Artificial Neural Network Based Dose Reduction In Dynamic Brain Pet Imaging
JOURNAL OF NUCLEAR MEDICINE(2019)
摘要
1474 Objectives: Artificial neural network (ANN) based image enhancement has demonstrated its promise in PET imaging dose reduction. The goal of this study is to develop and compare multiple ANN denoising methods with different training data in kinetic parametric image estimation of reduced-dose [11C]UCB-J brain PET data toward optimal performance. Methods To reduce noise in dynamic PET imaging, we work on ANN enhancement of individual activity image frames and parametric images, respectively. In the former scheme, the ANN enhances low-dose dynamic frames before compartmental analysis with the expectation of reduced noise in resulting parametric images. The latter technique processes the low-dose parametric images directly. For activity image frame-processing, we train ANNs to form a patch-to-patch mapping from multiple noise realizations of a reduced-dose dynamic frame to its corresponding full-dose frame. We design two methods in this category. The first one uses 1/10 reduced-dose and full-dose image patches from one healthy control (HC) subject to train the ANN. The trained ANN is then applied once, twice, or three times (cascades) to all 1/10 reduced-dose frames of a different HC subject and an Alzheimer’s disease patient. The second method uses 1/30 reduced-dose and full-dose image patches for training instead. For ANN enhancement of parametric images, the 1/10 reduced-dose and full-dose parametric (distribution volume VT) images from the HC are used for training. In all cases, a fully-connected feedforward ANN model with an input layer, a hidden layer, and an output layer is used to serve as a nonlinear multivariate regression function. The classic stochastic gradient descent (SGD) method is adopted to solve the nonlinear regression problem. We extract 100K 3D image patches from the reduced-dose dynamic frame or parametric image to feed into the ANN as the input. The desired output is the corresponding image patches extracted from the full-dose image. Both the intensity values of the input and output are normalized into [-1, 1] by the min-max scaling method. The normalization ensures the generalization of the ANN trained by one individual frame (or the parametric image) of one subject to all dynamic frames (or parametric images) of others. To evaluate the performance of these methods, we compute the ensemble normalized standard deviation (i.e., noise) among the 1/10 reduced-dose noise realizations and the relative error (RE) with respect to the full-dose image (i.e., bias) for all activity frames and the parametric image across 16 regions of interest (ROIs).\n Results: In activity image frame-processing, the noise reduction of the patient ROIs averaged 42%, 57%, and 66% by applying the 1/10-trained ANN once, twice, and three times to a typical frame, respectively. The ROI-averaged REs of these methods stayed at -1%. Although the 1/30-trained ANN achieved noise reduction of 58%, which was comparable to that from applying the 1/10-dose trained ANN twice, the bias increased to -4%. For the resulting patient VT images, average noise reduction of 39%, 56%, and 65% was achieved by applying the 1/10-trained ANN once, twice, and three times to the dynamic frames, with the averaged REs as 1%, -1%, and -2%. The VT-trained ANN had a noise reduction of 61%, which was between the results from applying the 1/10-frame-trained ANN twice and three times, but the averaged RE increased to 5%. Similar results were acquired in the non-trained HC subject evaluation.\n Conclusions: The cascaded ANN method applying the 1/10-frame-trained ANN three times is effective in noise reduction of the dynamic PET imaging while keeping the bias to a minimum, as compared with other methods studied. The VT images estimated from this method achieved an equivalent 8-fold dose increase compared to that from the original 1/10 reduced-dose [11C]UCB-J brain PET data. More subject evaluation is ongoing while more detailed analysis on the enhanced activity and parametric images will also be performed.
更多查看译文
AI 理解论文
溯源树
样例
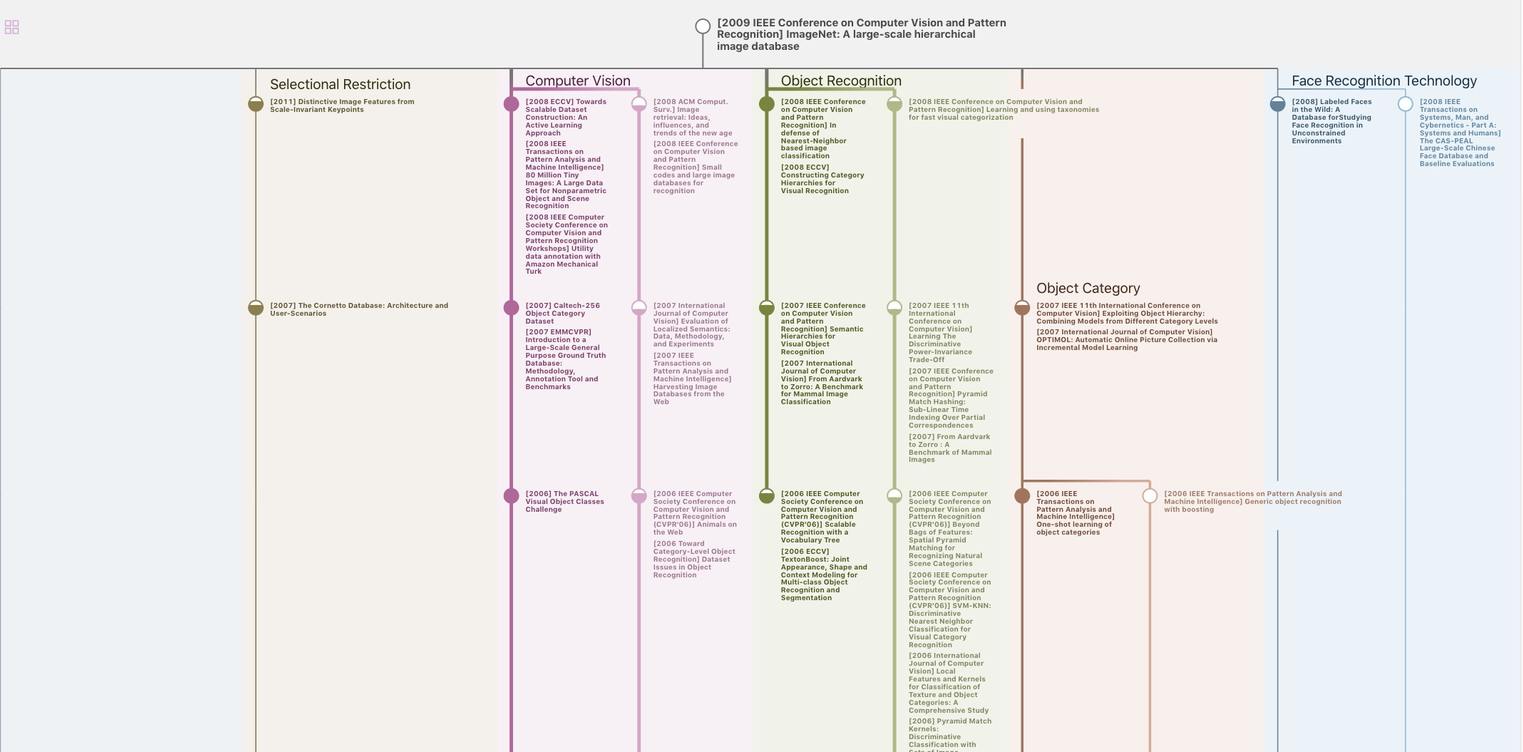
生成溯源树,研究论文发展脉络
Chat Paper
正在生成论文摘要