Improved Smartphone-Based Indoor Localization System Using Lightweight Fingerprinting And Inertial Sensors
IEEE ACCESS(2021)
摘要
The existing radio frequency-based positioning approaches widely used for indoor localization-based service (LBS) are fingerprinting and trilateration and their integration with the inertial sensors-based dead reckoning system. However, these localization methods have practical limits and challenges due to unstable signal strength, the cost of offline workload, computational complexity, terminal device heterogeneity, and accumulated sensor error. We propose a smartphone-based indoor localization system using weighted Spearman's foot-rule (WSF)-based probabilistic fingerprinting for reliable smartphone localization service. This localization system adopts a real-time fingerprinting position error estimation approach realizing an adaptive extended Kalman filter (AEKF) to integrate the proposed fingerprinting localization with inertial measurement unit (IMU)-based localization. This proposed WSF-based smartphone localization uses a Gaussian process regression (GPR)-based signal prediction module to deal with fingerprinting localization's offline workload. Furthermore, the smartphone localization system's expected high computational complexity is controlled by employing a data-clustering module. The proposed WSF also employs a rank vector that helps mitigate the effect of terminal device heterogeneity. The proposed localization system is experimentally evaluated at two different representative indoor environments. Experimental results obtained by real field deployment show that the mean error is 2.06 m in an elongated hallway corridor and 3.47 m in the crowded and well-furnished wide area.
更多查看译文
关键词
Location awareness, IP networks, Radio frequency, Wireless fidelity, Estimation, Computational complexity, Support vector machines, Adaptive EKF, Bluetooth low energy (BLE), data clustering, fingerprinting localization, inertial sensors, spearman’, s foot-rule, received signal strength (RSS)
AI 理解论文
溯源树
样例
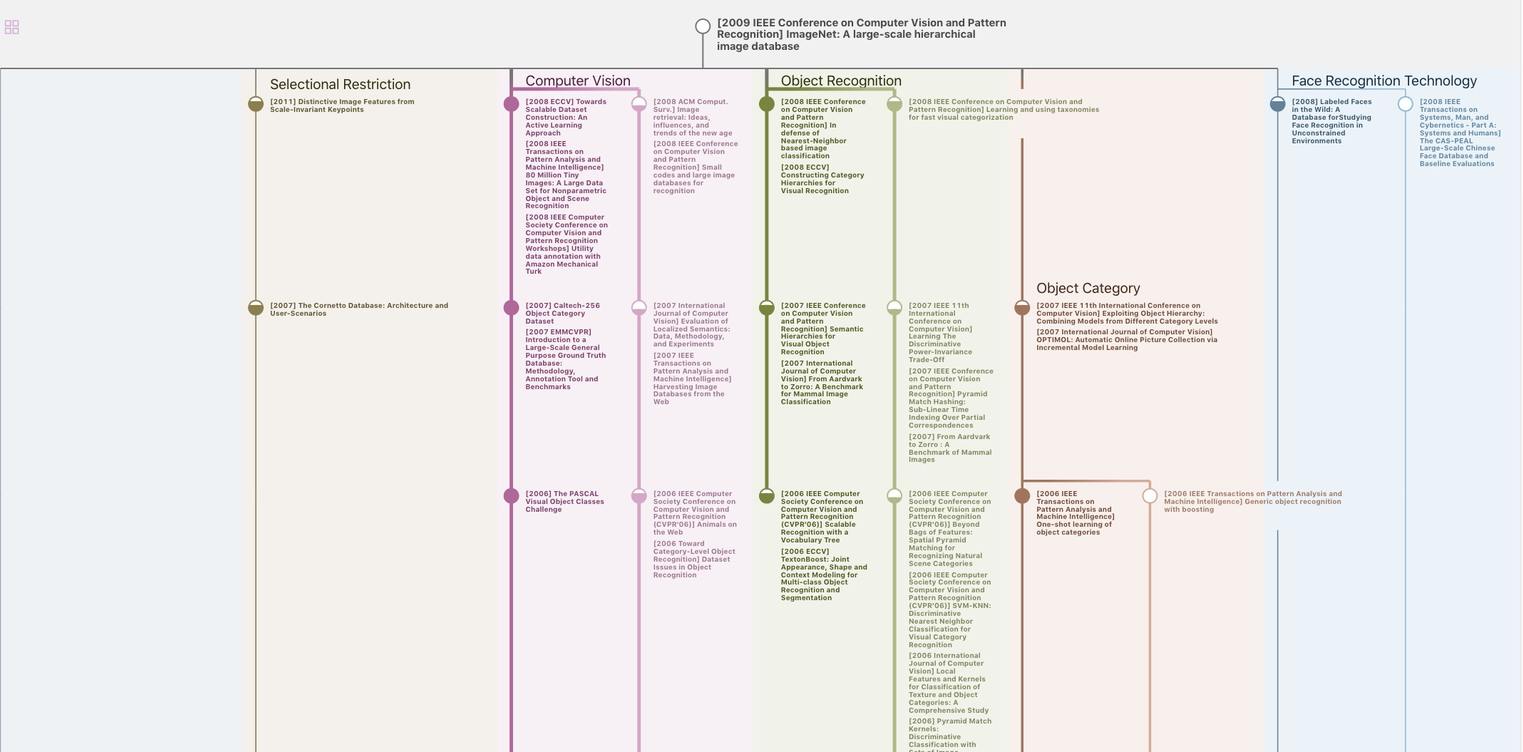
生成溯源树,研究论文发展脉络
Chat Paper
正在生成论文摘要