-to-end model. In addition, training such networks requires a large amount of prelabeled references, which are labor-intensive and time-consuming. Motivated by this observation, we integrate dual learning algorithm and disentangled representation theory to develop a novel approach, named content-invariant dual learning (CiDL), for either supervised or unsupervised change detection in remote sensing images. In our framework, two opposite Y-shaped networks, each of which consists of two encoders and one decoder, are introduced to translate bitemporal images from their original domains to each others, where their intrinsic content features are retained, while their style features are consistent. By training our hybrid framework, even without references, this method learns a category-wise cross-domain translation to suppress the discrepancies in paired unchanged regions, and meanwhile highlight those in paired changed regions. The experimental results on two typical change detection data sets and the comparison with other state-of-the-art deep learning-driven methods verify the effectiveness and competitiveness of our proposed CiDL.
更多查看译文
关键词
Remote sensing, Geology, Feature extraction, Task analysis, Generative adversarial networks, Training, Redundancy, Change detection, disentangled representation, dual learning, generative adversarial networks (GAN), remote sensing
AI 理解论文
溯源树
样例
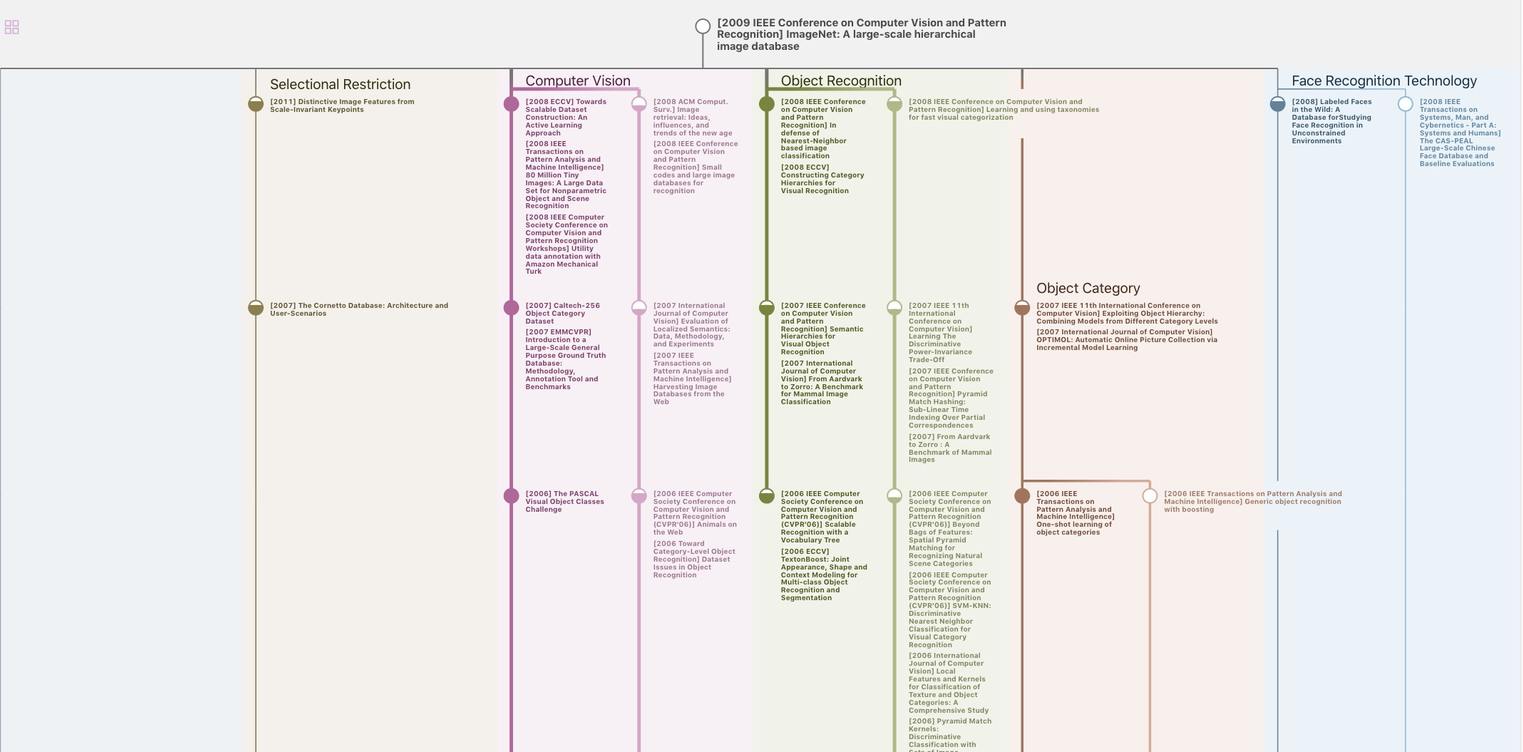
生成溯源树,研究论文发展脉络
Chat Paper
正在生成论文摘要