Machine Learning Classification Of Binary Semiconductor Heterostructures
PHYSICAL REVIEW MATERIALS(2021)
摘要
Heterostructures of two semiconductors are at the heart of semiconductor devices with tremendous technological importance. The prediction and designing of semiconductor heterostructures of a specific type is a difficult materials science problem, posing a challenge to experimental and computational investigations. In this study, we first establish that the prediction of heterostructure type can be made with good accuracy from the knowledge of the band structure of constituent semiconductors. Following this, we apply machine learning, built on features characterizing constituent semiconductors, on a known dataset of binary semiconductor heterostructures extended by a synthetic minority oversampling technique. A significant feature of engineering made it possible to train a classifier model predicting the heterostructure type with an accuracy of 89%. Using the trained model, a large number (872 number) of unknown heterostructure semiconductor types involving elemental and binary semiconductors is theoretically predicted. Interestingly, the developed scheme is found to be extendable to heterojunctions of semiconductor quantum dots.
更多查看译文
AI 理解论文
溯源树
样例
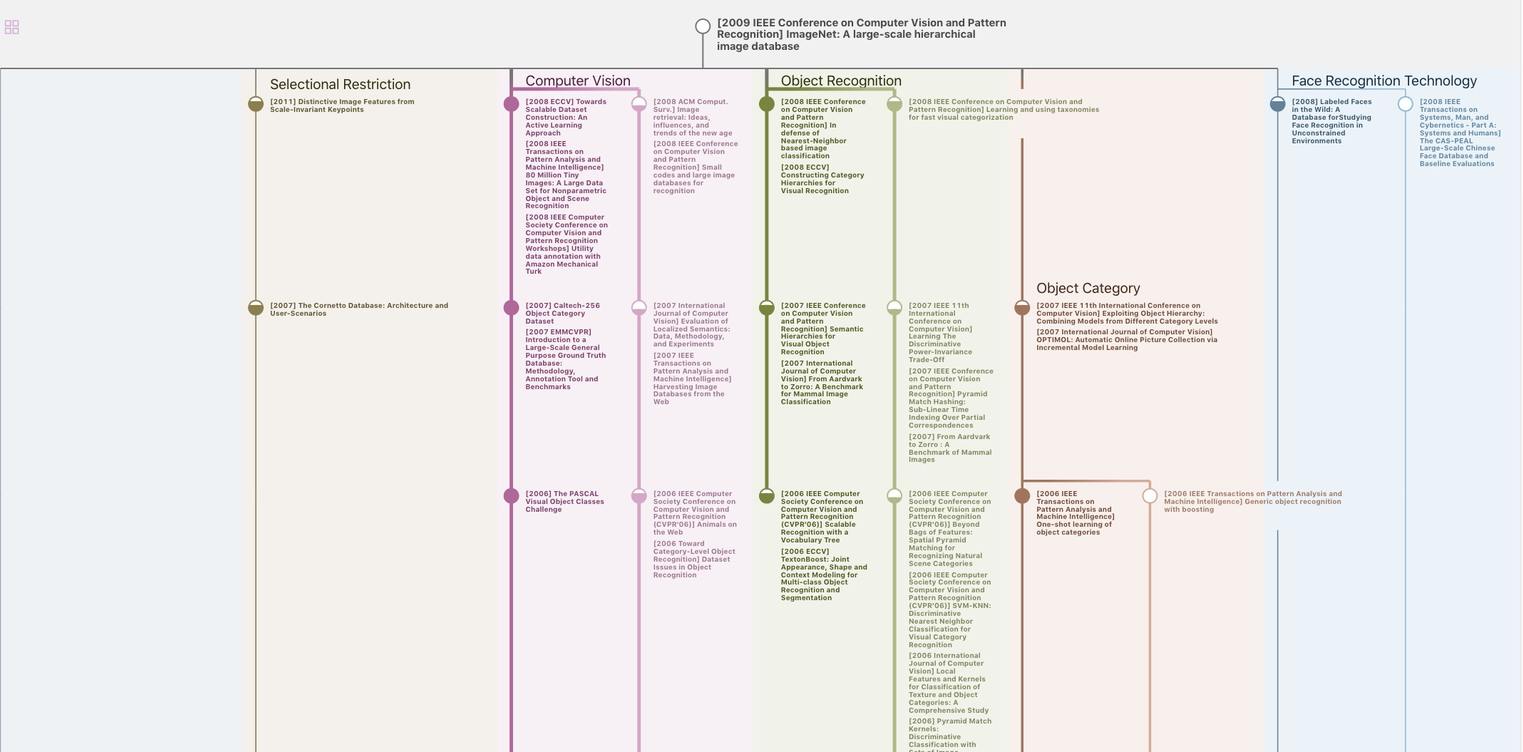
生成溯源树,研究论文发展脉络
Chat Paper
正在生成论文摘要